European Commission VP’s roundtable: influencers in the fight against misinformation / Open Knowledge Foundation
Text originally published at OKFN Greece Blog.
On 15 April, Věra Jourová, European Commission Vice-President for Values and Transparency, visited Greece as part of her Democracy Tour. During this tour, the Vice-President travelled to several European capitals to exchange views with stakeholders on how to better protect the information space in light of the upcoming European elections and the European Commission’s recent democracy package.
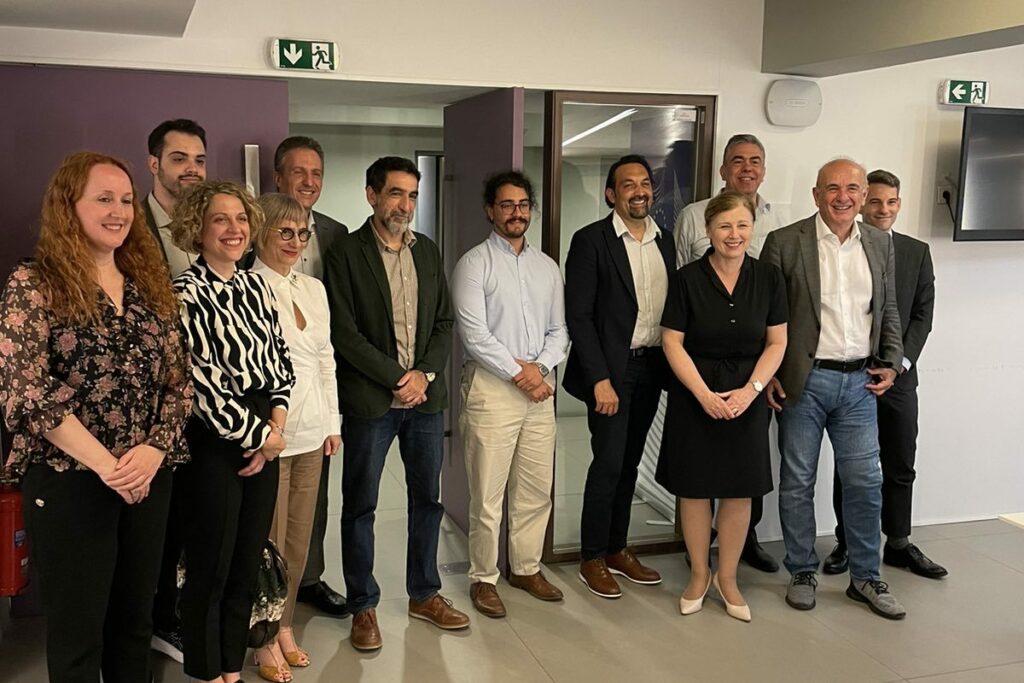
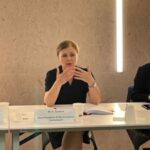
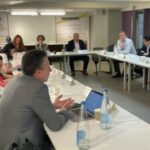
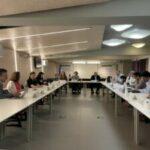
During the Vice President’s visit to Athens, a round table discussion on the fight against misinformation and the protection of the information sphere was organised on Monday 15 April at the offices of the European Commission Representation in Greece, with the participation of Mr. Charalambos Bratisas, Assistant Professor of the Department of Computer Engineering and Electronic Systems of the International University of Greece and President of Open Knowledge Foundation Greece.
The roundtable was attended by representatives of the agencies responsible for implementing the Digital Services Act and the Media Freedom Act to counter foreign propaganda. These were Professor Charalambos Bratsas (Open Knowledge Foundation Greece), Mr Nikos Sarris (MedDMO), Ms Anna Kandylla and Ms Evangelia Psychogopoulou (ELIAMEP), Professor Pantelis Vatikiotis and Professor Betty Tsakarestou (Panteion University), Professor Kostas Mourlas (University of Athens), Dr Yannis Kliafas (Athens Technology Centre), Konstantinos Karantzalos (Secretary for Telecommunications at the Ministry of Digital Governance), and journalists Stelios Pournis (Ellinika Hoaxes), Andronikos Koutroubelis (Fact Review), and Elias Nikolaidis (DiaNEOsis).
At this meeting, Prof Bratsas highlighted the crucial role of open data in the fight against misinformation. “By providing transparent and verifiable data, we can increase the credibility of information and more effectively address the challenges of misinformation,” he said.
Prof Bratsas also stressed the importance of creating tools and platforms that allow for easy and quick verification of the accuracy and authenticity of information. “In this way,” he said, “we can ensure that citizens have access to reliable and objective information while protecting public debate from the influence of misinformation.”
He also underlined the need for cooperation between Open Knowledge Foundation and the European Commission’s Committee on Values and Transparency. He stressed the importance of using open data to promote transparency and accountability and to counter misinformation. Finally, he expressed the desire to work together and exchange ideas on how to harness the potential of open data for the benefit of public trust and democracy.
Open Data Editor: 5 tips for building data products that work for people / Open Knowledge Foundation
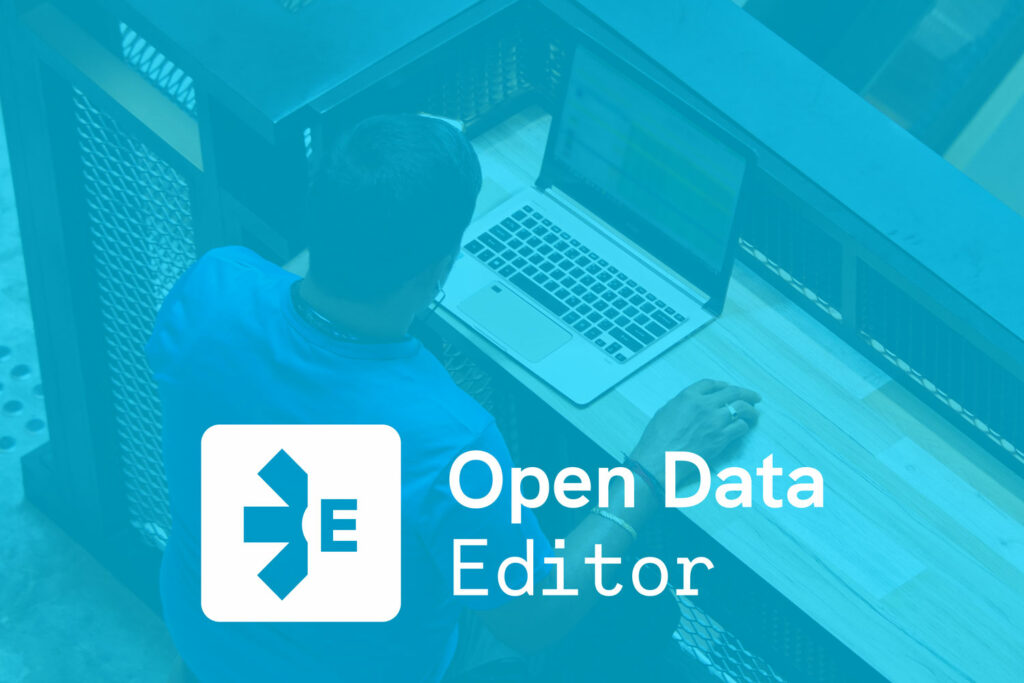
As announced in January, this year the Open Knowledge Foundation (OKFN) team is working to develop a stable version of the Open Data Editor (ODE) application. Thanks to financial support from the Patrick J. McGovern Foundation, we will be able to create a no-code tool for data manipulation and publishing that is accessible to everyone, unlocking the power of data for key groups including scientists, journalists and data activists. (Read more about the Open Data Editor).
[Disclaimer: Open Data Editor is currently available for download and testing in beta. We are working on a stable version. Updates will be announced throughout the year. Learn more here.]
The Open Data Editor is built on top of Frictionless Data specifications and software, and is an example of a simple, open-by-design alternative to the complex software offered by the Big Tech industry. Developing this type of technology is part of our current strategic focus on promoting and supporting the development of open digital public infrastructure that is accessible to all.
As part of this, we want to open up this process in a series of blogs, sharing with the community and those interested in the world of open data how each stage of the creation of this software is developing.
Since the beginning of the year, we’ve been working on building the ODE team and conducting the first phase of user research. We have interviewed 10 people so far, covering different user profiles such as journalists, people working in NGOs and the private sector, and data practitioners in general. Our vision of technology is centred on simplicity and people. We want to encourage real uses of really useful software.
Here’s what we are learning along the way when it comes to user research:
- Practice being a good listener. Being part of the open data community in LatAm, many of the problems people described to me during the interviews were the same ones I face every day. The interviews tested my ability to listen more and talk less
- Paper and pencil. Even if you are recording the interview, make sure you have paper and pencil to write down important things that people might say after you have finished recording.
- Think about your own biases. At OKFN we set a clear timeframe for the user interviews. For the first phase, we decided to start with people we knew from our open data network, based on clear data profiles. Most of them were women (7) and all of them were born in the Global South (Latin America), although some of them work in non-regional positions or the Global North. I disclosed this bias to the team and as the project progresses, I will ensure that I enrich the user research with people from other regions.
- Iterate between documentation, user research and the team you are working with. After your user research, revisit the tool’s documentation. This is always a good exercise. You will discover other key elements that you thought were not there before. Also, when trying to understand the technical elements of the project, ask more than one person in your team to explain to you “how this thing works”. I’m sure you’ll get different answers and they’ll be complementary
- You don’t have to transcribe everything: group the results of interviews by topic to see patterns clearly. Before interviewing people, try to think about the issues you want to address with your questions. Then label the answers so that you can easily see patterns.
If you want to get more closely involved with the development of the Open Data Editor application, you can express your interest in joining one of the testing sessions by filling this form.
You can also email us at info@okfn.org, follow the GitHub repository or join the Frictionless Data community. We meet once a month.
Smile: let's see your teeth / Lorcan Dempsey
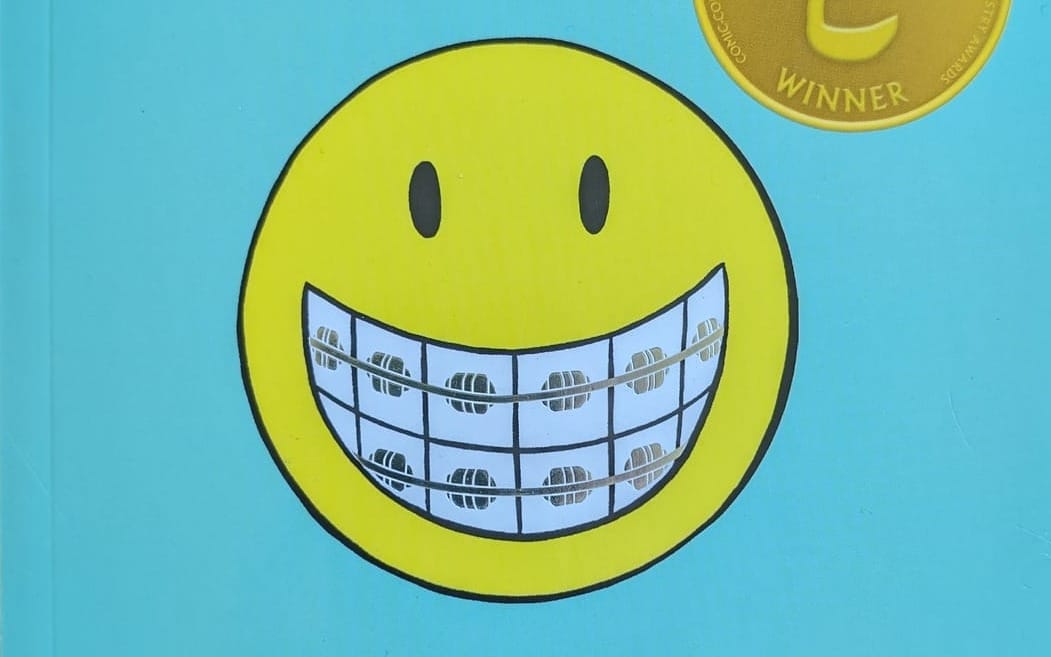
Smile is a very engaging novel. It is the most popular of Raina Telgemeier&aposs works, if measured by Amazon sales. I bought it last year, prompted by the enormously successful exhibition about her work at the Billy Ireland Cartoon Library and Museum at The Ohio State University.
The graphic novel version came out just too late to be a big part of our own children&aposs reading, so I was not familiar with it before.
It is based on the author&aposs own experience of damaging two front teeth in sixth grade, and the emotional, practical and social consequences that followed.
I liked the wit and the clever illustration. I liked how it spoke to the ramifications of such an event through so much of life at an important time in growing up. I have been interested to read a little about its web-comic origins as well as the general impact.
However, most of all, it resonated strongly with my own experiences, and caused me to reflect on those.
I broke a front tooth when I was about 10. We were streaming out of school on the last day of term when the boy in front of me suddenly turned; my upper teeth crashed into his forehead.
I remember the prolonged treatment to try and save the tooth as it became infected. I traveled many times on the bus with my mother to the dental clinic in the city centre, near the looming large and grey Christ Church Cathedral. The clinic was a cold, institutional building.
However, I ended up losing three of my front teeth as the infection spread. I was fitted with a dental plate, which over the years would break from time to time. It also fit progressively less well as I got older, and sometimes in class, I would realise I was moving the teeth in and out with the suction of my tongue on the plate.
I had to take the false teeth out for sports, and it was before people wore mouth guards. In retrospect, I was lucky not to have one of the teeth on either side of the gap knocked out on the rugby field. Swimming was definitely safer. I was not, I confess, a natural athlete, but having to play without front teeth further dampened my desire to participate. In general people were kind, although I was occasionally called Dracula.
Years later, I had what seemed like an overly prominent bridge fitted when I worked for a year in a rubber factory in Germany. When the dentists saw the denture, they were amused and disbelieving that I still wore something so old and by now ill-fitting. Years later again, I had a new less prominent bridge fitted when living and working in the UK. And then when I came to the US, I needed something of an overhaul as the teeth holding the bridge were replaced by implants and I got another bridge.
Yes, with this and other things I have spent a lot of time in the dentist, and a lot of money. Rather more, maybe, than the character in Smile.
I believe the experience shaped me in various ways. It certainly made me self-conscious about smiling, which played into more general social interactions, conspiring with a natural shyness to make me sometimes seem aloof. And I do not like posing for photographs, as smiling on demand does not come naturally.
I was drawn to the way in which psychological and emotional consequences were explored in Smile. I admired the wit with how the story was told and illustrated. However, my normal critical responses were largely suspended as I was transported back into my own at once similar and very different experience.
Picture: Part of the cover of my copy of Smile.
#ODDStories 2024 @ Kathmandu, Nepal 🇳🇵 / Open Knowledge Foundation
Open Tech Community celebrated Open Data Week 2024 with the Open Datathon event. As a pre-event, orientation events were organized through various colleges in Kathmandu with student clubs of the college. The pre-event collective reached approximately 500 students across institutions, the Open Datathon aims to promote open technologies, encourage the use of open data, and share insights about the upcoming Open Data Week 2024.
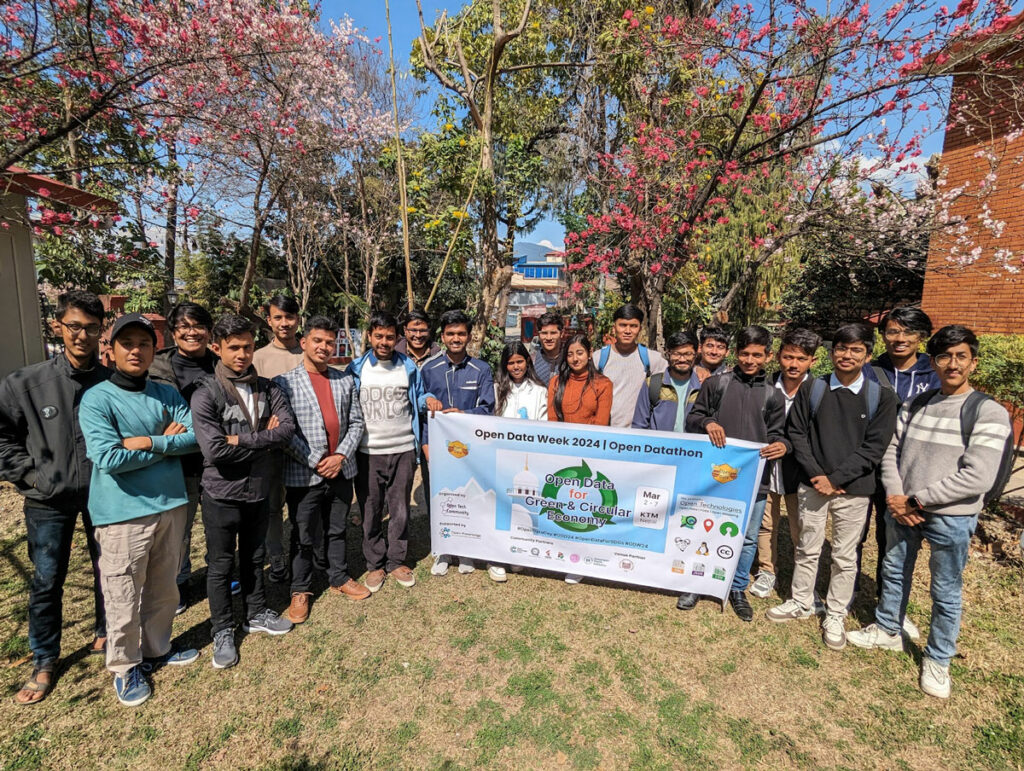
College Collaborators for Open Data Week 2024 (ODW24) pre-events
The goal of the pre-events were to orient the students of colleges to understand the concept of Open Technologies and gain the technical aspect of Open Data which would ease them in participating in the OWD24. Also, these events would bring new and fresh open data enthusiasts to the Open Data Community.
As student clubs were the target of the ODW24, the pre-event was supported by:
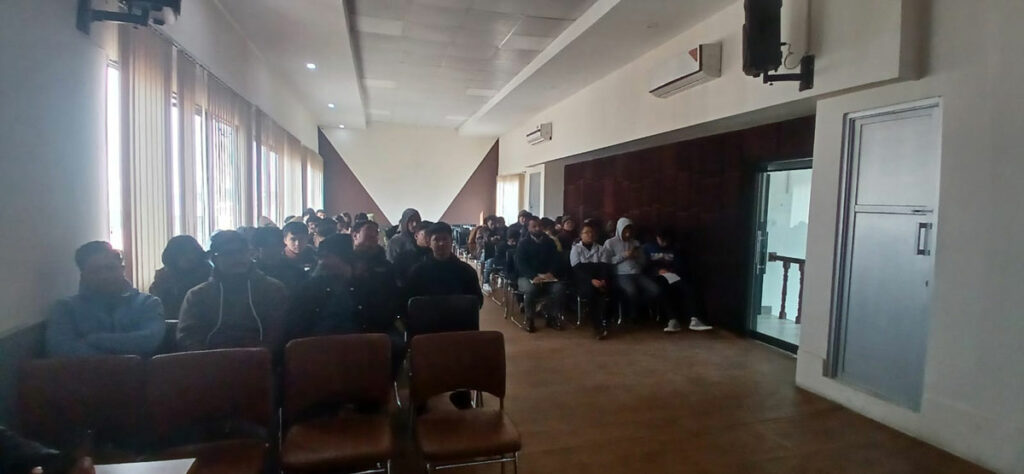
1. Pre-event at Virinchi College
Located at the Lalitpur city of Kathmandu district we did this event on February 25.
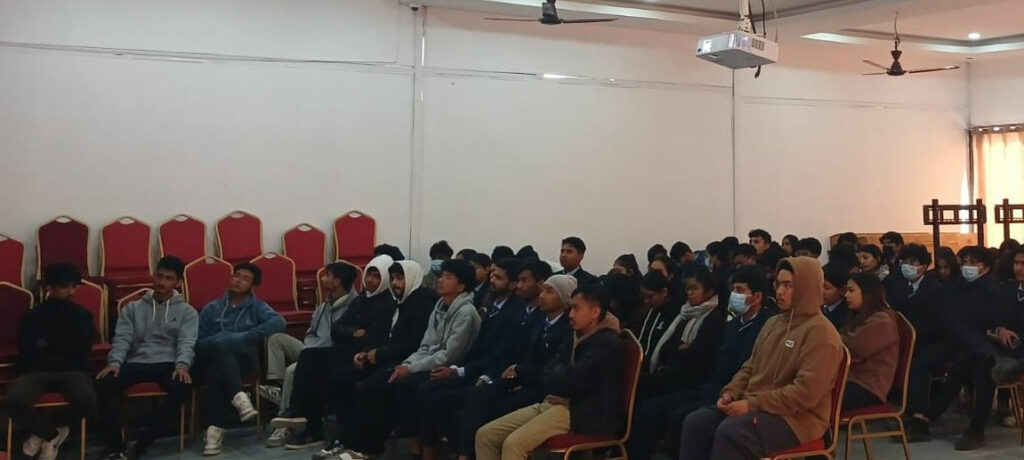
2. Pre-event at Texas International College
Located in Kathmandu city we did this event on February 26.
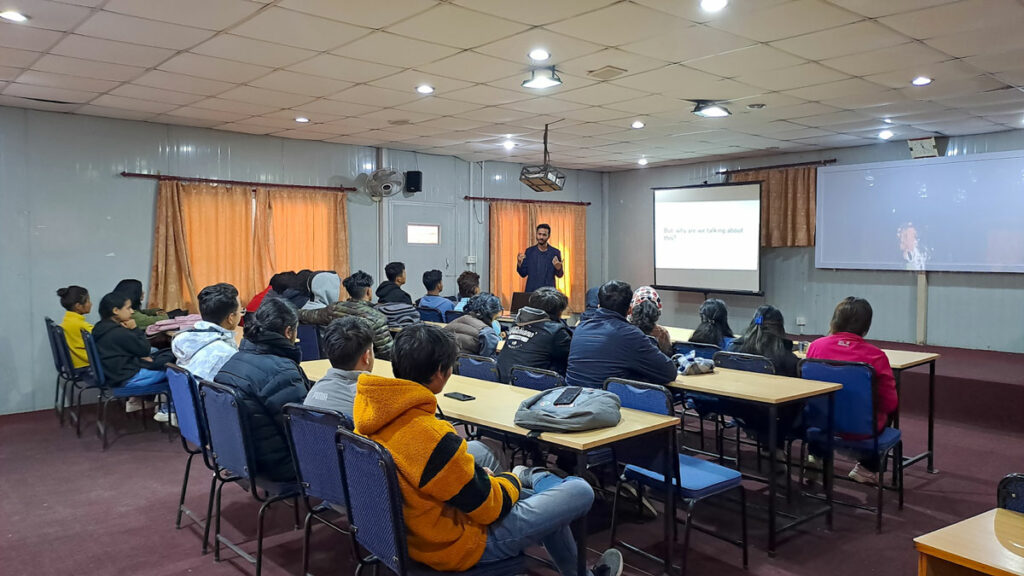
3. Pre-event at Institute of Crisis Management Studies, Samarpan Academy
Located in Kathmandu city we did this event on February 27.
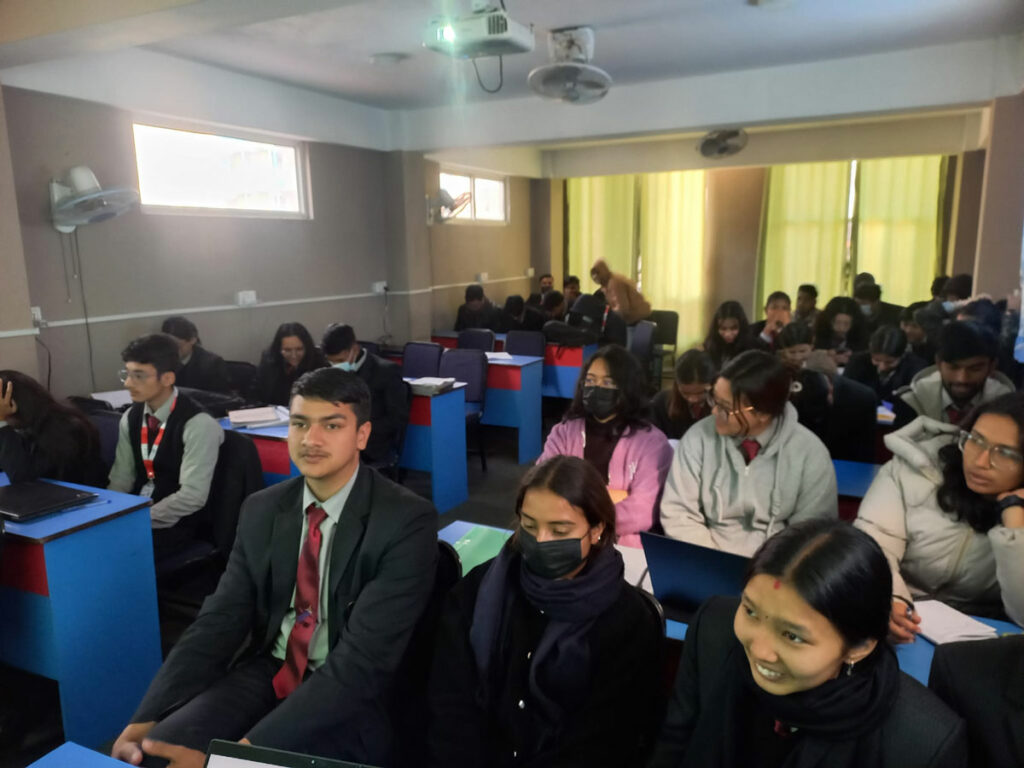
4. Medhavi College
Located in Kathmandu city, we did this event on February 28.
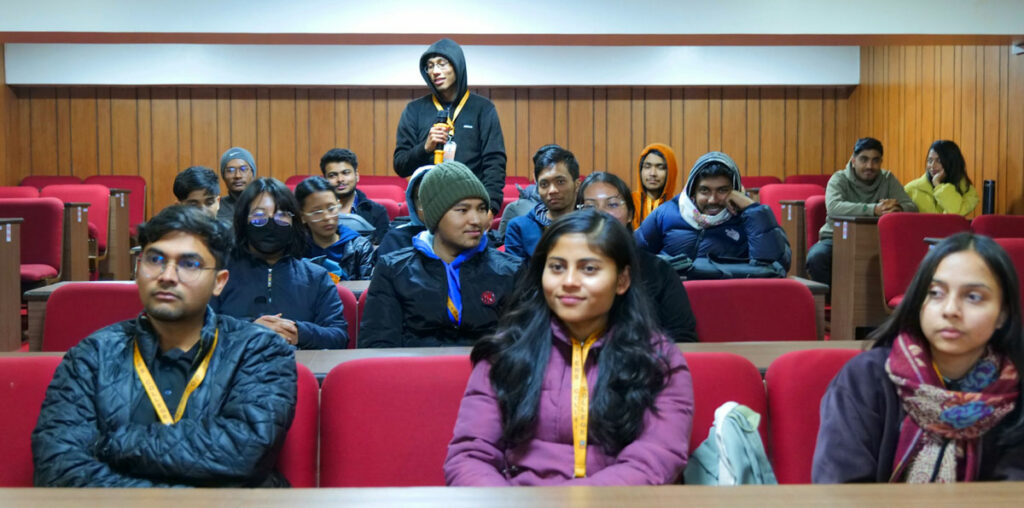
5. St. Xavier’s College
Located in Kathmandu city, we did this event on February 29.
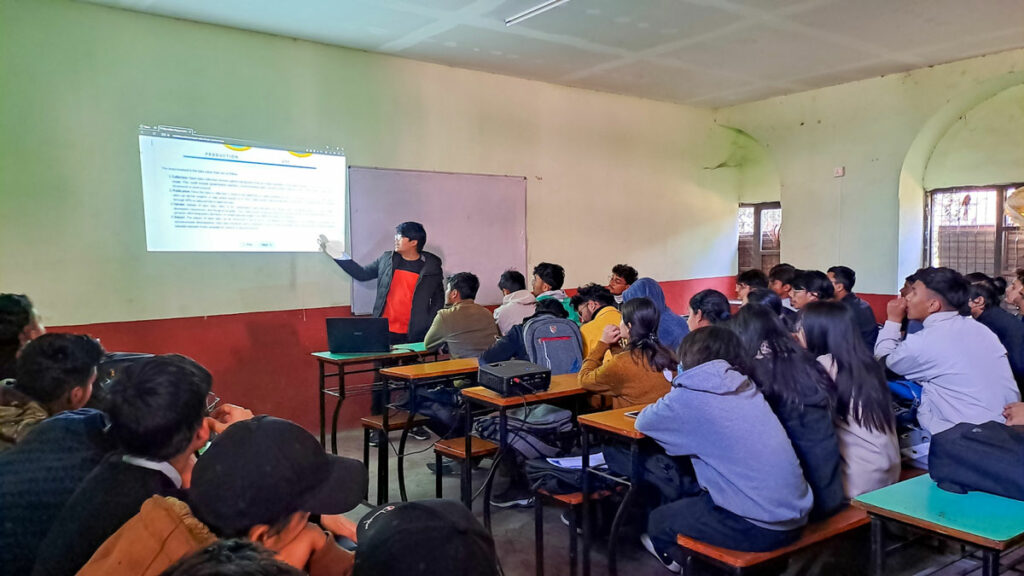
6. Amrit Campus
Located in Kathmandu city, we did this event on February 29.
Each college plays a vital role to create a vibrant environment for students to learn and explore on open technologies and open data.
Social Media Buzz
We used Open Tech Community’s Facebook page and twitter page as our social media outlets of the events and activities:
- Facebook: facebook.com/opentechcommunity
- Twitter: twitter.com/OpenTechNP
- YouTube: youtube.com/@opentechcommunity
Main Event – Open Data Week 2024, Open Datathon
- Event Title: Open Data for Green and Circular Economy
- SDG Focus: Sustainable Cities and Communities
- Date: March 02 – March 07
The main event is set to explore the potential of open data in promoting sustainable practices, with a specific focus on Sustainable Cities and Communities. This aligns with the broader global effort to achieve the United Nations Sustainable Development Goals (SDGs).
We open up an online application form for participants to submit their idea and selected 7 teams from the submitted idea:
- Dumba: Easing in public transportation
- Impact Minds: Finding accommodation easily
- TechBug: Improve local bus transportation
- Team Infinite: Community driven farming
- Nyano Nano: Managing parking spaces
- Team ICMS: City pollution analysis
- Glocal: Recycling city and community waste
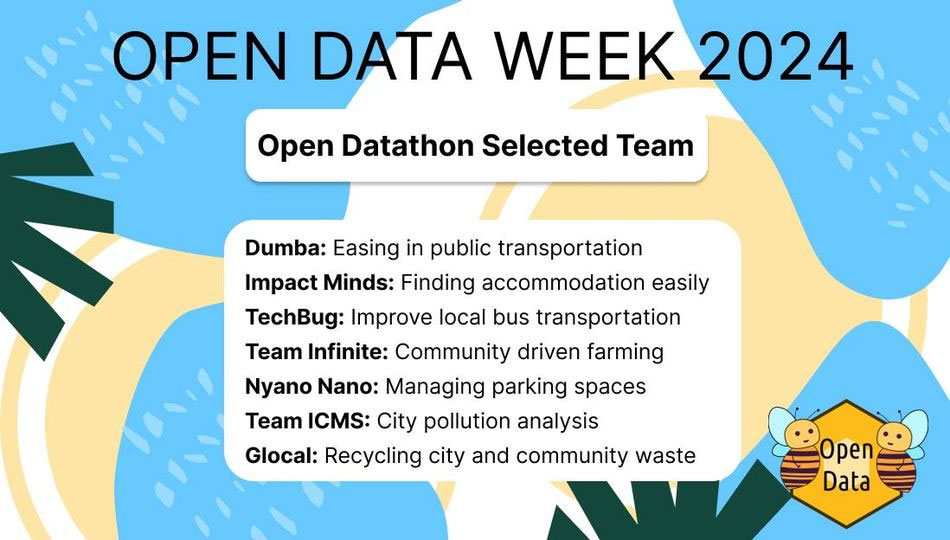
Local Community Partners as Mentors
To support the participants of Datathon to build their idea we take support of esteemed local community partners to mentor participants. These include
- Flutter Kathmandu: Damodar Lohani helped the participants on solving problems related to mobile application development.
- Kathmandu Living Labs: Bhawak Pokhrel helped the participants on solving problems related to building civic technology.
- Open Knowledge Nepal: James Shrestha helped the participants on solving problems related to the concepts of open data.
- YouthMapper Nepal: Nishan Aryal helped the participants on solving problems related to the concepts of data used in open source communities and the concept of green and circular economy.
- Women Leader in Information Technology: Sarila Ngakhusi helped the participants on solving problems related to the concepts of data visualization, web application and business model.
- Open Tech Community: Pawan Kumar Kandel & Arjun Gautam helped the participants on solving problems related to the concepts of building product.
Event Schedule
The event unfolds in a multi-faceted schedule:
- First day at the venue
- Four days of remote mentorship with online sessions
- Final day back at the venue
First day at the venue
On the first day of the datathon event, teams and mentors gathered to share their ideas. Teams talked about their plans, and mentors gave guidance. When teams got closer to mentors, the mentors helped them understand what they needed to do and what they didn’t need to worry about. It was a day of exchanging ideas and getting helpful advice.
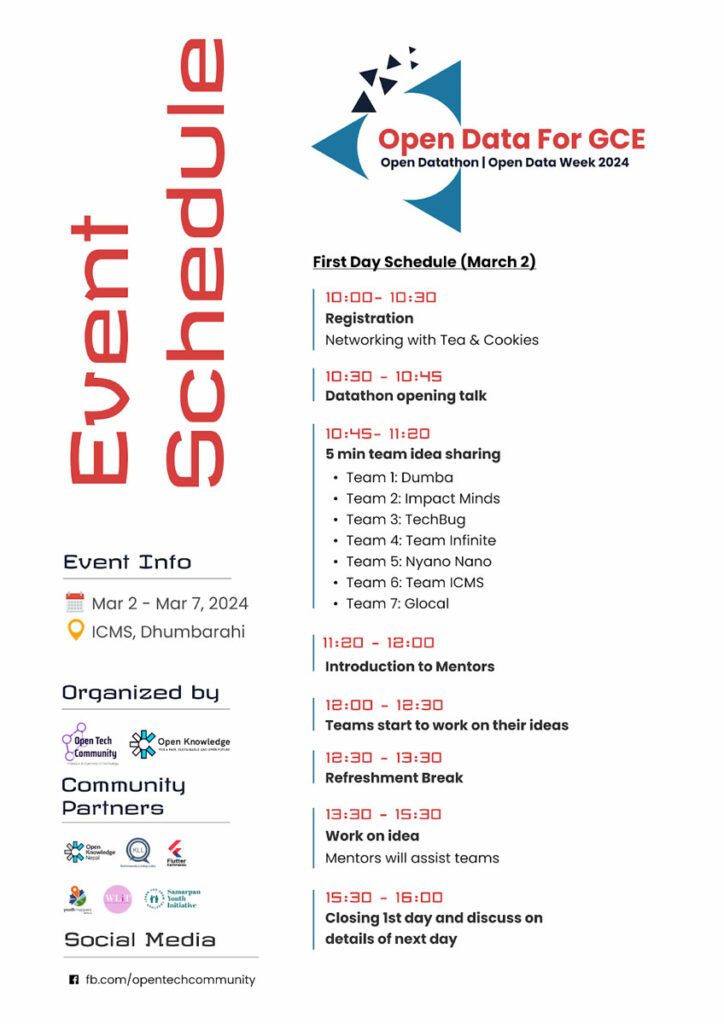
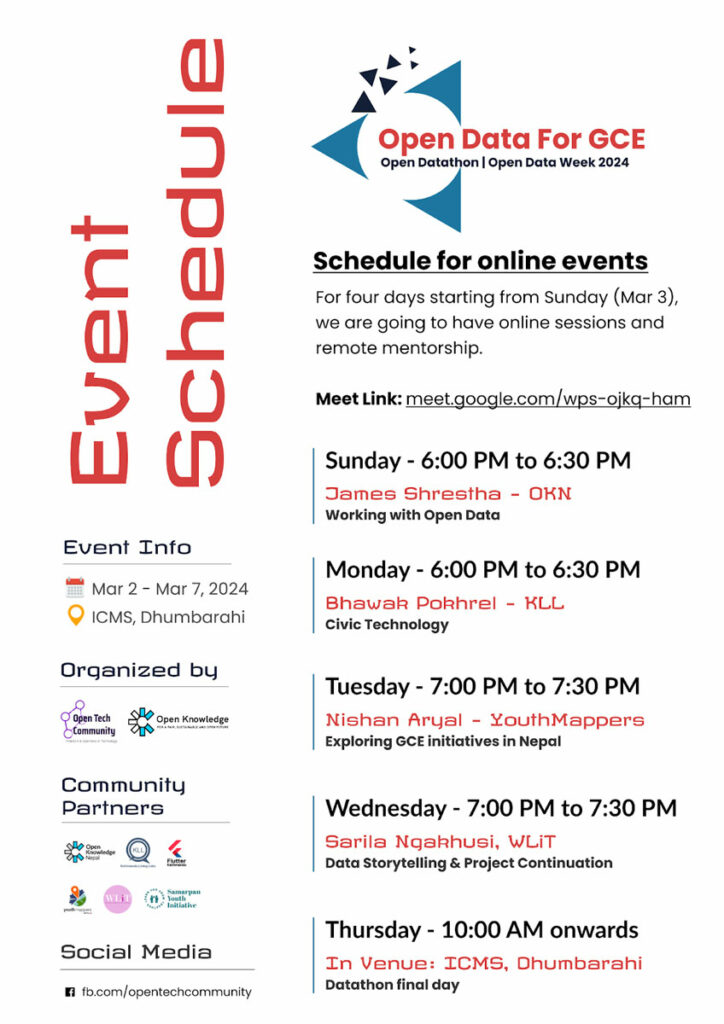
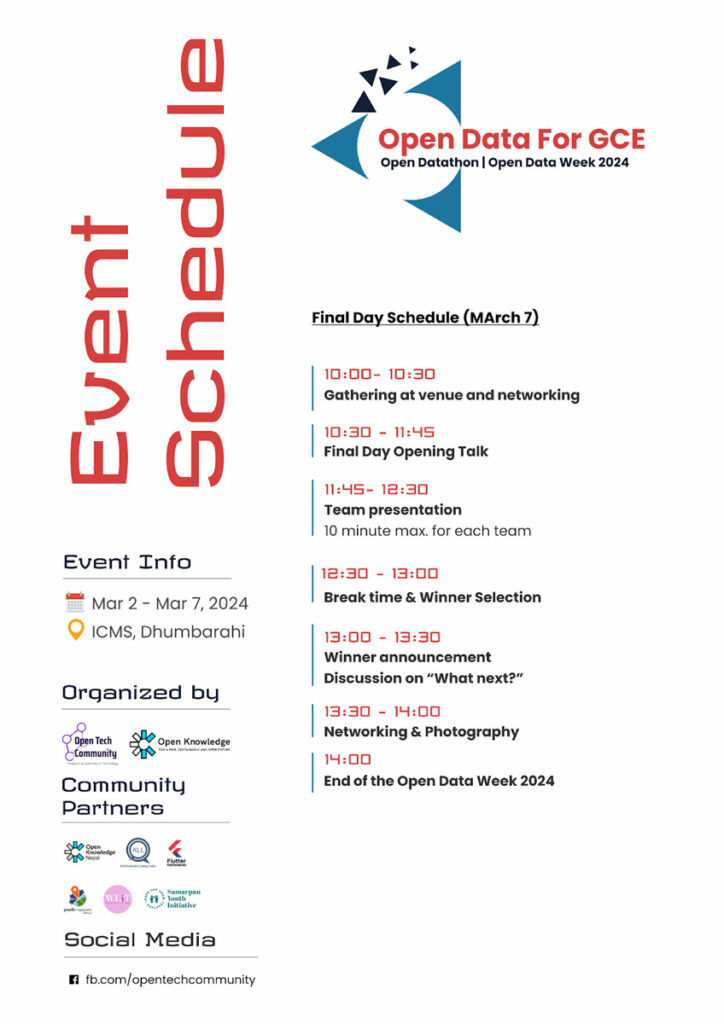
Four days of remote mentorship with online sessions
For the next four days, the event continued online with two sessions each day. In the first session, mentors talked about things that would help teams develop a strong idea. Then, in the second session, participants could ask questions, interact, and share how they were doing with their projects. It was a way for everyone to learn and work together.
Final day back at the venue
On the last day, participants finished up their ideas and plans for a product that uses open data to solve a problem. They then presented these ideas to the judges. The judges for the day were:
- Nishan Aryal, who is an ambassador for YouthMapper in Asia-Pacific
- Bhawak Pokhrel, a senior mobile engineer at Kathmandu Living Labs
- Arjun Gautam, who is a community organizer for Open Tech Community.
This diverse format allows participants to immerse themselves in the collaborative spirit of the Open Datathon, benefiting from both in-person interactions and remote guidance.
Open Datathon Event
The Open Datathon Event was a dynamic and engaging gathering that fostered collaboration and innovation within the realm of data-driven problem-solving on Green and Circular Economy (SDG 11: Sustainable cities and communities). Participants showcase their ideas and expertise in leveraging data to address real-world challenges related to GCE. The event’s interactive nature between mentors and participants created a vibrant atmosphere where participants actively presented their unique perspectives and potential solutions.
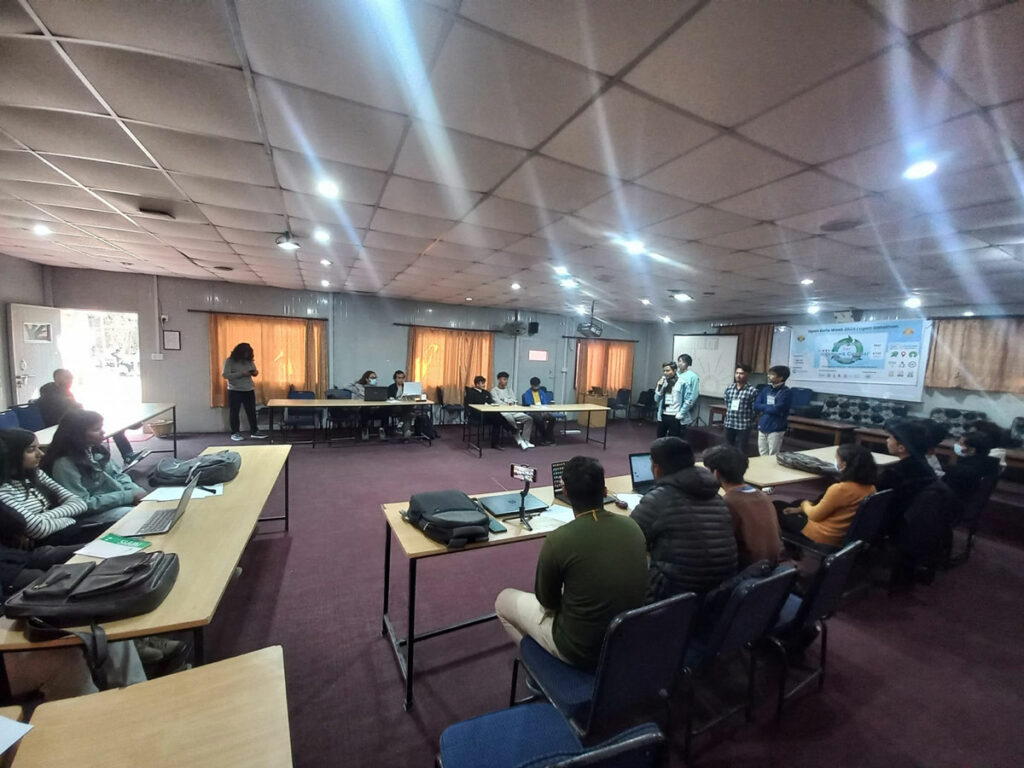
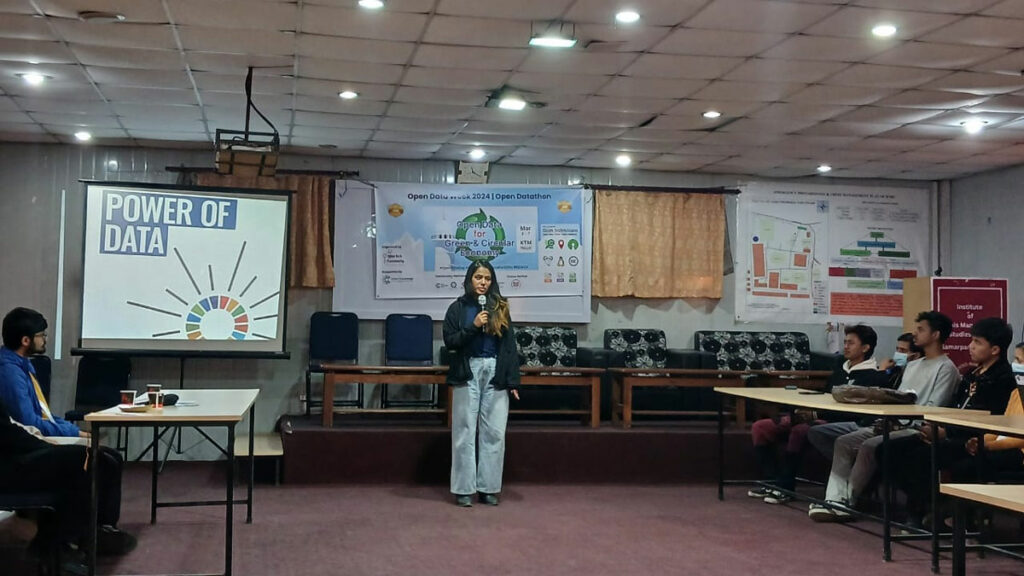
Mascot Release
A symbol of Open Data has always been a search for us in community activities. We released a new Open Data Mascot as a contribution to Open Data communities under Creative Commons license.
The brand-new Open Data Mascot, “DataBuzz Duo” promotes the idea of transparency, collaboration, and the power of data!
As its designer Arjun Gautam says: “DataBuzz Duo” is brought to life by two adorable cartoon bees, presenting the idea of a dynamic spirit of collaboration and teamwork. The background has regular hexagonal shape with text “Open Data”, symbolizing the strength and unity within our Open Data Community.
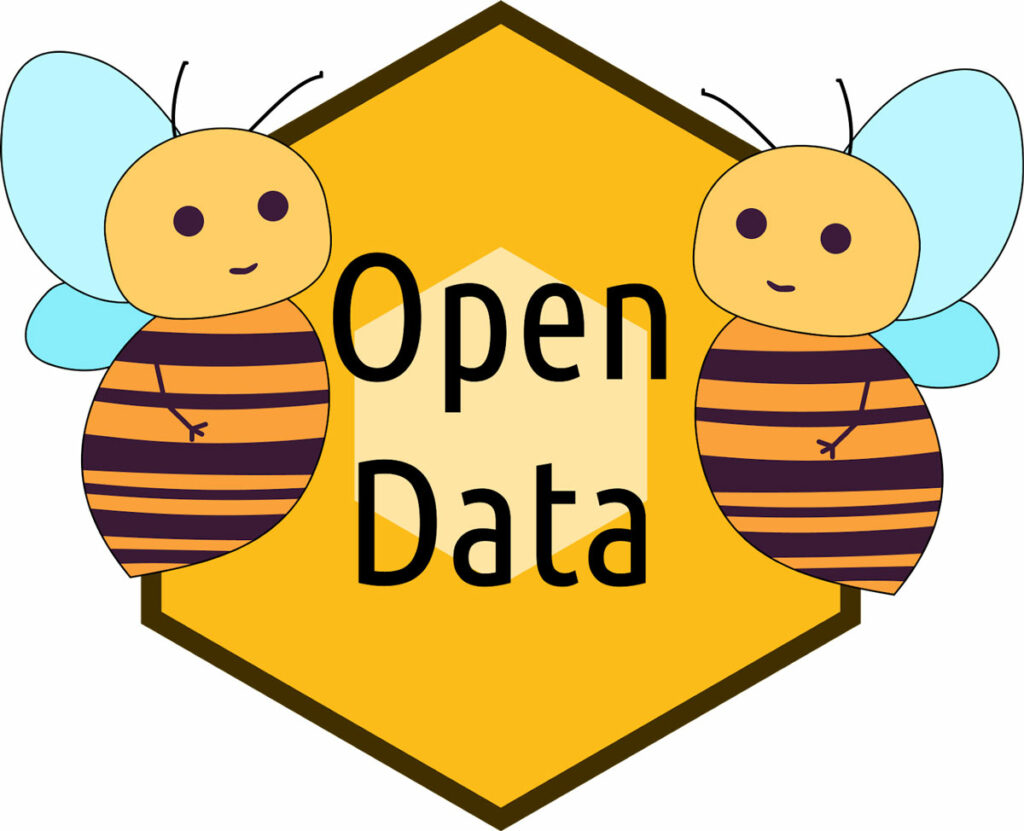
Winning Team at Open Datathon
Out of the 7 teams that took part, the winning team is “Team Dumba” from Amrit Campus. They stood out because of their amazing product idea called “YetaUta.” This idea is all about making transportation in busy city areas smoother and more efficient.
“Team Dumba” showed everyone how their innovation could really make a difference. They came up with creative ways to improve how people move around in crowded cities. Their outstanding effort and unique approach set them apart from the other teams. This recognition highlights their dedication and clever thinking in solving real-world problems during the Datathon event.
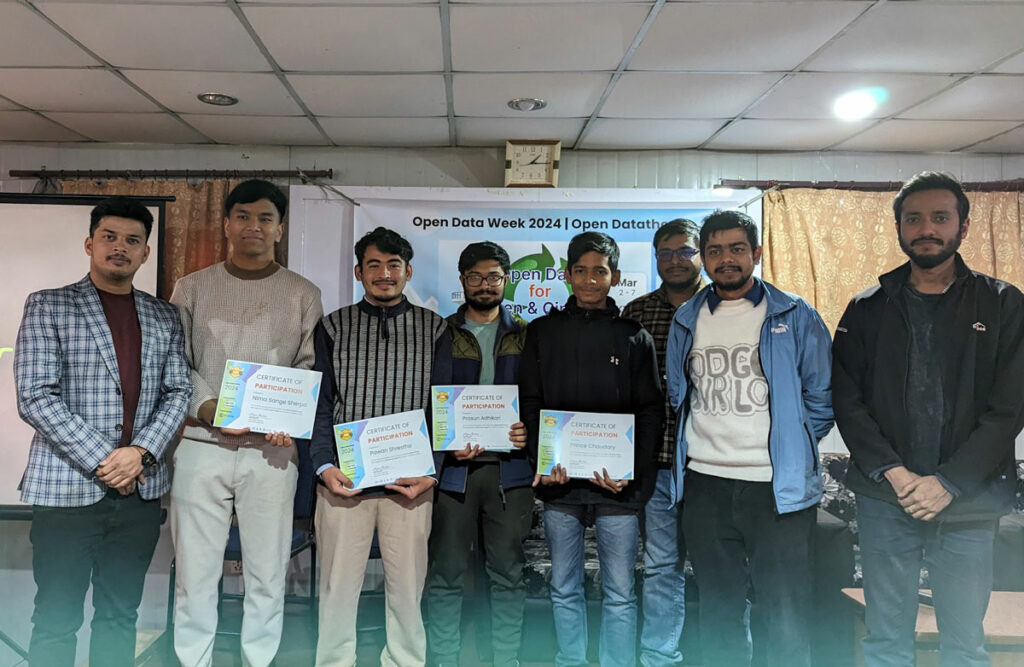
Next Steps for the Winners
For the winning team, “Team Dumba,” the journey doesn’t end with the Datathon victory. We are keen on coordinating their participation in a national-level conference in Kathmandu, offering them a platform to showcase their innovation to a wider audience.
Event Banner, Participants, and Venue Partner
Keep an eye out for the vibrant event banner that encapsulates the essence of the Open Datathon. The diverse pool of participants promises a dynamic exchange of ideas and skills, creating an atmosphere ripe for innovation. A special acknowledgment goes to the venue partner for providing the space and support necessary to make this event a reality.
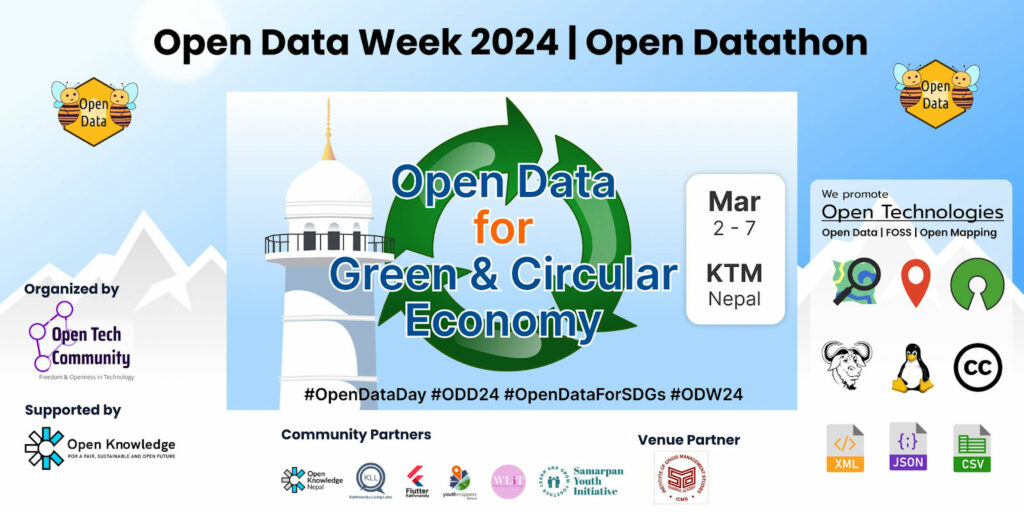
As the Open Datathon gains momentum, it’s evident that this gathering of minds is not just an event; it’s a step towards a more sustainable and connected future.
Outcome
The Open Datathon event energized our open data community, fostering collaboration and innovation. It engaged 7 diverse teams, promoting sustainable solutions for a greener future. Through mentorship and idea-sharing, participants developed impactful projects, supporting the power of open data in solving real-world challenges. This vibrant gathering not only celebrated creativity but also laid the groundwork for a connected future. A heartfelt thanks to the Open Knowledge Foundation for this opportunity, ensuring that our community remains dynamic and ready to tackle tomorrow’s challenges with the spirit of openness and collaboration.
About Open Data Day
Open Data Day (ODD) is an annual celebration of open data all over the world. Groups from many countries create local events on the day where they will use open data in their communities.
As a way to increase the representation of different cultures, since 2023 we offer the opportunity for organisations to host an Open Data Day event on the best date within a one-week period. In 2024, a total of 287 events happened all over the world between March 2nd-8th, in 60+ countries using 15 different languages.
All outputs are open for everyone to use and re-use.
In 2024, Open Data Day was also a part of the HOT OpenSummit ’23-24 initiative, a creative programme of global event collaborations that leverages experience, passion and connection to drive strong networks and collective action across the humanitarian open mapping movement
For more information, you can reach out to the Open Knowledge Foundation team by emailing opendataday@okfn.org. You can also join the Open Data Day Google Group to ask for advice or share tips and get connected with others.
Decistifying trans and gender diverse inclusion in library work: A literature review / In the Library, With the Lead Pipe
In Brief
This comprehensive review illuminates the current state of scholarly literature on trans and gender diverse inclusion in libraries, with the intention to provide a foundation and identify gaps for further research. Covering 50 works published between 2002 and 2023 in the areas of general inclusion, public libraries, academic libraries, experiences of library workers, archives, information behavior, and cataloging, we found that, with the exception of works on archives and cataloging, little scholarly literature goes beyond introductory talking points on basic information about trans and gender diverse people. We conclude with a call for much more in-depth research on this essential topic.
by Keahi Adolpho and Stephen G. Krueger
Trans and gender diverse people1 are present in all areas of library work. We use libraries of all types, as members of the public, as students, as researchers. Though as of this writing the most recent public demographics from the American Library Association do not count us (Rosa & Henke, 2017), we are library workers and volunteers. We are library students and professors. Just as importantly, we are people who are not any of those things, often due to exclusionary practices on the part of library systems, spaces, or individual employees. The harm may not always be intentional; many library workers mean well and simply have not educated themselves on how to eliminate trans-exclusionary practices and institute gender inclusive ones.2 This literature review is one tool for supporting that work.
Scope and Purpose
Through our engagement with gender inclusion work since we joined the library profession, we have observed that the majority of the literature consists of trans 101 talking points (e.g. definitions of gender terminology, explanations about the difference between gender identity and sexual or romantic orientation, basic information about pronouns, statistics about violence and other harm enacted upon trans and gender diverse people). While this kind of remedial content is necessary, it merely asks individuals to reduce or avoid harm, rather than imagine possibilities for truly gender inclusive library systems, practices, and spaces.
This article is an overview of the current state of scholarly literature on gender inclusion in libraries, in order to provide a foundation and identify gaps for further research. The material covered includes journal articles, proceedings from library conferences, book chapters, and full books. It is worth acknowledging that our scope leans more toward the types of literature that academic librarians are more likely to both publish and engage with, rather than public or school librarians. Many—though still not enough—blog posts, magazine articles, webinars, and other materials address the topic, so we do not present this article as an encapsulation of everything library workers have created about gender inclusion.
In order to focus on our topic, as well as to make the project feasible, we placed a number of other restrictions on what is included.
- Library-centric: There are some works that address the central theme from external perspectives, such as articles about trans and gender diverse information behavior, without being tied back to the library context. Materials of this type were not included, as the intent was to focus on how library workers and scholars are addressing trans and gender diverse topics in the context of library work.
- Trans and gender diverse-centric: There is a lot of library literature on LGBTQ+3 identities, including but not specific to trans and gender diverse people. While these materials may have some content relevant to the topic and are valuable in their own right, they were not included. The needs and experiences of trans and gender diverse people are not synonymous with those of others under the LGBTQ+ umbrella, though there may be some overlap, and the purpose of this project was to look at materials specifically about trans and gender diverse people in regards to library work. In addition, a great deal of work claiming to cover LGBTQ+ people omits trans and gender diverse people entirely. Pieces about gender that did not center trans and gender diverse people were likewise excluded.
Methodology
We have both been working on gender inclusion in libraries for several years, so we were familiar with a lot of the existing literature already. Long before deciding to write this article, Stephen created a Zotero group for anything on the topic that anyone can add items to. Many of the materials came from citation chasing. We performed a final search of LISTA (Library, Information Science & Technology Abstracts) on December 1, 2023, using the search terms (transgender OR transexual OR transsexual OR gender diverse OR nonbinary OR non-binary) AND librar* to make sure we had everything we could find before plunging into analysis. An overall analytical methodology would have been difficult to apply given the variety of types, lengths, and focuses of the materials. For each piece we considered its context and objectives and assessed it accordingly. For example, a brief introductory article would not be expected to go into as much depth as a chapter researching a specific topic. Reviews, newspapers, and works in languages other than English were filtered out. The resulting list had 324 items, most of which fell outside the scope outlined above; we looked through these to find ones that did not. With these search techniques and limitations, we ended up with a total of 50 works, dated from 2002 to 2023.
Literature Review
This review begins with general literature that addresses gender inclusion in libraries broadly. Then, we look at the literature in public libraries and academic libraries. Topically, these two sections cover representation, youth services, recommendations, misinformation, collection development, and task forces. The fourth section reviews literature by and about trans and gender diverse library workers on their experiences in the profession. The fifth section discusses archives, looking specifically at access barriers, description issues, and collections. The next section is on information behavior which includes studies on trans and gender diverse people in order to better understand how libraries can serve these communities. The last section is the most well developed area of gender inclusion work in libraries; it addresses cataloging and metadata, looking specifically at the history of trans and gender diverse subject access, the development of best practices, and Resource Description and Access (RDA) rule 9.7, which instructed catalogers to record gender information in name authority records.
General
A few pieces address gender inclusion in libraries broadly. An essay by Marquez (2014) provided basic definitions, followed by an overview of the current (at the time) literature on trans and gender diverse issues in libraries, discussion of restrooms, and a brief section on other elements of gender inclusion. A conference paper by Pun et al. (2017) profiled all gender restroom situations in different types of libraries, including contextual factors like local and state politics and institution type; the authors recommended that libraries collaborate with community and institutional partners such as gender studies programs or diversity centers.
In an article directed towards those in leadership positions, Keralis (2023) asked “how do libraries become gendered places, what harm might that cause – however unintentionally – to transgender and gender non-conforming patrons and staff, and how can library leadership ameliorate this harm if they choose to do so?” Keralis defines “gendered place” as a space that perpetuates the gender binary, further explaining that binary to functionally be male and not-male (p. 284). Suggested actions included growing and learning, modeling inclusive behavior, consistently enforcing policies, and—most importantly—actively and publicly leading.
So far, the only full book on gender inclusive practices in library work is Supporting Trans People in Libraries. The first few chapters covered trans 101 and pronouns; the next several offered practical guidance for the most common issues that come up in libraries, including how to gather personal information, hiring practices, conferences/events, and, of course, restrooms. Interactions with patrons and with employees get a chapter each, as do library schools, academic libraries, access services, and collection development. Concluding material includes a glossary and recommended resources (Krueger, 2019).
Public Libraries
The two pieces on youth services in public libraries mainly address representation, information behavior, and how public librarians can better serve trans and gender diverse youth. Sokoll (2013) discussed the importance of representation in young adult literature for trans youth and the inadequacies of traditional collection development tools in locating relevant materials. The article included recommendations of specific titles to purchase and supplementary websites to consult for collection development purposes. Austin’s (2019) chapter attempts to cover a lot of ground, which is perhaps an understandable desire given how little in library literature moves beyond basic information about gender identity. From trans 101 to broad issues (representation in mass media, legal recognition and medical care for trans and gender diverse youth, surveillance) to library-adjacent topics (information behavior of trans and gender diverse youth, how youth turn to zines and online spaces to share information and find community), the scope is extremely broad. The chapter included a number of suggestions that would significantly widen the scope of work of public librarians, which includes that librarians could perform outreach to trans-friendly medical providers to co-create resources, maintain lists of trans-friendly medical providers, and create resources on processes for youth seeking transition-related care (p. 177). Other suggestions fell within regular library job duties, such as providing critical information literacy support around misinformation and fake news, curating library guides of online resources and booklists, and creating racially diverse displays that link printed images to online projects such as the Digital Transgender Archive. Recommended general practices included hiring and respecting trans and gender diverse staff, and not expecting trans and gender diverse people to serve as information sources.
Byrne (2020) described the need for public library management to actively support gender inclusive practices in addition to expressing good intentions; after describing a committee’s attempt to enact meaningful change in their library, the author concluded that they must rely on themself rather than hoping for movement from managers and administrators.
The chapter by Sancho-Brú, McIntyre, and Bermúdez Raventós (2019) discussed the Trans Identities and Gender project to build a collection of materials about and for the trans and gender diverse community in Catalonia, which (at the time, at least, and possibly still) “is the only collection centered on trans identity that exists in the public library network in Catalonia” (p. 252). The project, which was started by a trans activist who began their transition while working in libraries, included a photographic exhibition, a living library, and “ongoing activities using the library as a communal meeting point between the local and the trans community as well as a means of promoting the collection and its use” (p. 244). The opening event had the highest public attendance of any event held by the library in its 20 year history.
Academic Libraries
In a conference paper on trans inclusion in libraries presented at an academic library conference, Smith-Borne (2017) provided nine recommendations. The only portions specific to the academic library context are recommendations to learn about and connect with campus resources such as LGBTQ+ centers. The other items vary between applicable to most libraries, such as “Remove gendered language on signage, library forms, web forms, or surveys” (p. 112), and the most basic elements of trans inclusion, such as not assuming gender. A few years later, Smith-Borne (2019) wrote about creating an inclusive environment for trans and gender diverse music library users; with a few exceptions (such as creating an exhibit featuring trans composers and encouraging music faculty to refer to choir parts by name rather than assumed gender), this guidance too was essentially about gender inclusion in libraries overall. Both pieces covered trans 101 level information. Krueger and Matteson (2017) suggested relationship building with campus and local LGBTQ+ centers, workshops and training to build a foundation of knowledge, as well as the importance of moving beyond education into practical support. This would include removing gendered language from written and oral communication, not guessing the pronouns or gender of patrons, all-gender restrooms, anonymous online chat, and self-checkout options.
Two articles discussed how anti-trans bias relates to misinformation, and what academic libraries can do to combat this. Lockmiller (2023) discussed the harm of anti-trans misinformation through a theory called the “Misinformation – Legislation Pipeline,” using Florida as a case study to show how stigmatization and misinformation can lead into legislation around healthcare (pp. 742-753). In conclusion, Lockmiller discussed the role of health science librarians in recognizing these pipelines, ensuring that collections do not feed into them, redirecting researchers away from misinformation, challenging the need for assignments and research to always address “both sides” of a debate, and creating curricula around understanding these pipelines (pp. 757-758). Krutkowski et al. (2019) discussed the global, UK legal and social, and institutional contexts around anti-trans discrimination and fake news before describing the aims, content, and overwhelmingly positive reception of a professional development session on these topics (pp. 115-118). They concluded by encouraging information literacy interventions against misinformation and bias towards marginalized groups (p. 124).
Occasionally, an academic library develops a working group or task force on gender inclusion. Marrall (2015) described one such effort at Western Washington University to develop best practices for serving trans and gender diverse patrons (p. 184), following a university resolution to implement gender neutral restrooms across campus and a preferred names policy. Marrall concluded that whether other libraries should implement best practices for gender inclusion depends on if there is administrative support, prior DEI work on this topic, patron need, collegial support, existing expertise, and more. Marrall reminded readers that committing to this work requires ongoing learning and labor (pp. 187-189). Doherty and Coghill (2020) described the Virginia Commonwealth University Libraries’ Gender-Inclusive Work Group, which started out by seeking direct feedback from student workers as well as conducting a literature review and environmental scan. The libraries had already implemented some practices including optional Safe Zone training, removal of gendered pronouns from job postings, and a few all gender restrooms. The group recommended immediate actions, such as wastebaskets in all restrooms, and long-term ones, such as hiring a diversity and inclusion librarian.
Leahy (2023) described the process of selecting a 20-item collection of “transgender life-writing” for an academic library. Noting that “the project recognizes that the category of ‘life writing, autobiography, or memoir’ does not reflect all transgender experiences,” Leahy explained that items beyond this official scope were included in order to increase the racial diversity of the materials and represent a greater variety of trans experiences (p. 96). Difficulties included the often outdated and offensive cataloging terms the materials were tagged with, and the division of what had been envisioned as a cohesive collection into different areas of the library (some closed-access).
Wagner and Crowley (2020) used critical discourse analysis to examine library guides, article abstracts and author biographies of work produced by trans and gender diverse scholars, and shelving at academic libraries as examples of “continued systematic failures” that “alienate, ignore and exploit” trans and gender diverse people (p. 160). Their analysis of library guides revealed an overreliance on academic sources that largely focused on white gay men, and that only 50% of the guides addressed or mentioned trans and gender diverse students. Their analysis of article abstracts and author biographies found only one author referred to with the correct name and pronouns, six authors misgendered, and one author both deadnamed and misgendered. In their review of shelving at academic libraries, they found trans and gender diverse books near books about intersex studies, sex work, kinks, and fetishes. They argued that, while none of these topics should be viewed negatively, the proximity of the materials may lead to incorrect connections and conflations (pp. 168-175). Wagner and Crowley conclude by stating “valuing justice and equity will always be different from doing justice and equity” and by encouraging libraries to “also look inward to think about how they might never have been inclusive” (p. 178).
Library Workers
The first book in the Series on Gender and Sexuality in Information Studies is Out Behind the Desk: Workplace Issues for LGBTQ Librarians (Nectoux, 2011). Four of its nearly 30 chapters make up the section “The Rest of the Rainbow,” which seems to mean everything beyond lesbian and gay. Only one, “Passing Tips and Pronoun Police: A Guide to Transitioning at Your Local Library,” fully centers trans identities, though others touch on them. The largely personal narrative includes the experience of coming out (and being outed, though not maliciously) during a job search, and of transitioning at work: “My coworkers didn’t have much experience with a trans colleague, but I didn’t have much experience being a trans colleague, so ultimately we were well matched” (Roberto, 2011, p. 125). The chapter concludes with a section speaking directly to trans and gender diverse library workers, which is refreshing given how rarely LIS literature acknowledges our presence as anything other than patrons.
In contrast is the most recent (as of this writing) book in the same series, Trans and Gender Diverse Voices in Libraries (2023), whose three editors and almost 60 chapter authors are all trans or gender diverse people (Adolpho et al.). (Full disclosure: two of those editors are the authors of this article, so we may be a little biased about the book.) Consisting primarily of personal essays, with some professional thoughts and even a few poems, it showcases a range of experiences and identities of trans and gender diverse LIS students and library workers. The first section, Personal Experiences, consists of chapters that center the author’s identity more than a particular area of library work. LIS Education covers the experiences of trans and gender diverse LIS graduate students. It is worth noting that half of the 10 authors in this section opted for anonymity; the library field as a whole would do well to reflect on what it means that incoming members question whether they can share such experiences under their professional names, enough so to pass on the benefits of having a published chapter on their CVs. The sections Public Libraries, Academic Libraries, and Archives and Special Collections all, as one might expect, include chapters by people in these specialties. Professional Reflections consists of essays and one poem on a variety of topics related to the field. The final section, Leaving Libraries, is only two chapters; it demonstrates that the caution demonstrated by all those anonymous student authors is warranted. As a whole, the book is intended to show that trans and gender diverse people are not just patrons of libraries, but are also past, current, and future members of the library profession.
Ness (2023) conducted a study on bias towards trans and gender diverse people in the attitudes and behaviors of LIS workers in Illinois. Due to the nature of the survey consisting of fairly generic questions that a primarily cisgender group of respondents self-reported their attitudes and actions to, the results are of limited use without further research using different types of assessment. Ness identified a need for meaningful actions and training rather than relying on individual good intentions and broad professional standards.
Archives
Archival work is one of the areas where professional library literature moves beyond trans 101 and gets into what it does or would look like to incorporate gender inclusion into our work. Rawson (2009) discussed access barriers for trans researchers and (potentially) trans materials in archives. These barriers for patrons included environmental accessibility, such as the existence of gender neutral restrooms and what types of materials and images are on display (pp. 127-129). For materials, the largest barrier centered around description. How do we describe materials when the creator or subject did not explicitly identify as trans? How do we balance self-identification (especially for individuals who lived prior to the term “transgender” being coined) with the fact that not labeling materials relevant to the history of “people who trans-gender” may render them invisible to researchers (p. 131)? Rawson (2018) later examined the metadata for objects held in various archives that depicted “transgressive gender presentations” to discuss the rhetorical power of archival description (p. 330). He stated that this power “stems from the ability of those records to persuade us to see historical materials as they are described, to share their orientation to the past,” and then described the tension between how “unqueer” archival description can be with the need to, as a scholar of rhetoric, “queer the archive” (p. 348). Also on the topic of archival description, Wagner (2021) wrestled with issues pertaining to visual media in archives, suggesting that catalogers and archivists “embrace ambiguity,” “describe gendered ways of being and not the gender,” take caution with pre-existing description, use alternative text description and thesauri, and develop case-specific rules, all of which would explore and expand inclusion for trans people and materials with the hopes that temporary solutions will be followed by new systems of organization (pp. 30-31, 36, 39).
The bulk of professional literature on trans and gender diverse topics in archives comes from the “Archives and Archiving” special issue of Transgender Studies Quarterly, edited by Rawson and Devor (2015). The first two sections explore themes and issues in emerging and established archives. The in-scope emerging archives described are trans and gender diverse resources in the Pittsburgh Queer History Project Archive (Apple, 2015); Gender DynamiX, a trans and gender diverse archive from sub-Saharan African countries (Theron & Kgositau, 2015); and a trans and gender diverse military archive (Barnett & Hill, 2015). The three established archives discussed in the second section include materials in the University of Toronto’s Sexual Representation Collection (Matte, 2015), University of Minnesota’s Tretter Collection (Vecoli, 2015), and Schlesinger Library on the History of Women in America (Peimer, 2015). The remaining sections are largely out of scope.
Information Behavior
Taylor (2002) conducted the first study on trans and gender diverse information needs geared toward library workers. From the 316 surveys sent out, 45 usable responses were received, and the sample population of respondents was 94% white (compared to 75.1% of the US in 2004) and largely well-educated (36% held graduate degrees, compared to 8% of the US population at the time) (p. 89-90). The majority of the survey questions asked what information sources respondents consulted (internet, peers, books, etc.), how they learned about trans identity, early versus current information needs (for example, interest in researching anti-trans hate crimes increased from 4.5% to 24.4%, p. 93), and most read and recommended books. Taylor concluded by stating that the study was meant to help libraries better understand trans and gender diverse information needs, but that “we cannot draw any sweeping conclusions from these results” given the nonrandom and small sample size (p. 95).
In 2007, Beiriger and Jackson published an article on a community information needs assessment project conducted in Portland, Oregon. The intent was to learn if there were information needs in trans and gender diverse communities that were not being addressed by libraries. The results indicated that legal information and health information were the two top-ranked priorities for participants (p. 54). This study yielded 99 responses; 88% of respondents identified as white and 100% selected English as their primary language (pp. 50-51). Largely relying on the above studies to understand information needs for trans and gender diverse people, Jardine (2013) discussed access barriers and practical solutions for trans and gender diverse patrons meeting their information needs in libraries (pp. 240-241). Identified barriers include prejudice, censorship, and the inadequacy of controlled vocabularies for trans and gender diverse topics (pp. 253-258). Needs include restrooms, optional gender information on library card application forms, inclusive or gender neutral language in interactions, and inclusive policies (pp. 245-249).
Thompson (2012) observed that “attempts to promote effective library service to the LGBT community have by and large overlooked the ‘T,’or the transgender members of their communities” (p. 12). The essay served as an overview of LIS literature on information needs (referencing Taylor, 2002, and Beiriger & Jackson’s 2007 studies) and access barriers for trans and gender diverse communities, as well as resources for LIS professionals to consult in order to better meet those needs.
Drake and Bielefield (2017) conducted a study to better understand how trans and gender diverse people use and perceive libraries. They described the demographics of the study as a “close match” to the population, with 74% of the respondents identifying as non-Latine white, 6% Latine, and 4% Black (compared to the following population demographics from the 2013 US census: 62% white, 17% Latine, and 12% Black). Ninety-one of the 102 respondents were under the age of 40, 78 were assigned female at birth (note: “gender assigned at birth” was the only gender-related information shared in the article), and 96 used English as their primary language (p. 162). Slightly over a quarter of respondents stated that they visited the library in the last year specifically for trans-related research, with some reports that libraries were inadequate in meeting their information needs. Most respondents stated they did not ask for reference assistance due to fear of discrimination, a fear others demonstrated was well-founded when they described the “clear distaste” and invasive questions from library staff they interacted with (p. 164). Ninety-seven percent of respondents indicated that libraries needed to make accommodations in order for them to feel safe. The top five accommodations included: current literature and information, single stall gender neutral restrooms (non-key access), non-discrimination policies, a remote name change process, and removing or creating open text fields for gendered information on library forms (pp. 165-166).
Lyttan and Laloo (2020) discussed information seeking patterns, gender neutral restrooms, collection development, reference interactions, centering patron privacy, the need for staff training, and the idea of the library as a safe space. Despite including “transgender” in the article title, the majority of the article discusses sexual orientation along with gender identity, perhaps due to the paucity of literature focused exclusively on trans and gender diverse people and resources. The article also contains outdated language for the time, such as a section titled “Library collections related to transgenders,” and strangely classified transgender people as “the third gender” (pp. 51, 54). The article has since been retracted by the publisher due to the authors misrepresenting and altering direct quotations (“Statement of Retraction,” 2024).
Tenney et al. (2021) discussed erasure and discrimination that trans and gender diverse people experience in healthcare settings generally, and specifically in navigating breast cancer health information. They called on academic health librarians to curate content dedicated or inclusive to trans and gender diverse people, recommended culturally competent LIS education, and increased advocacy for trans and gender diverse people (pp. 138-140). Shen (2023) reviewed the Tenney, et al. article using the Evidence Based Librarianship Critical Appraisal Checklist. Shen found that the article’s validity rating was below the accepted threshold of 75%, largely due to population and data collection issues and questions around how the literature search was conducted and articles were selected (p. 128). That being said, Shen believed the health information resource gap identified and the practical implications for the LIS profession were important (p. 129).
It should be noted that there are a number of articles on general and health-specific information behavior by and about trans and gender diverse people that we considered out of scope for this literature review, as they did not focus explicitly on libraries (e.g., Adams & Peirce, 2006; Hawkins & Gieseking, 2017; Huttunen, 2023; Huttunen et al., 2019, 2020; Huttunen & Kortelainen, 2021; Ortiz-Myers & Costello, 2021; Pohjanen & Kortelainen, 2016). These pieces are frequently cited in library literature.
Cataloging
While a number of authors have addressed cataloging and classification of trans and gender diverse resources, only a few focused explicitly on the historical angle. Johnson (2010) discussed the history of subject access to trans and gender diverse materials and then compared Library of Congress Subject Headings (LCSH) with three LGBT thesauri: Michel Thesaurus, Internationale Homo- en Lesbisch Informatiecentrumen Archief (IHLIA) Thesaurus, and LGBT Life (p. 665). Johnson found that some terms used by LGBT thesauri were eventually incorporated into LCSH, which, when combined with OCLC encouraging adherence to LCSH, unfortunately led to many LGBT collections no longer developing and maintaining specialized controlled vocabularies (p. 675). It is worth noting that Johnson’s article predates Homosaurus, an international LGBTQ+ linked data vocabulary that grew out of IHLIA, by six years. While there are many articles discussing Homosaurus, they are out of scope for this literature review as they tend to focus on the entire LGBTQ+ umbrella. In 2014, Transgender Studies Quarterly published their inaugural issue containing articles focused on keywords or concepts central to transgender studies. Angell and Roberto (2014) contributed an article on the keyword “cataloging,” which discussed cataloging and controlled vocabularies, and specifically the history of the treatment of transgender topics in LCSH (pp. 53-55).
The harmful and inadequate nature of LCSH, Library of Congress Classification, and Dewey Classification when describing and classifying transgender and gender diverse resources has been discussed as well. Adler (2009) compared LCSH and user tags in LibraryThing for twenty books with trans themes (p. 319). Unsurprisingly, Adler found that the most popular tags with users were hardly represented or were non-existent on Worldcat (p. 323). In 2011, Roberto published “Inflexible Bodies: Metadata for Transgender Identities,” which argued that inaccurate and offensive description and classification creates a “passively hostile” environment for trans and gender diverse users, as well as a disconnect between the reality of our collections and their representation that “haunt library catalogs by being partially visible yet not fully manifest” (pp. 57, 63).
In recent years, there has been increased attention towards developing best practices for describing trans and gender diverse resources and how to approach describing (or not describing) gender in visual resources. After conducting a qualitative study on the gendering practices of thirteen North American catalogers, Wagner (2022) suggested a “body-oriented approach” to cataloging, stating, “the answer is not to identify the correct way a body is gendered within visual information but instead to imagine the ways gender is being communicated as an action and an idea through a body” (pp. 622, 641.) Also in 2022, the Trans Metadata Collective released “Metadata Best Practices for Trans and Gender Diverse Resources,” a set of best practices for classifying trans and gender diverse resources and individuals in cultural heritage institution settings. Meyer (2022) reviewed the guidelines, referring to them as a “timely alternative to vocabularies that misrepresent and pose harm to trans and gender diverse community members” (p. 1).
After RDA rule 9.7, which instructed catalogers to record gender information in name authority records (NARs), was adopted in 2013, there were a number of critiques published. Billey, Drabinski, and Roberto (2014) argued that RDA 9.7, which at the time required catalogers to assign authors as male, female, or “not known” with dates of transition for known trans creators, “denies the shifting and contextual nature of gender identities” fixing them into one of two binary genders and ignoring the many gender identities that fall outside of the binary (pp. 414, 417). The article also delved into examples of errors and other complications with recording gender in NARs that had, at the time of writing, already been found (pp. 418-419). Ultimately, the authors called for the rule to record gender to be rescinded (p. 420).
In 2016, Thompson reviewed 50 NARs for trans creators and found that 65% contained some form of outing information in the 375 or 670 field, including mentions of “sex change operation[s]” and other details about creators’ medical histories, identities, and changing pronouns (p. 149). Thompson demonstrated that recording gender in NARs frequently outs trans people and that fields beyond the 375 “warrant further scrutiny from the cataloging community” (p. 153).
Billey and Drabinski (2019) provided an overview of the history of name authority control and updates about RDA 9.7. The article discussed a 2015 proposal to add the term “transgender” to the list of possible gender options, before a new proposal was submitted to the RDA Steering Committee meeting to record gender in NARs without predefined terms. The Program for Cooperative Cataloging’s (PCC) Ad Hoc Task Group on Gender in Name Authority Records produced a best practices report on how to carry out this work in 2016 (pp. 121-122).
Ethical Questions in Name Authority Control, edited by Sandberg (2019), contains several chapters addressing RDA 9.7 and the 2016 Report of the PCC Ad Hoc Task Group on Gender in Name Authority Records. Adolpho’s (2019) chapter stated that the Report’s “proposed best practices are still rooted in Western-centric, cisnormative understandings of gender that deny gender diverse people both agency over our own identities and decision making power over the frameworks that seek to include us” (p. 113). Polebaum-Freeman (2019) argued that “authority record catalogers consider transgender authors puzzles that need solving,” and that they “made purposeful, transphobic decisions about the nature of the metadata selected for inclusion” in NARs for trans and gender diverse people (pp. 171, 156). Shiraishi’s (2019) chapter used a philosophy of language approach to NARs, discussing how self-identification and publicly disclosed information may contradict one another alongside issues with assigning dates to gender, emphasizing the potential social impact and harm in recording gender. Wagner’s (2019) chapter discussed issues around “naming and unnaming queerness” for historical figures when it may not be clear how they self-identified, as well as issues with anachronistic language (p. 197). Overall, while it seemed possible for the proposed best practices to be revised to do less harm, the only way to do no harm would be to rescind the rule, which ultimately occurred in 2022 (Billey et al., 2022).
Conclusion
Clearly, we have work to do as a profession. Aside from examples in archives and cataloging, library literature has seldom strayed beyond trans 101 content. Some of us—those who are already well aware of how few libraries have implemented basic gender inclusion practices—may find this unsurprising. It can be difficult to imagine future possibilities when so many library workers cannot even go to the restroom or fill out human resources paperwork without being forced to misgender ourselves, and when we must use systems that out and misgender patrons at every step. And so, gaps in library practices go hand in hand with gaps in the literature.
And the gaps are enormous. Some topics have been covered in the past, but need updating, while others have received only little attention. Other topics, such as restrooms, are barely covered at all outside of magazine articles and blog posts. The literature we found focuses largely on academic libraries (we suggest that this is related to who is permitted and encouraged to research and publish as part of their jobs). There have been no U.S.-based case studies on gender inclusion in public libraries, and the ways in which they can best support and serve trans and gender diverse patrons has been little explored in the scholarly literature (though it is certainly a topic of discussion through other avenues).
Even in academic libraries, writing on gender inclusion occasionally delves deeper than surface-level from one angle or another, but it largely reiterates the basics. This is not a critique on the authors of these works; on the contrary, it demonstrates that the field as a whole has made little progress, and reminding academic library workers that trans and gender diverse people exist at all is still necessary. Wagner and Crowley’s (2020) point that holding a value does not equate to doing the work to uphold it is one that academic libraries—and others—need to pay attention to. There is a fair bit of talk about being inclusive, and sometimes even discussion of what that looks like in practice. But it seems that the actual work remains limited or nonexistent in most places.
There has been very little in the literature acknowledging the fact that trans and gender diverse people are library workers in addition to being library patrons, and our experiences in the profession have hardly been discussed until recently. Personal accounts from trans and gender diverse people of color, people with disabilities, and people who work outside of academic and public libraries are still underrepresented. As stated previously, the fact that there is so little literature by trans and gender diverse people on our experiences in the profession stems at least partially from safety issues around very publicly and irrevocably coming out as trans or gender diverse. Even anonymous accounts may be recognizable, especially for individuals who are multiply marginalized. Other trans and gender diverse library workers may simply not feel like writing publicly about their personal experiences, and should not be expected to do so.
The few studies on information behavior that are tied to libraries (instead of information behavior more broadly) focus on how trans and gender diverse people discover our identities and learn about issues. While literature on information behavior and barriers has shifted somewhat over time to focus more on perceptions of libraries and what librarians can actively do to remove these barriers, there is still much work to be done. For starters, the demographics of respondents from the three studies we found were overwhelmingly white trans and gender diverse people with graduate degrees who spoke English as their primary language. Moreover, the studies all had fairly small and/or non-representative sample sizes, which makes it difficult to generalize the results and leaves out many patron communities.
As stated previously, description in cataloging and archives is the most developed area in the literature. While earlier literature on gender inclusion in cataloging largely focused on history and examples of exclusions and harm, in recent years we have seen a shift in focus towards developing and implementing best practices. Outside of the Transgender Studies Quarterly issue, most professional literature on archives addresses description and other access barriers. While there is still room for growth in these areas, they have received the most consideration thus far.
There are too many gaps in the literature for us to list them all. Even the most detailed of the topics covered here have all sorts of work left to do; most of the others have yet to move past trans 101. Many areas of library work do not appear in this review at all because nothing has been published on them in the scholarly literature. As a summary, here are some of the many possible areas of future research, some of which are new and some of which build on the literature covered above.
- General: restroom options and usage in all types of libraries and the impact on trans and gender diverse people; intersectional studies on trans and gender diverse information behavior, access barriers, and needs in libraries; gender inclusion in library work that goes beyond the colonial gender system (which attempts to map everyone along the binaries of man/woman, cis/trans, and binary/non-binary, excluding Indigenous gender systems and other ways of being); gender inclusion (or the lack of it) in LIS education.
- Public libraries: meeting room issues related to anti-trans speakers, youth services, book bans
- Academic libraries: gender inclusive instruction and research support, collection development of materials on trans and gender diverse people, campus-wide limitations and opportunities around gender inclusion in the library
- Library workers: gender demographics of the library profession, surveys on the experiences of trans and gender diverse library workers (including student workers), institutional efforts around gender inclusion, anti-trans bias and transantagonistic behavior by library workers, career tracking of trans and gender diverse library workers
- Archives: collection development, relationship building, practical workflows and policies for gender inclusion
- Cataloging: how to discuss (or not discuss) gender information in the 670 field of NARs (an issue raised by Thompson in 2016 and acknowledged in the 2022 PCC report as an area for future work), the history of subject analysis for materials on Indigenous and non-colonial genders
- Types of libraries and areas of library work not mentioned in the review: literally anything.
The most effective approach to making libraries safer and more welcoming for trans and gender diverse people is fairly simple in concept, largely because the bar is currently so low as to be nonexistent. Essentially, the library profession needs to start behaving as though it knows that trans and gender diverse people exist. All library workers must learn the basics of gender inclusion, and to apply those practices to their work. That goes for trans and gender diverse library workers too–being of an identity does not automatically transfer expertise on workplace practices, and the wide variety of trans and gender diverse people means that any two of us may or may not have many common experiences.
Filling the gaps in the scholarly literature is more difficult. Every library worker needs to learn the basics in order to be considered competent at their work, otherwise they end up harming and excluding trans and gender diverse patrons and coworkers. But the level of self-education needed to conduct research on or about trans and gender diverse people is significantly higher—it is insufficient to know that gender has no place on a library card application, or how to give instructions to the restroom without misgendering a patron. We don’t need more trans 101 articles (well, we do, probably—see that low, low bar—but our hope in writing this literature review is that we also get to move on to more interesting and impactful issues). The past and present of gender diversity is too huge and complicated a subject for knowing everything to be a realistic expectation for future researchers; transgender studies is an entire field in its own right. At the very least, though, one should be aware of the scale of the topic and the relevant specifics before deciding to conduct original research.
Library workers are not in a position of neutrality regarding gender inclusion; in most cases, they are enacting exclusion and harm, intentionally or not. This article is partly intended as a call to action, but it is not enough to mean well. We need people to fill the many enormous gaps in the literature; in order to do that, we need people to do the work of extensive self-education first. Perhaps then we can move beyond an endless series of trans 101 articles pleading for harm reduction, and into a future where libraries truly welcome and support trans and gender diverse people.
We envision a library profession in which nobody needs to write another trans 101 article for their specialty because that knowledge is assumed to be the norm, a baseline for anyone working in libraries. In which people can instead pursue whatever gloriously niche aspect of gender inclusion brings them joy. In which trans and gender diverse library students and workers can choose whether or not to be out based on personal preference, not concerns for safety and job security. We hope that the next time someone attempts to do a literature review on this topic, they find that they cannot possibly fit all the new material into one article. We ask for your help in making that future come true.
- Trans and gender diverse people is an umbrella term that includes trans people, as well as anyone whose gender does not fall into the binaries of man or woman and trans or cis (meaning, those who identify with the gender they were assigned at birth)
︎
- Gender inclusion means practices that result in equitable treatment for people of all genders. We do not specify trans and gender diverse inclusion because there is no such thing as gender inclusive behavior that applies only to cis people.
︎
- This may be a good place to note that this article does not intend to provide introductory information about queer identities. If you need this acronym spelled out or don’t have a working understanding of gender identity basics, much of the following material won’t make sense until you have filled that knowledge gap.
︎
Acknowledgements
We would like to express our sincere thanks to our article’s external peer reviewer, nicholae cline, internal peer reviewer, Jessica Schomberg, and Lead Pipe Publishing Editors Brittany Paloma Fiedler and Ryan Randall. We would also like to thank Anastasia Chiu and Adrian Williams for offering feedback on our draft before submission. This literature review benefited greatly from all their labor. Additionally, we’d like to share our gratitude to K.J. Rawson for writing or editing the bulk of the existing literature on trans and gender diverse inclusion in archives, K.R. Roberto for writing the first scholarly piece (that we could find) by a trans or gender diverse person on our experiences in the profession, the Trans Metadata Collective and Travis Wagner for their work on creating practical guidance on gender inclusive metadata and cataloging, and everyone who helped end RDA 9.7.
References
(2024) Statement of retraction: Equitable access to information in libraries: A predicament for transgender people. Journal of Access Services, 21(1), 68-68, DOI: 10.1080/15367967.2024.2314905
Adams, S. S., & Peirce, K. (2006). Is there a transgender canon?: Information seeking and use in the transgender community. Proceedings of the Annual Conference of the Canadian Association of Information Science 2006, 1–7. https://doi.org/10.29173/cais161
Adler, M. (2009). Transcending library catalogs: A comparative study of controlled terms in Library of Congress Subject Headings and user-generated tags in LibraryThing for transgender books. Journal of Web Librarianship, 3(4), 309–331. https://doi.org/10.1080/19322900903341099
Adolpho, K. K. (2019). Who asked you? Consent, self-determination, and the report of the PCC Ad Hoc Task Group on Gender in Name Authority Records. In J. Sandberg (Ed.), Ethical questions in name authority control, 111–131. Library Juice Press.
Adolpho, K. K., Krueger, S. G., & McCracken, K. (Eds.). (2023). Trans and gender diverse voices in libraries. Library Juice Press.
Angell, K., & Roberto, K. R. (2014). Cataloging. Transgender Studies Quarterly, 1(1–2), 53–56. https://doi.org/10.1215/23289252-2399587
Apple, H. (2015). The $10,000 woman: Trans artifacts in the Pittsburgh Queer History Project archive. Transgender Studies Quarterly, 2(4), 553-564.
Austin, J. (2019). Lines of sight and knowledge: Possibilities and actualities of transgender and gender-nonconforming youth in the library. In Mehra, B. (Ed.), LGBTQ+ librarianship in the 21st Century: Emerging directions of advocacy and community engagement in diverse information environments, 167–196.
Barnett, J. T. & Hill, B. J. (2015). Covert Operation: Archiving the experiences of transgender service members in the US military. Transgender Studies Quarterly, 2(4), 584-594.
Beiriger, A., & Jackson, R. M. (2007). An assessment of the information needs of transgender communities in Portland, Oregon. Public Library Quarterly, 26(1/2), 45-60. https://doi.org/10.1300/J118v26n01_03
Billey, A., Colbert, J., Haugen, M., Hostage, J., Ilik, V., Sack, N., & Schiff, A. (2022, April 7). Revised report on recording gender in personal name authority records. Library of Congress. https://www.loc.gov/aba/pcc/documents/gender-in-NARS-revised-report.pdf.
Billey, A., & Drabinski, E. (2019). Questioning authority: Changing library cataloging standards to be more inclusive to a gender identity spectrum. Transgender Studies Quarterly, 6(1), 117–123. https://doi.org/10.1215/23289252-7253538
Billey, A., Drabinski, E., & Roberto, K. R. (2014). What’s gender got to do with it? A critique of RDA rule 9.7. Cataloging & Classification Quarterly, 52(4), 412–421. https://doi.org/10.1080/01639374.2014.882465
Billey, A., Haugen, M., Hostage, J., Sack, N., & Schiff, A. L. (2016, October 4). Report of the PCC Ad Hoc Task Group on gender in name authority records. Library of Congress. https://www.loc.gov/aba/pcc/documents/Gender_375%20field_RecommendationReport.pdf.
Burns, J., Cronquist, M., Huang, J., Murphy, D., Rawson, K. J., Schaefer, B., Simons, J., Watson, B. M., & Williams, A. (2022). Metadata best practices for trans and gender diverse resources. Librarian Publications & Presentations, 52. https://digitalcommons.osgoode.yorku.ca/librarians/52
Byrne, A. (2020). Organizational change and gender identity: When good intentions fall short. In H. L. Seibert, A. Vinogradov, & A. H. McClellan (Eds.), The library workplace idea book: Proactive steps for positive change, 91-94. ALA Editions.
Doherty, M. T., & Coghill, D. E. (2020). Virginia Commonwealth University Libraries’ Gender-Inclusive Work Group experience. In H. L. Seibert, A. Vinogradov, & A. H. McClellan (Eds.), The library workplace idea book: Proactive steps for positive change, 95-100. ALA Editions.
Drake, A. A., & Bielefield, A. (2017). Equitable access: Information seeking behavior, information needs, and necessary library accommodations for transgender patrons. Library & Information Science Research, 39(3), 160–168. https://doi.org/10.1016/j.lisr.2017.06.002
Hawkins, B., & Gieseking, J. J. (2017). Seeking ways to our transgender bodies, by ourselves: Rationalizing transgender-specific health information behaviors. Proceedings of the Association for Information Science and Technology, 54, 702–704. https://doi.org/10.1002/pra2.2017.14505401122
Huttunen, A. (2023). “I had to teach my own doctor what this was about”: Information sharing barriers and information evaluation of Finnish transgender people. Library & Information Science Research, 45(2). https://doi.org/10.1016/j.lisr.2023.101235
Huttunen, A., Kähkönen, L., Enwald, H. & Kortelainen, T. (2019). Embodied cognition and information experiences of transgender people. Information Research, 24(4). http://InformationR.net/ir/24-4/colis/colis1940.html
Huttunen, A., Hirvonen, N., & Kähkönen, L. (2020). Uncomfortable in my own skin – emerging, early-stage identity-related information needs of transgender people. Journal of Documentation, 76(3), 709–729. https://doi.org/10.1108/JD-09-2019-0193
Huttunen, A., & Kortelainen, T. (2021). Meaning‐making on gender: Deeply meaningful information in a significant life change among transgender people. Journal of the Association for Information Science & Technology, 72(7), 799–810. https://doi.org/10.1002/asi.24447
Jardine, F. (2013). Inclusive information for trans* persons. Public Library Quarterly, 32(3), 240–262. https://doi.org/10.1080/01616846.2013.818856
Johnson, M. (2010). Transgender subject access: History and current practice. Cataloging & Classification Quarterly, 48(8), 661–683. https://doi.org/10.1080/01639370903534398
Keralis, S. D. C. (2023). Is the library a gendered place? Journal of Library Administration, 63(2), 284–289. https://doi.org/10.1080/01930826.2022.2159246
Krueger, S. G. (2019). Supporting trans people in libraries. Libraries Unlimited.
Krueger, S., & Matteson, M. (2017). Serving transgender patrons in academic libraries. Public Services Quarterly, 13(3), 207–216. https://doi.org/10.1080/15228959.2017.1338543
Krutkowski, S., Taylor-Harman, S., & Gupta, K. (2019). De-biasing on university campuses in the age of misinformation. Reference Services Review, 48(1), 113–128. https://doi.org/10.1108/RSR-10-2019-0075
Leahy, A. (2023). Developing a collection around transgender life-writing at Senate House Library, University of London. Collection & Curation, 42(3), 94–98. https://doi.org/10.1108/CC-10-2022-0035
Lockmiller, C. (2023). Decoding the misinformation-legislation pipeline: An analysis of Florida Medicaid and the current state of transgender healthcare. Journal of the Medical Library Association, 111(4), 750–761. https://doi.org/10.5195/jmla.2023.1724
Lyttan, B., & Laloo, B. (2020). Equitable access to information in libraries: A predicament for transgender people. Journal of Access Services, 17(1), 46–64. https://doi.org/10.1080/15367967.2019.1671850
Marrall, R. M. (2015). Developing best practices for serving transgender patrons at academic library service points. ACRL 17th National Conference. 184–191. https://alair.ala.org/handle/11213/17848
Marquez, A. (2014). Supporting transgender individuals in libraries: Developing responsive policies. The Journal of Creative Library Practice.
Matte, N. (2015). Without a minority/identity framework: Highlighting trans archival materials at the University of Toronto’s Sexual Representation Collection. Transgender Studies Quarterly, 2(4), 595-606.
Meyer, B. (2022) Review of metadata best practices for trans and gender diverse resources. VRA Bulletin, 49(2). Article 3. https://online.vraweb.org/index.php/vrab/article/view/224/224
Ness, C. “Dismantling structural and individual cisgenderism in Illinois libraries: A descriptive research study on cisnormativity, transprejudice and biases against transgender and nonbinary populations.” Reference Services Review. https://doi.org/10.1108/RSR-03-2023-0031.
Ortiz-Myers, M., & Costello, K. L. (2021). The information practices of parents of transgender and non-binary youth: An exploratory study. Library Trends, 70(2), 1–29. https://doi.org/10.1353/lib.2021.0018
Peimer, L. (2015). Trans* collecting at the Schlesinger Library: Privacy protection and the challenges of Description and access. Transgender Studies Quarterly, 2(4), 614-620.
Pohjanen, A. M., & Kortelainen, T. A. M. (2016). Transgender information behavior. Journal of Documentation, 72(1), 172–190. https://doi.org/10.1108/JD-04-2015-0043
Polebaum-Freeman, H. (2019). Violent cis-tems: Identifying transphobia in Library of Congress name authority records. In J. Sandberg (Ed.), Ethical questions in name authority control, 155–179. Library Juice Press.
Pun, R., Flash, K. S., Nangle, D., & Hernandez Perez, J. (2017). Libraries and gender neutral / inclusive bathrooms: Case studies on promoting information, inclusivity and access in open spaces. IFLA WLIC, Wrocław, Poland. http://library.ifla.org/1670/
Rawson, K. J. (2009). Accessing transgender // desiring queer(er?) archival logics. Archivaria, 68, 123–140.
Rawson, K. J. (2018) The rhetorical power of archival description: Classifying images of gender transgression. Rhetoric Society Quarterly, 48(4), 327-351. https://doi.org/10.1080/02773945.2017.1347951
Rawson, K. J., & Devor, A. (Eds.). (2015). Archives and archiving. Transgender Studies Quarterly, 2(4).
Roberto, K. R. (2011). Passing tips and pronoun police: A guide to transitioning at your local library. In T. Nectoux (Ed.), Out behind the desk: Workplace issues for LGBTQ librarians, 121–127. Library Juice Press.
Roberto, K. R. (2011). Inflexible bodies: Metadata for transgender identities. Journal of Information Ethics, 20(2), 56–64. https://doi.org/10.3172/JIE.20.2.56
Rosa, K., & Henke, K. (2017). 2017 ALA demographic survey [Text]. ALA Office for Research and Statistics. https://www.ala.org/tools/research/initiatives/membershipsurveys
Sancho-Brú, E., McIntyre, P., & Raventós, I. B. (2019). Bringing the trans and local community together: The “Trans Identities and Gender” project. Advances in Librarianship, 45, 243–270. https://doi.org/10.1108/S0065-283020190000045018
Sandberg, J. (Ed.) (2019). Ethical questions in name authority control. Library Juice Press.
Shen, L. (2023). Transgender and gender-nonconforming populations experience unique challenges in health information environment developed for heteronormative audience. Evidence Based Library & Information Practice, 18(1), 127–129. https://doi.org/10.18438/eblip30289
Shiraishi, N. (2019). Accuracy of identity information and name authority records. In J. Sandberg (Ed.), Ethical questions in name authority control, 181–194. Library Juice Press.
Smith-Borne, H. (2017). Best practices for creating a welcoming environment for transgender and gender non-conforming individuals in libraries. Brick & Click: An Academic Library Conference, 109–118. https://files.eric.ed.gov/fulltext/ED578189.pdf#page=116
Smith-Borne, H. (2019). Creating a welcoming and inclusive environment for transgender and gender fluid music library users. Music Reference Services Quarterly, 22(1/2), 18–29. https://doi.org/10.1080/10588167.2018.1536691
Sokoll, T. (2013). Representations of trans* youth in young adult literature: A report and a suggestion. Young Adult Library Services, 11(4), 23–26.
Taylor, J. K. (2002). Targeting the information needs of transgender individuals. Current Studies in Librarianship, 26(1/2), 85–110.
Tenney, C. S., Surkan, K. J., Hammond Gerdido, L., & Betts-Green, D. (2021). A crisis of erasure: Transgender and gender-nonconforming populations navigating breast cancer health information. The International Journal of Information, Diversity, & Inclusion, 5(4), 132-149. https://doi.org/10.18438/eblip30289.
Theron, L., & Kgositau, T. R. (2015). The emergence of a grassroots African trans archive. Transgender Studies Quarterly, 2(4), 578-583.
Thompson, K. J. (2012). Where’s the “t”?: Improving library service to community members who are transgender- identified. B Sides, 1–17.
Thompson, K. J. (2016). More than a name: A content analysis of name authority records for authors who self-identify as trans. Library Resources & Technical Services, 60(3), 140-155. https://doi.org/10.5860/lrts.60n3.140.
Vecoli, L. (2015). The Tretter Collection: What we have, what’s missing, and the challenges of trans history. Transgender Studies Quarterly, 2(4), 607-613.
Wagner, T. L. (2019). Finding “Miss Betty” Joe Carstairs: The ethics of unpacking misnaming in cataloging and biographical practices. In J. Sandberg (Ed.), Ethical questions in name authority control, 195–211. Library Juice Press.
Wagner, T. L. (2021). “She started wearing men’s clothes and acting more masculine”: Queering historical knowledge, gendered identity making, and trans potentialities in visual information. The International Journal of Information, Diversity, & Inclusion, 5(4), 21–42. https://www.jstor.org/stable/48641978
Wagner, T. L., & Crowley, A. (2020). Why are bathrooms inclusive if the stacks exclude?: Systemic exclusion of trans and gender nonconforming persons in post-Trump academic librarianship. Reference Services Review, 48(1), 159–181. https://doi.org/10.1108/RSR-10-2019-0072
Wagner, T. (2022). Body-oriented cataloging as a method of inclusive gender representation. Cataloging & Classification Quarterly, 60(6–7), 620–645.
Comment Policy
In the Library with the Lead Pipe welcomes substantive discussion about the content of published articles. This includes critical feedback. However, comments that are personal attacks or harassment will not be posted and repeat offenders will be blocked from commenting at the discretion of the editorial board. All comments are moderated before posting to ensure that they comply with the Code of Conduct. The editorial board reviews comments on an infrequent schedule (and sometimes WordPress eats comments), so if you have submitted a comment that abides by the Code of Conduct and it hasn’t been posted within a week, please email us!
Open Knowledge Network: Introducing the Regional Hubs Prototype Programme / Open Knowledge Foundation
We’re thrilled to unveil today the latest addition to the Open Knowledge Network: the Regional Hubs Prototype Programme! This programme aims to foster collaboration and expand our reach and impact within our diverse community spread across the globe, unlocking the full potential of our Network. The Regional Hubs will serve as a vital nexus for driving our mission of creating a fair, sustainable and open digital future, advancing open knowledge as a design principle everywhere.
Find out all about this programme below.
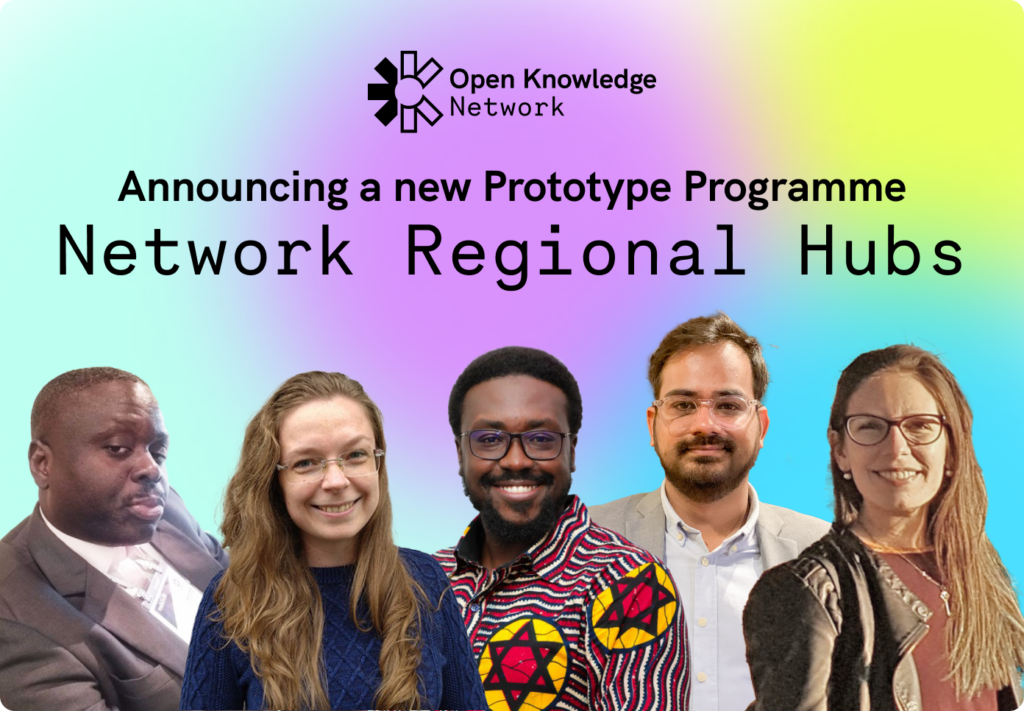
What is the Regional Hubs Prototype Programme?
Over the last 2 years, the Open Knowledge Network has been growing consistently, and a lot of it is happening in regions that we at OKFN know less well than others, and around new topics too. For some time we have felt the need to be closer to our members, so we started prototyping this programme.
At its core, the Network Regional Hubs are designed to cultivate a network of individuals committed to advancing open knowledge principles in their respective regions. With five hubs strategically located across different continents, this initiative seeks to better understand the local specificities, use languages other than English to advocate for open knowledge, and amplify our collective voice.
What are the main goals of this programme?
This is an 11-month prototype programme ending in March 2025. Throughout the year, the coordinators’ cohort will test ways to:
- Grow awareness of open knowledge in each region.
- Identify, seek out, and act on opportunities for collaboration with local entities: NGOs, activists, open advocates, and government.
- Be closer to local Network members.
- Decentralise the Network coordination.
- Find better ways to work together.
An additional tentative objective is to explore ways for transitioning this prototype programme into a sustainable, well-funded initiative.
Who are the coordinators?
Selecting the Hub Coordinators was a difficult task. Excellent candidates applied through the open call last January. After a long process, we are happy to present five dynamic individuals chosen for their expertise, commitment, and vision for open knowledge. These coordinators bring a wealth of experience from diverse backgrounds, ranging from data advocacy and community engagement to open science and policy.
Get to know our awesome coordinators:
Anglophone Africa Hub
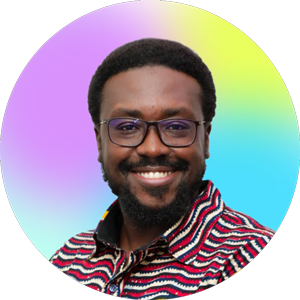
Inspiring educator, tech enthusiast, and environmental sustainability advocate. He is a co-founder of Wiki Green Initiatives, organisers of Wiki Green Conferences. He serves as Knowledge Manager of the Ghana Pidgin Wikimedia Community, preserving linguistic diversity. He actively advocates for indigenous languages as a co-organiser of the Ghanaian Languages Wikimedia Community. He manages Open Knowledge Ghana.
Maxwell holds executive roles in internet governance, including Chairperson of Climate Change and Emerging Technologies at the Internet Society Ghana Chapter. He serves as a steering committee member of the Ghana Youth Internet Governance Forum and a steering committee member of the Ghana Internet Governance Forum.
Asia Hub
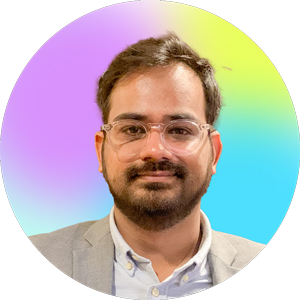
Policy practitioner with extensive experience researching and conducting legal and policy advocacy on societal impacts of technologies in the majority world. He is currently leading the Learning portfolio at the Global Network Initiative. He has previously worked with research institutes, civil society organisations, and think tanks. Setu is a public policy graduate of Central European University, and a law graduate of University of Mumbai.
Europe Hub
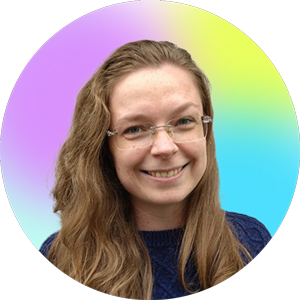
Data Steward (Delft University of Technology) and Open Science Community Member (The Turing Way, OLS, IsoArcH) based in the Netherlands. In these roles, Esther facilitates a more equitable way of knowledge generation, encouraging others to work more transparently. Her expertise includes a wide array of subjects including Open Science, Research Data Management, Open Data, FAIR principles, and Isotope Archaeology.
Francophone Africa Hub
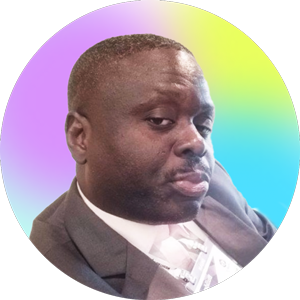
IT professional, highly active in the Open Data, Open Government, and Open Science sectors in the Democratic Republic of the Congo (DRC) and Sub-Saharan Africa. He has been involved in several projects promoting the Open Data, Open Government, Open Science movements, and digital security for many years, making positive contributions. He is the founder of the Open Data Initiative in the DRC, an initiative advocating for the openness and transparency of public data in the DRC. Narcisse is based in Kinshasa, DRC. He is fluent in English, French, Swedish, Lingala and Kikongo.
Latin America Hub
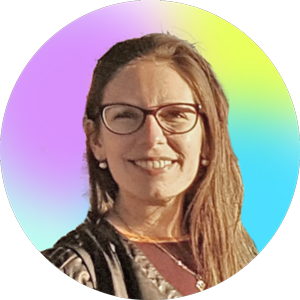
Zoologist and data scientist from Argentina. She has over 7 years of experience in academia researching the molecular mechanisms of learning and memory, and has recently moved to the private sector to work as a data scientist. Julieta is deeply interested in all the ways we can use technology to help create a more sustainable and inclusive future.
What are the immediate next steps?
The coordinators have just been incorporated, so it will take some time for them to familiarise themselves with all the tools and services offered by OKFN, and our language and broad advocacy strategy.
At first, they will be involved in mapping out their regions, and identifying the main stakeholders related to the open movement. The mappings will be shared openly over the coming months, and will also be open to collaboration.
In a second phase, the prototype programme will focus on an exploration of governance model(s) for the Network itself. The coordinators will work together with OKFN staff and Network members to research and find better ways to work together, strengthen the Network, and improve its sustainability. The outcome of this exercise will be openly published.
The coordinators will also be points of contact for developing opportunities to participate in events, alliances and other activities to advance the adoption of open knowledge. As a part of it, they will be actively participating in the production of the next Open Data Day in 2025.
In the coming weeks, we will be carrying out special deliveries of the 100+ Conversations to inspire our new direction series in which we will present each coordinator in more depth through an open dialogue with members of the Network in each region.
Ways of getting in touch
Anyone with an interest in the open movement can join the Open Knowledge Network. There is no prerequirement and we have a low barrier to entry. While we do have technical tools, they are not the main focus of the Network. What we are interested in is bringing together at the same table all different aspects of the open movement.
So if you’re keen to collaborate in your region, get in touch with them directly via their social media profiles or by emailing network@okfn.org. You can find out more about how the Network works and its current members here.
—
Finally, here’s a photo taken past week during the welcome meeting. Most of us put an image of the city/region where we’re based, or with which we identify, in the background.
It’s very gratifying to see our Network grow! Join us in welcoming the wonderful Regional Hubs Coordinators, and stay tuned for updates and opportunities to engage with them.
The Roach Motel Of Banking / David Rosenthal
![]() |
Source |
Follow me below the fold as I discuss the article and finally get to why the sting in the tail is interesting..
The article starts:
The BTM industry surged during the pandemic: The number of installed units increased more than five-fold over four years to about 31,100 units nationwide, according to Coin ATM Radar. But a closer look into the BTM boom revealed that the machines are often disproportionately located in areas with a majority of Black and Latino residents, charging fees as high as 22% per transaction.
![]() |
Wrosenb2 CC BY-SA 4.0 |
A Bloomberg review of Bitcoin Depot locations and data from the Census Bureau shows that states with proportionally large Black and Latino populations tend to have more of the company’s BTMs, especially in southern states like Georgia and Texas. Bitcoin Depot President and Chief Executive Officer Brandon Mintz dismissed any suggestion that the company targeted areas with underrepresented groups in deciding where to place its machines.But clearly there is something different about the populations of these areas:
“Never in our history have we once targeted an area based on any sort of racial profile,” Mintz told Bloomberg News. “Our focus is targeting areas that have low competition and that have populations that can support a Bitcoin ATM profitably.”
In Alabama, the concentration of Black and Latino residents within a mile radius of Bitcoin Depot BTMs is 20 percentage points higher than the broader state average, per a Bloomberg analysis of location data and the 2022 American Consumer Survey. In Dallas, BTMs are consistently located in areas where the highest percentages of Black and Latino people live.Among the mantras the crypto-bros never tire of repeating is that they are "banking the unbanked" and promoting "financial inclusion":
Proponents of cryptocurrency often tout the asset as a way to reach unbanked people, who lack a more traditional bank account. In the US, that comprised 6% of adults in 2022, per the Federal Reserve. Black and Hispanic people were more likely to be more unbanked than their White counterparts.The BTM operators are no exception:
Bitcoin Depot, the largest US operator with about 7,300 BTMs as of April 8, charges some of the highest fees in the industry while touting financial inclusion, a concept that ensures that all customers, regardless of their socioeconomic standing, have access to such financial services as savings, credit and insurance. Over 80% of Bitcoin Depot’s customers earn less than $80,000 a year, according to a November 2023 investor presentation from the company.I am a bit baffled as to how HODL-ing Bitcoin would provide "access to such financial services as savings, credit and insurance". I don't think Equifax and Trans-Union pay attention to your pseudonymous HODL-ings. And there is the matter of the fees the BTM operators charge for providing this access:
Mintz, the Bitcoin Depot CEO, said the percentage of a transaction the Atlanta-based company retains as its fees is typically in the “low twenties,” but would not provide a bottom or top boundary. “Nothing’s definitive, it just depends on the market and what we need to do to cover our expenses,” Mintz said. The company also charges a flat $3 fee on every transactionHow do the BTMs end up in locations near people lacking "financial inclusion"?
...
CoinFlip and Bitstop, Bitcoin Depot’s main rivals, charge transaction fees as high as 22%, depending on the location, according to company representatives and customer service agents. CoinFlip also charges a “network fee” of $2.49 on every transaction.
One restaurant owner in Essex, Maryland, who declined to give his name, said Bitcoin Depot paid $145 a month for the kiosk that was installed a month ago. Another store owner in New Jersey, who identified himself only as Jai, said his store received $200 a month for the kiosk, also operated by Bitcoin Depot.$145/month at 22% would be the fees on $660/month in transactions, and $200/month would be the fees on $909/month, which puts a floor on the business these BTMs do. It is likely much higher.
With all that as background, now for the sting in the tail:
The majority of BTMs — 92% of machines in the US, as indexed by Coin ATM Radar — don’t allow users to sell their crypto in exchange for cash.These machines are the Roach Motels of banking, your cash can check in but it can't check out. The question in my mind is:
What kind of customer needs to pay 22% plus $3 for "access to ... financial services" which won't let you cash out?Clearly, someone who cannot use conventional banks which, even if they do charge fees, will let you take money out. Two kinds of customers come immediately to mind:
- Criminals, who are willing to pay high fees to launder their ill-gotten cash into Bitcoin, which they will then convert into Tether at some exchange.
- Victims of crimes such as pig-butchering, where the perpetrators require payment in Bitcoin.
Library support for bibliometrics and research impact: Insights from an RLP leadership roundtable / HangingTogether
The following post is part of a series related to the provision of bibliometrics and research impact services at OCLC Research Library Partnership institutions.
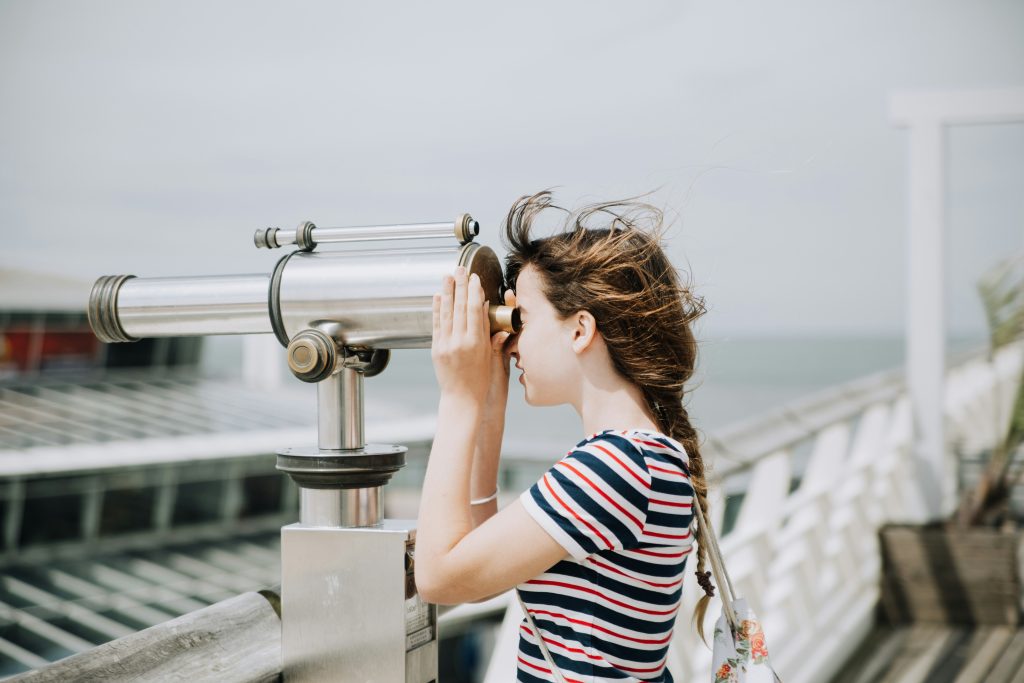
Bibliometrics and research impact (BRI) support is an emerging service category for many research libraries, with a range of activities and practices. To learn more, the OCLC Research Library Partnership convened roundtable discussions with library leaders from RLP institutions in March 2023, where participants shared about current practices and challenges in the provision of BRI services. Support for BRI is universal, but is highly dependent upon local resources, with a focus on services for researchers.
Overall, 51 individuals from 33 RLP institutions in four countries participated in one of three sessions:
Brandeis University | Temple University | University of Nevada, Reno |
Colorado State University | Tufts University | University of Notre Dame |
CUNY Graduate Center | University of Calgary | University of Pennsylvania |
George Washington University | University of Chicago | University of Pittsburgh |
Getty Library | University of Delaware | University of Sydney |
London School of Economics and Political Science | University of Delaware | University of Tennessee, Knoxville |
Monash University | University of Hong Kong | University of Texas at Austin |
Montana State University | University of Hong Kong | University of Toronto |
Ohio State University | University of Manitoba | University of Utah |
Stony Brook University | University of Maryland, College Park | University of Waterloo |
Syracuse University | University of Michigan | Virginia Tech |
One size does not fit all
Support for researchers and students
All of the institutions represented in the roundtable discussions reported some research impact activities, most frequently through education, training, and advocacy to researchers. This commonly takes the form of LibGuides on the topic. Research impact is frequently addressed in workshop offerings to researchers, in a variety of ways. Some institutions described specific workshop offerings on topics like altmetrics and understanding research impact indicators, while others described how these topics were briefly covered in workshops focused on scholarly identity and researcher profiles, citation management, or scholarly publishing.
Many institutions are also providing individual support and guidance to faculty, researchers, and students. Support for faculty preparing tenure dossiers is particularly common and valued. More than one participant said that while their library provides direct support, they do not advertise or promote these services because they would be unable to scale up if demand increased.
Support for campus units
While most efforts are directed at researchers, a small number of libraries are providing direct support to other campus units and decision support for institutional leaders, which can include:
- Institutional level analyses of research activity
- Yearly reporting and KPIs for programs and units
- Benchmarking and comparative analysis against institutional and disciplinary peers
- Competitive intelligence analysis in support of grant proposal and academic program development
These types of activities require dedicated staffing.
Managing metadata and infrastructure
Responsibilities can also extend to curating metadata to support reporting needs. A few institutions have assumed responsibility for reviewing and deprecating Scopus Author IDs for affiliated researchers, with implications for local, national, and international reporting and rankings; however, at least one institution described their approach to instead train researchers to manage their own researcher profiles.
Libraries may also play a role in supporting infrastructure that aggregates the institutional scholarly record, primarily through research information management systems (RIMS) that may serve as data sources for faculty annual activity reporting and/or tenure/promotion reports. For example, we learned that at one US institution, the local open access policy requires faculty members to deposit their research outputs into the institutional repository; the library supports and provides metadata enrichment, both to support discovery and faculty activity reporting. Furthermore, several institutions described efforts to not only encourage ORCID adoption by researchers but to also increasingly connect ORCID with campus systems like the campus directory, RIMS, and repository.
Advocacy for responsible metrics
Educating researchers as well as institutional leaders about responsible metrics and bias was mentioned as a priority at a few institutions, which can extend to the development and revision of institutional responsible data statements and position papers. A few participants described efforts to move their institutions towards adoption of the SCOPE Framework for Research Evaluation and/or formal institutional commitment to the San Francisco Declaration on Research Assessment (DORA).
Staffing and organization
Several libraries support BRI activities through librarians working in dedicated roles with titles like Research Impact Librarian or Research Information Analyst. However, the majority of institutions described an ad hoc approach that “takes a village of liaisons.” Indeed, even at institutions with a dedicated research impact librarian, there are many other librarians supporting research impact education, training, and analysis as a small component of their jobs. This can provide a way to scale the work as well as meet researchers at the point of need through a trusted liaison, but these liaisons may find it challenging to maintain a level of specialist knowledge in a rapidly changing environment.
We heard from a handful of institutions that have tried to address this challenge by making organizational changes. For example, the library at a private US university has subdivided its liaison librarians into several functional groups within scholarship and research, including research impact. The research impact team, which is comprised of a team leader, three liaison librarians, and a graduate assistant, serves as the locus for research impact work at the library, supporting both researcher and institutional needs, such as tenure dossier preparation, Scopus data cleanup, RIMS administration, and institutional reporting. The hope is that with this configuration, librarians can develop deeper expertise in fewer areas, with clearer path for skills development and career advancement.
While bibliometrics and research impact support is largely an emerging area for most RLP libraries, with few dedicated resources or discrete services, we did hear from a few libraries that have been developing BRI services–and staffing–for several years. These institutions report rapid growth, appreciation for services by users (both researchers and campus units), and the need to continuously assess, strategize, redefine, and scope. In a few cases, the library has assumed the role of campus leader and expert. Two separate Canadian libraries described how the library now plays an important campus leadership role by convening communities of practice around institutional data, comprised of users in the library, campus institutional research/analysis, the research office, and other campus units. At one US institution, the library’s BRI portfolio is supported by 4 FTE librarians plus several graduate assistants! This is certainly the exception rather than the rule, but demonstrates an area of potential growth for research libraries.
Licenses and tools
Participants briefly shared about the products their institution licenses to support BRI activities. Most institutions reported licensing one or both of the Web of Science and Scopus indexes, which are also, of course, of keen value for discovery. Other products include Journal Citation Reports, Altmetric Explorer, Lens, Overton, and OpenAlex. Several institutions reported using RIM systems like Pure, Elements, and VIVO, and/or were implementing a faculty information system like Interfolio, all with implications for metadata curation and interoperability. Furthermore, several institutions license research intelligence products like SciVal, InCites, and Dimensions. Even when another campus unit was the primary user of a product like SciVal, the library usually still manages the license, with the client user covering the costs, with the exception of Academic Analytics, which was usually purchased by a central unit like the Provost’s Office, and only at US institutions. There are also tools in use like DataBricks for ETL processing, as well as VosViewer, PowerBI, and Tableau for supporting data visualizations.
A few institutions describe the challenges of license coordination across campus. Because research institutions are characterized by self-organized independent agents working in a non-linear fashion, it’s not a big surprise to find that individual (non-library) units may license resources without enterprise coordination. This can lead to duplication, and libraries find they may have a role to play in corralling these licenses, particularly since some may be provided by vendors with which the library has preexisting relationships.
Is bibliometrics and research impact support a new service category for libraries?
I think so. After all, no other campus unit has the same expertise with bibliometric metadata. And indeed, there are a few libraries that are demonstrating how the library can take a lead in this space. I’ve blogged about some of these institutions here previously, including Virginia Tech, the University of Waterloo, the University of Pennsylvania, and Syracuse University.
But resources are a huge challenge for the majority of libraries, and many participants described a very reasonable reluctance to invest in advance of demand from both researchers and campus units. And the environment is made even more challenging by the unrelenting churn taking place in leadership roles up and down the ladder, resulting in months or even years of waiting as positions are filled, seats are pulled up to the table, and new strategies are unveiled. But most critically, other needs are simply more urgent. Support for systematic reviews was mentioned as a stronger competing priority by many participants, second only to the urgent need to support research data management activities. There is simply too much to do, and too few resources with which to do all the things.
Understanding the landscape and what’s next
RLP leadership roundtable participants learned a great deal from their peers through this effort, giving their institution a knowledge advantage when they return to think strategically about research support priorities. Many institutions without formalized research impact librarian roles or programs found solidarity with each other as well as possible partners as they contemplate future needs assessment activities.
We will next convene the research support roundtables in June will be discussing the challenges and opportunities of cross-campus collaborations in the provision of research support. I look forward to learning from our partners.
The post Library support for bibliometrics and research impact: Insights from an RLP leadership roundtable appeared first on Hanging Together.
#ODDStories 2024 @ Kigali City, Rwanda 🇷🇼 / Open Knowledge Foundation
EcoMappers, the OpenStreetMap community of Rwanda, celebrated Open Data Day on March 9, 2024, with the participation of representatives from various organizations and students. The event’s theme centered around “Mapping Nyarugenge High-Risk Zone for Disaster Preparedness,” with the goal of generating open data to aid areas within the Nyarugenge District of Kigali City, Rwanda, in their disaster preparedness efforts. The event was organized and encompassed a range of activities designed not only to contribute to open data but also to enhance the knowledge of Youth and stakeholders, empowering them in decision-making processes.
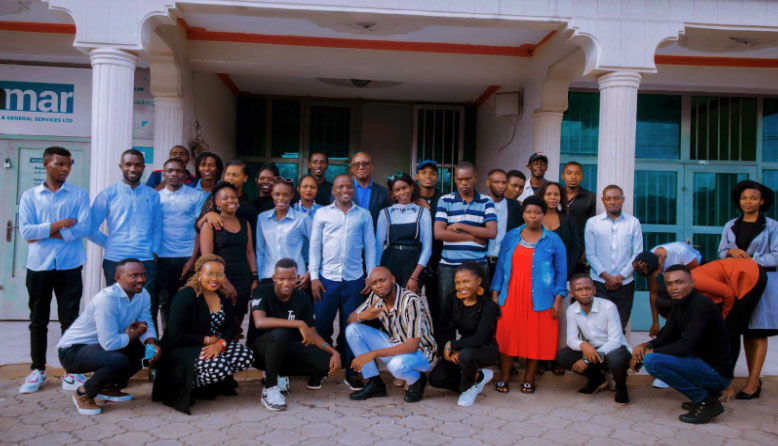
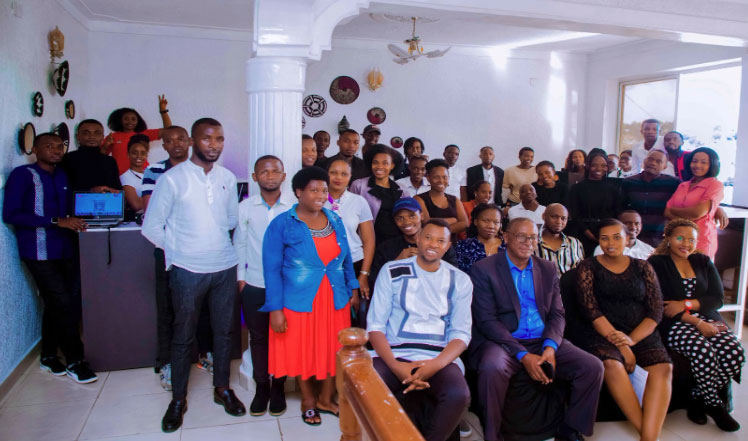
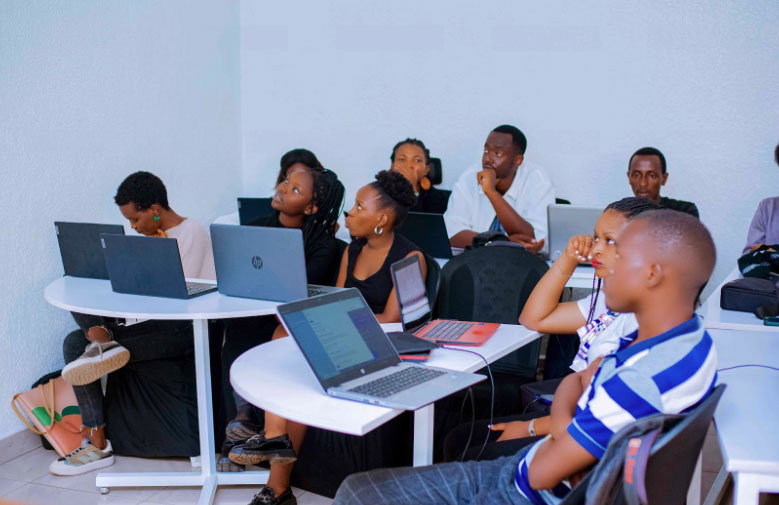
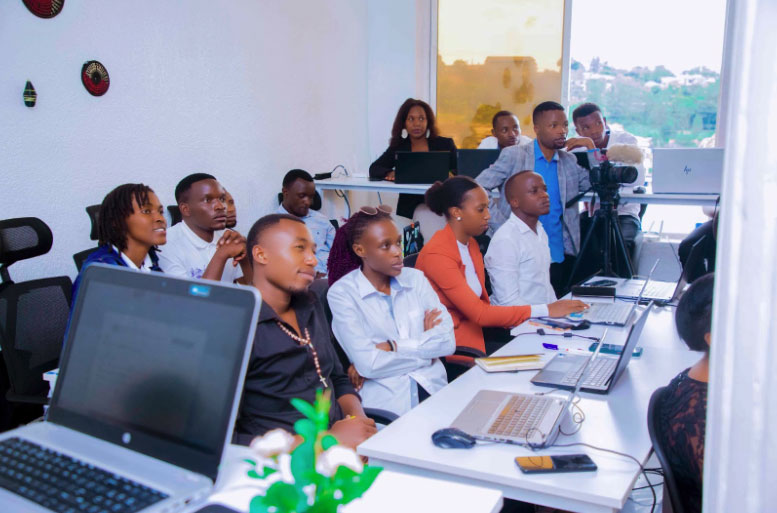
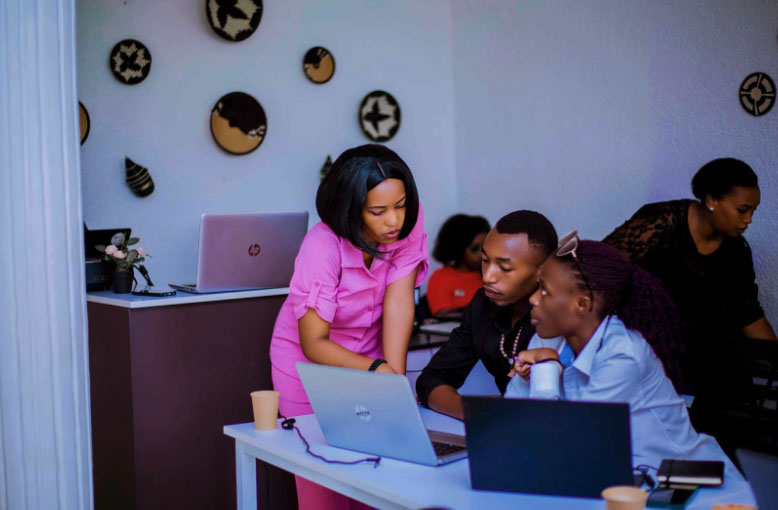
The event also served as an occasion to commemorate Open Data Day and International Women’s Day, recognizing and celebrating the significant impact of women across Rwanda. It aimed to provide training opportunities for young girls, equipping them with the skills to contribute to open data development. The emphasis was on elucidating the importance of open data for the advancement of various development initiatives, underscoring its pivotal role in fostering progress and resilience in communities.
By fostering collaboration and knowledge-sharing among diverse stakeholders, the event sought to foster a culture of data-driven decision-making and empower individuals, particularly Youths, women and young girls, to actively engage in shaping the future through open data initiatives. Through these efforts, EcoMappers, OpenStreetMap community reaffirmed their commitment to leveraging data for positive social impact and sustainable development in Rwanda and beyond.
Event Outline:
Training Sessions:
- Beginner Training: Introduction to ID Editor for contributing to Open Data development and Humanitarian Response. Participants signed up for OpenStreetMap.
- Advanced Training: Usage of Java OpenStreetMap (JOSM) Editor for advanced mapping and contribution to Open Data development and Humanitarian Response.
Guest Speakers:
- Mr. Ivan Buendía Gayton – Product Innovation Manager & Humaintain Advisor from Humanitarian OpenStreetMap, discussing open data development, free software like QGIS, and the importance of community participation for driving open-source data and knowledge.
- Ms. Hajar El Ouafi from TomTom – Speaking about the necessity of women’s inclusion and diversity in open data development.
- Mr. Esdras RWAYITARE – Emphasizing open data development and its importance, particularly in geospatial data for humanitarian response, focusing on Rwanda’s susceptibility to natural disasters.
- Mr. Bernard Hakizimana – Geospatial Expert highlighting the significant contributions of open data development to the country’s development, with a special emphasis on youth participation, considering Rwanda’s high youth population.
- Mapathon:
- An intensive Mapping Party (Mapathon) lasting three hours, wherein participants contributed to open data projects on the HOT Tasking Manager.
- Mapping Nyarugenge High-Risk Zone for Disaster Preparedness was the focus project, aimed at identifying high-risk zones in Rwanda, susceptible to disasters like flooding, landslides, volcanic eruptions, and earthquakes.
- Participants dedicated two hours to trace ground features, aiding stakeholders in utilizing the data effectively.
The celebration of Open Data Day by EcoMappers, the OpenStreetMap community of Rwanda, holds immense significance for both the present and future of our society. Through initiatives like mapping Nyarugenge High-Risk Zone for Disaster Preparedness, we underscore the unique importance of open data in addressing critical challenges such as disaster preparedness and response.
By generating and utilizing open data, stakeholders gain invaluable insights that empower informed decision-making processes, leading to more effective responses to societal needs. Also, by providing training opportunities to youth, particularly young girls, we invest in the next generation of leaders and innovators, equipping them with the skills to contribute meaningfully to open data development and sustainable progress.
The celebration of Open Data Day not only highlights the impact of data on stakeholder decision-making but also emphasizes its role in fostering community sustainability and resilience. Through collaboration and knowledge-sharing, we reinforce our commitment to leveraging open data for positive social impact and sustainable development in Rwanda and beyond.
In essence, Open Data Day serves as a reminder of the transformative power of data-driven initiatives and the collective effort required to harness this potential for the betterment of our communities. As we reflect on the successes of this celebration, let us reaffirm our dedication to promoting open data principles and advancing towards a more inclusive, transparent, and prosperous future for all.
About Open Data Day
Open Data Day (ODD) is an annual celebration of open data all over the world. Groups from many countries create local events on the day where they will use open data in their communities.
As a way to increase the representation of different cultures, since 2023 we offer the opportunity for organisations to host an Open Data Day event on the best date within a one-week period. In 2024, a total of 287 events happened all over the world between March 2nd-8th, in 60+ countries using 15 different languages.
All outputs are open for everyone to use and re-use.
In 2024, Open Data Day was also a part of the HOT OpenSummit ’23-24 initiative, a creative programme of global event collaborations that leverages experience, passion and connection to drive strong networks and collective action across the humanitarian open mapping movement
For more information, you can reach out to the Open Knowledge Foundation team by emailing opendataday@okfn.org. You can also join the Open Data Day Google Group to ask for advice or share tips and get connected with others.
Decentralized Systems Aren't / David Rosenthal
As usual, you don't need to take notes. The text of my talk with links to the sources will go up at blog.dshr.org after this seminar.
Why Decentralize?
![]() |
Tweets by language |
The platonic ideal of a decentralized system has many advantages over a centralized one performing the same functions:
- It can be more resilient to failures and attacks.
- It can resist acquisition and the consequent enshittification.
- It can scale better.
- It has the economic advantage that it is hard to compare the total system cost with the benefits it provides because the cost is diffused across many independent budgets.
Why Not Decentralize?
But history shows that this platonic ideal is unachieveable because systems decentralization isn't binary and systems that aim to be at the decentralized end of the spectrum suffer four major problems:- Their advantages come with significant additional monetary and operational costs.
- Their user experience is worse, being more complex, slower and less predictable.
- They are in practice only as decentralized as the least decentralized layer in the stack.
- They exhibit emergent behaviors that drive centralization.
What Does "Decentralization" Mean?
![]() |
Source |
The Nakamoto coefficient is the number of units in a subsystem you need to control 51% of that subsystem.
Subsystem | Bitcoin | Ethereum |
Mining | 5 | 3 |
Client | 1 | 1 |
Developer | 5 | 2 |
Exchange | 5 | 5 |
Node | 3 | 4 |
Owner | 456 | 72 |
Blockchains exemplify a more rigorous way to assess decentralization; to ask whether a node can join the network autonomously, or whether it must obtain permission to join. If the system is "permissioned" it cannot be decentralized, it is centralized around the permission-granting authority. Truly decentralized systems must be "permissionless". My title is wrong; the talk is mostly about permissionless systems, not about the permssioned systems that claim to be decentralized but clearly aren't.
TCP/IP
![]() |
IBM Cabling System |
Domain Name System
![]() |
Source |
The basis of TCP/IP is the end-to-end principle, that to the extent possible network nodes communicate directly with each other, not relying on functions in the infrastructure. So why the need for root servers and IANA? It is because nodes need some way to find each other, and the list of root servers' IP addresses provides a key into the hierarchical structure of DNS.
This illustrates the important point that a system is only as decentralized as the least decentralized layer in its stack.
LOCKSS
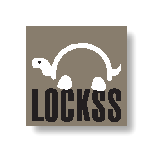
- A centralized system would provide an attractive target for litigation by the publisher oligopoly.
- The paper library system already formed a decentralized, permissionless network.
Why LOCKSS Centralized
- Software monoculture
- Centralized development
- Permissioning ensures funding
- Big publishers hated decentralization
- Although we always paid a lot of attention to the security of LOCKSS boxes, we understood that a software monoculture was vulnerable to software supply chain attacks. So we designed a very simple protocol hoping that there would be multiple implementations. But it turned out that the things that a LOCKSS box needed to do other than handling the protocol were quite complex, so despite our best efforts we ended up with a software monoculture.
- We hoped that by using the BSD open-source license we would create a diverse community of developers, but we over-estimated the expertise and the resources of the library community, so Stanford provided the overwhelming majority of the programming effort.
- The program got started with small grants from Michael Lesk at NSF, then subsequently major grants from the NSF, Sun Microsystems and Don Waters at the Mellon Foundation. But Don was clear that grant funding could not provide the long-term sustainability needed for digital preservation. So he provided a matching grant to fund the transition to being funded by the system's users. This also transitioned the system to being permissioned, as a way to ensure the users paid.
- Although many small and open-access publishers were happy to allow LOCKSS to preserve their content, the oligopoly publishers never were. Eventually they funded a completely closed network of a dozen huge systems at major libraries around the world called CLOCKSS. This is merely the biggest of a number of closed, private LOCKSS networks that were established to serve specific genres of content, such as government documents.
Gossip Protocols
If LOCKSS was to be permissionless there could be no equivalent of DNS, so how did a new node find other nodes to vote with?Wikipedia
Suppose you have a decentralized network with thousands of nodes that can join and leave whenever they want, and you want to send a message to all the current nodes. This might be because they are maintaining a shared state, or to ask a question that a subset might be able to answer. You don't want to enumerate the nodes, because it would be costly in time and network traffic, and because the answer might be out-of-date by the time you got it. And even if you did sending messages individually to the thousands of nodes would be expensive. This is what IP multicast was for, but it doesn't work well in practice. So you build multicast on top of IP using a Gossip protocol.
Each node knows a few other nodes. The first time it receives a message it forwards it to them, along with the names of some of the nodes it knows. As the alternate name of "epidemic protocol" suggests, this is a remarkably effective mechanism. All that a new node needs in order to join is for the network to publish a few "bootstrap nodes", similar to the way an Internet node accesses DNS by having the set of root servers wired in. But this bootstrap mechanism is inevitably centralized.
The LOCKSS nodes used a gossip protocol to communicate, so in theory all a library needed to join in was to know another library running a node. In the world of academic libraries this didn't seem like a problem. It turned out that the bootstrap node all the libraries knew was Stanford, the place they got the software and the support. So just like DNS, the root identity was effectively wired-in.
Bitcoin
Satoshi Nakamoto
Fast forward another ten years and Satoshi Nakamoto published Bitcoin: A Peer-to-Peer Electronic Cash System, a ledger implemented as a chain of blocks containing transactions. Like LOCKSS, the system needed a Sybil-proof way to achieve consensus, in his case on the set of transactions in the next block to be added to the chain. Unlike LOCKSS, where nodes voted in single-phase elections, Nakamoto implemented a three-phase selection mechanism:
- One node is selected from the network using Proof-of-Work. It is the first node to guess a nonce that made the hash of the block have the required number of leading zeros.
- The selected node proposes the content of the next block via the gossip network.
- The "longest chain rule", Nakamoto's most important contribution, ensures that the network achieves consensus on the block proposed by the selected node.
Increasing Returns to Scale
![]() |
Source |
The application to the Bitcoin network starts with this observation. The whole point of the Bitcoin protocol is to make running a miner, a node in the network, costly. The security of the system depends upon making an attack more costly to mount than it would gain. Miners need to defray the costs the system imposes in terms of power, hardware, bandwidth, staff and so on. Thus the protocol rewards miners with newly minted Bitcoin for winning the race for the next block.
Bitcoin Economics
Nakamoto's vision of the network was of many nodes of roughly equal power,"one CPU one vote". This has two scaling problems:- The target block time is 10 minutes, so in a network of 600 equal nodes the average time between rewards is 100 hours, or about 4 days. But in a network of 600,000 equal nodes it is about 4,000 days or about 11 years. In such a network the average node will never gain a reward before it is obsolete.
- Moore's law means that over timescales of years the nodes are not equal, even if they are all CPUs. But shortly after Bitcoin launched, miners figured out that GPUs were much better mining rigs than CPUs, and later that mining ASICs were even better. Thus the miner's investment in hardware has only a short time to return a profit.
![]() |
Mining Pools 02/27/23 |
The block rewards inflate the currency, currently by about $100M/day. This plus fees that can reach $23M/day, is the cost to run a system that currently processes 400K transactions/day, or over $250 per transaction plus up to $57 per transaction in fees. Lets talk about the excess costs of decentralization!
Like most permissionless networks, Bitcoin nodes communicate using a gossip protocol. So just like LOCKSS boxes, they need to know one or more bootstrap nodes in order to join the network, just like DNS and LOCKSS.
Haseeb Qureshi, Bitcoin's P2P NetworkThere are also fall-back nodes in case of DNS failure encoded in chainparamsseeds.h:
/** * List of fixed seed nodes for the bitcoin network * AUTOGENERATED by contrib/seeds/generate-seeds.py * * Each line contains a BIP155 serialized (networkID, addr, port) tuple. */
Economies of Scale in Peer-to-Peer Networks
![]() |
Source |
I suddenly realized that this centralization wasn't something about Bitcoin, or LOCKSS for that matter. It was an inevitable result of economic forces generic to all peer-to-peer systems. So I spent much of the weekend sitting in one of the hotel's luxurious houses writing Economies of Scale in Peer-to-Peer Networks.
My insight was that the need to make an attack expensive wasn't something about Bitcoin, any permissionless peer-to-peer network would have the same need. In each case the lack of a root of trust meant that security was linear in cost, not exponential as with, for example, systems using encryption based upon a certificate authority. Thus any successful decentralized peer-to-peer network would need to reimburse nodes for the costs they incurred. How can the nodes' costs be reimbursed?:
There is no central authority capable of collecting funds from users and distributing them to the miners in proportion to these efforts. Thus miners' reimbursement must be generated organically by the blockchain itself; a permissionless blockchain needs a cryptocurrency to be secure.And thus any successful permissionless network would be subject to the centralizing force of economies of scale.
Cryptocurrencies
![]() |
ETH miners 11/2/20 |
The fact that the coins ranked 3, 6 and 7 by "market cap" don't even claim to be decentralized shows that decentralization is irrelevant to cryptocurrency users. Numbers 3 and 7 are stablecoins with a combined "market cap" of $134B. The largest stablecoin that claims to be decentralized is DAI, ranked at 24 with a "market cap" of $5B. Launching a new currency by claiming better, more decentralized technology than Bitcoin or Ethereum is pointless, as examples such as Chia, now ranked #182, demonstrate. Users care about liquidity, not about technology.
The holders of coins show a similar concentration, the Gini Coefficients Of Cryptocurrencies are extreme.
Ethereum's Merge
![]() |
ETH Stakes 05/22/23 |
Ethereum Validators
PROOF-OF-STAKE (POS)
- A "smart contract" uses a pseudo-random algorithm to select one node and a "committee" of other nodes with probabilities based on the nodes' stakes.
- The one node proposes the content of the next block.
- The "committee" of other validator nodes vote to approve the block, leading to consensus.
Like Bitcoin, the nodes taking part in consensus gain a block reward currently running at $2.75M/day and fees running about $26M/day. This is the cost to run a distributed computer 1/5000 as powerful as a Raspberry Pi.
Validator Centralization
...
Coinbase Global Inc. is already the second-largest validator ... controlling about 14% of staked Ether. The top provider, Lido, controls 31.7% of the staked tokens,
...
US institutions issuing Ether-staking ETFs are more likely to pick an institutional digital asset custodian, such as Coinbase, while side-stepping decentralized protocols such as Lido. That represents a growing concentration risk if Coinbase takes a significant share of staked ether, the analysts wrote.
Coinbase is already a staking provider for three of the four largest ether-staking ETFs outside the US, they wrote. For the recently approved Bitcoin ETF, Coinbase was the most popular choice of crypto custodian by issuers. The company safekeeps about 90% of the roughly $37 billion in Bitcoin ETF assets, chief executive officer Brian Armstrong said
Yueqi Yang, Ether ETF Applications Spur S&P Warning on Concentration Risks
Producer Centralization
![]() |
Producers 03/18/24 |
Olga Kharif and Isabelle Lee report that these concentrations are a major focus of the SEC's consideration of Ethereum spot ETFs:
In its solicitations for public comments on the proposed spot Ether ETFs, the SEC asked, “Are there particular features related to ether and its ecosystem, including its proof of stake consensus mechanism and concentration of control or influence by a few individuals or entities, that raise unique concerns about ether’s susceptibility to fraud and manipulation?”
Software Centralization
![]() |
Source |
A bug in Ethereum's Nethermind client software – used by validators of the blockchain to interact with the network – knocked out a chunk of the chain's key operators on Sunday.The fundamental problem is that most layers in the software stack are highly concentrated, starting with the three operating systems. Network effects and economies of sclae apply at every layer. Remember "no-one ever gets fired for buying IBM"? At the Ethereum layer, it is "no-one ever gets fired using Geth" because, if there was ever a big problem with Geth, the blame would be so widely shared.
...
Nethermind powers around 8% of the validators that operate Ethereum, and this weekend's bug was critical enough to pull those validators offline. ... the Nethermind incident followed a similar outage earlier in January that impacted Besu, the client software behind around 5% of Ethereum's validators.
...
Around 85% of Ethereum's validators are currently powered by Geth, and the recent outages to smaller execution clients have renewed concerns that Geth's dominant market position could pose grave consequences if there were ever issues with its programming.
...
Cygaar cited data from the website execution-diversity.info noting that popular crypto exchanges like Coinbase, Binance and Kraken all rely on Geth to run their staking services. "Users who are staked in protocols that run Geth would lose their ETH" in the event of a critical issue," Cygaar wrote.
The Decentralized Web
One mystery was why venture capitalists like Andreesen Horwitz, normally so insistent on establishing wildly profitable monopolies, were so keen on the idea of a Web 3 implemented as "decentralized apps" (dApps) running on blockchains like Ethereum. Moxie Marlinspike revealed the reason:companies have emerged that sell API access to an ethereum node they run as a service, along with providing analytics, enhanced APIs they’ve built on top of the default ethereum APIs, and access to historical transactions. Which sounds… familiar. At this point, there are basically two companies. Almost all dApps use either Infura or Alchemy in order to interact with the blockchain. In fact, even when you connect a wallet like MetaMask to a dApp, and the dApp interacts with the blockchain via your wallet, MetaMask is just making calls to Infura!Providing a viable user experience when interacting with blockchains is a market with economies of scale and network effects, so it has centralized.
It Isn't About The Technology
What is the centralization that decentralized Web advocates are reacting against? Clearly, it is the domination of the Web by the FANG (Facebook, Amazon, Netflix, Google) and a few other large companies such as the cable oligopoly.These companies came to dominate the Web for economic not technological reasons. The Web, like other technology markets, has very large increasing returns to scale (network effects, duh!). These companies build centralized systems using technology that isn't inherently centralized but which has increasing returns to scale. It is the increasing returns to scale that drive the centralization.
![]() |
Source |
If a decentralized Web doesn't achieve mass participation, nothing has really changed. If it does, someone will have figured out how to leverage antitrust to enable it. And someone will have designed a technical infrastructure that fit with and built on that discovery, not a technical infrastructure designed to scratch the itches of technologists.I think this is still the situation.
BitTorrent
Seven years ago I wrote:Unless decentralized technologies specifically address the issue of how to avoid increasing returns to scale they will not, of themselves, fix this economic problem. Their increasing returns to scale will drive layering centralized businesses on top of decentralized infrastructure, replicating the problem we face now, just on different infrastructure.
![]() |
Source |
Blockchains
In 2022 DARPA funded a large team from the Trail of Bits cybersecurity company to publish a report entitled Are Blockchains Decentralized? which conformed to Betteridge's Law by concluding "No":Every widely used blockchain has a privileged set of entities that can modify the semantics of the blockchain to potentially change past transactions.The "privileged set of entities" must at least include the developers and maintainers of the software, because:
The challenge with using a blockchain is that one has to either (a) accept its immutability and trust that its programmers did not introduce a bug, or (b) permit upgradeable contracts or off-chain code that share the same trust issues as a centralized approach.
![]() |
Source |
A dense, possibly non-scale-free, subnetwork of Bitcoin nodes appears to be largely responsible for reaching consensus and communicating with miners—the vast majority of nodes do not meaningfully contribute to the health of the network.And second:
Of all Bitcoin traffic, 60% traverses just three ISPs.
![]() |
Source |
The Ethereum ecosystem has a significant amount of code reuse: 90% of recently deployed Ethereum smart contracts are at least 56% similar to each other.The risk isn't confined to individual ecosystems, it is generic to the entire cryptosphere because, as the chart shows, the code reuse spans across blockchains to such an extent that Ethereum's Geth shares 90% of its code with Bitcoin Core.
Decentralized Finance
![]() |
Source |
TL;DR: DeFi is neither decentralized, nor very good finance, so regulators should have no qualms about clamping down on it to protect the stability of our financial system and broader economy.DeFi risks and the decentralisation illusion by Sirio Aramonte, Wenqian Huang and Andreas Schrimpf of the Bank for International Settlements similarly conclude:
While the main vision of DeFi’s proponents is intermediation without centralised entities, we argue that some form of centralisation is inevitable. As such, there is a “decentralisation illusion”. First and foremost, centralised governance is needed to take strategic and operational decisions. In addition, some features in DeFi, notably the consensus mechanism, favour a concentration of power.
Protocol | Revenue | Market |
---|---|---|
$M | Share % | |
Lido | 304 | 55.2 |
Uniswap V3 | 55 | 10.0 |
Maker DAO | 48 | 8.7 |
AAVE V3 | 24 | 4.4 |
Top 4 | 78.2 | |
Venus | 18 | 3.3 |
GMX | 14 | 2.5 |
Rari Fuse | 14 | 2.5 |
Rocket Pool | 14 | 2.5 |
Pancake Swap AMM V3 | 13 | 2.4 |
Compound V2 | 13 | 2.4 |
Morpho Aave V2 | 10 | 1.8 |
Goldfinch | 9 | 1.6 |
Aura Finance | 8 | 1.5 |
Yearn Finance | 7 | 1.3 |
Stargate | 5 | 0.9 |
Total | 551 |
Based on the [Herfindahl-Hirschman Index], the most competition exists between decentralized finance exchanges, with the top four venues holding about 54% of total market share. Other categories including decentralized derivatives exchanges, DeFi lenders, and liquid staking, are much less competitive. For example, the top four liquid staking projects hold about 90% of total market share in that category,Based on data on 180 days of revenue of DeFI projects from Shen's article, I compiled this table, showing that the top project, Lido, had 55% of the revenue, the top two had 2/3, and the top four projects had 78%. This is clearly a highly concentrated market, typical of cryptocurrency markets in general.
Federation
![]() |
Source |
How attractive are these advantages? The first bar chart shows worldwide web traffic to social media sites. Every single one of these sites is centralized, even the barely visible ones like Nextdoor. Note that Meta owns 3 of the top 4, with about 5 times the traffic of Twitter.
![]() |
Source |
That leaves Mastodon with a total of 1.8 million monthly active users at present, an increase of 5% month-over-month and 10,000 servers, up 12%In terms of monthly active users, Twitter claims 528M, Threads claims 130M, Bluesky claims 5.2M and Mastodon claims 1.8M. Note that the only federate-able one with significant market share is owned by the company that owns 3 of the top 4 centralized systems. Facebook claims 3,000M MAU, Instagram claims 2,000M MAU, and WhatsApp claims 2,000M MAU. Thus Threads is about 3% of Facebook alone, so not significant in Meta's overall business. It may be early days yet, but federated social media have a long way to go before they have significant market share.
Summary
Radia Perlman's answer to the question of what exactly you get in return for the decentralization provided by the enormous resource cost of blockchain technologies is:a ledger agreed upon by consensus of thousands of anonymous entities, none of which can be held responsible or be shut down by some malevolent governmentThis is what the blockchain advocates want you to think, but as Vitalik Buterin, inventor of Ethereum pointed out in The Meaning of Decentralization:
In the case of blockchain protocols, the mathematical and economic reasoning behind the safety of the consensus often relies crucially on the uncoordinated choice model, or the assumption that the game consists of many small actors that make decisions independently. If any one actor gets more than 1/3 of the mining power in a proof of work system, they can gain outsized profits by selfish-mining. However, can we really say that the uncoordinated choice model is realistic when 90% of the Bitcoin network’s mining power is well-coordinated enough to show up together at the same conference?As we have seen, in practice it just isn't true that "the game consists of many small actors that make decisions independently" or "thousands of anonymous entities". Even if you could prove that there were "thousands of anonymous entities", there would be no way to prove that they were making "decisions independently". One of the advantages of decentralization that Buterin claims is:
it is much harder for participants in decentralized systems to collude to act in ways that benefit them at the expense of other participants, whereas the leaderships of corporations and governments collude in ways that benefit themselves but harm less well-coordinated citizens, customers, employees and the general public all the time.But this is only the case if in fact "the game consists of many small actors that make decisions independently" and they are "anonymous entities" so that it is hard for the leader of a conspiracy to find conspirators to recruit via off-chain communication. Alas, the last part isn't true for blockchains like Ethereum that support "smart contracts", as Philip Daian et al's On-Chain Vote Buying and the Rise of Dark DAOs shows that "smart contracts" also provide for untraceable on-chain collusion in which the parties are mutually pseudonymous.
Questions
If we want the advantages of permissionless, decentralized systems in the real world, we need answers to these questions:- What is a viable business model for participation that has decreasing returns to scale?
- How can Sybil attacks be prevented other than by imposing massive costs?
- How can collusion between supposedly independent nodes be prevented?
- What software development and deployment model prevents a monoculture emerging?
- Does federation provide the upsides of decentralization without the downsides?
Beyond Keywords: How AI Vector Search Transforms Your Search Experience / Lucidworks
Discover how AI-powered vector search goes beyond simple keyword matching to deliver significantly more relevant and intuitive search results.
The post Beyond Keywords: How AI Vector Search Transforms Your Search Experience appeared first on Lucidworks.
Empowering Communities: Open Knowledge Somalia’s Open Street Mapping Workshop / Open Knowledge Foundation
Text originally published at OK Somalia Blog.
Open Data Day is an annual global celebration of open data, and this year, Open Knowledge Somalia took part by hosting a training workshop on “Open Street Mapping” on March 25, 2024. The event, sponsored by Zamzam University of Science and Technology, featured Abdiaziz Hassan Ahmed from Open Knowledge Somalia as the lead facilitator. The workshop aimed to enlighten attendees on the fundamentals and applications of open street mapping, a collective method for creating and refining maps with freely accessible geographic data.
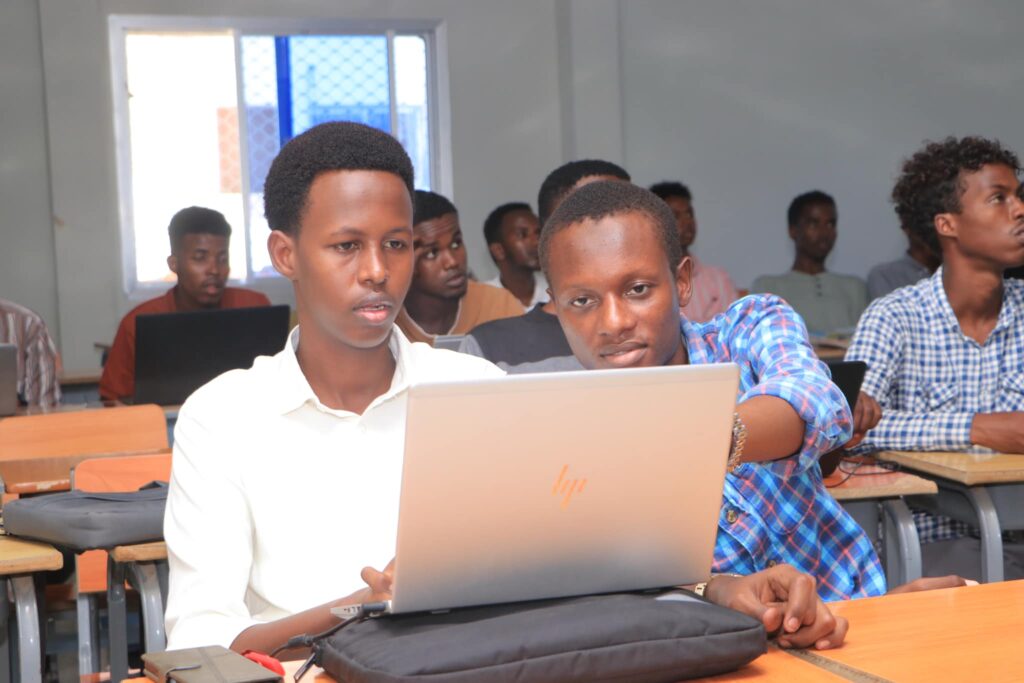
Throughout the workshop, various facets of open street mapping were explored, including its significance in disaster relief, urban planning, public health, and community development. Attendees were introduced to essential tools and methodologies for contributing to open street mapping projects, such as gathering data through GPS devices or satellite images and collaboratively editing maps on platforms like OpenStreetMap. Furthermore, the training provided participants with valuable insights and competencies in open street mapping initiatives, offering advantages for both individuals and organizations.
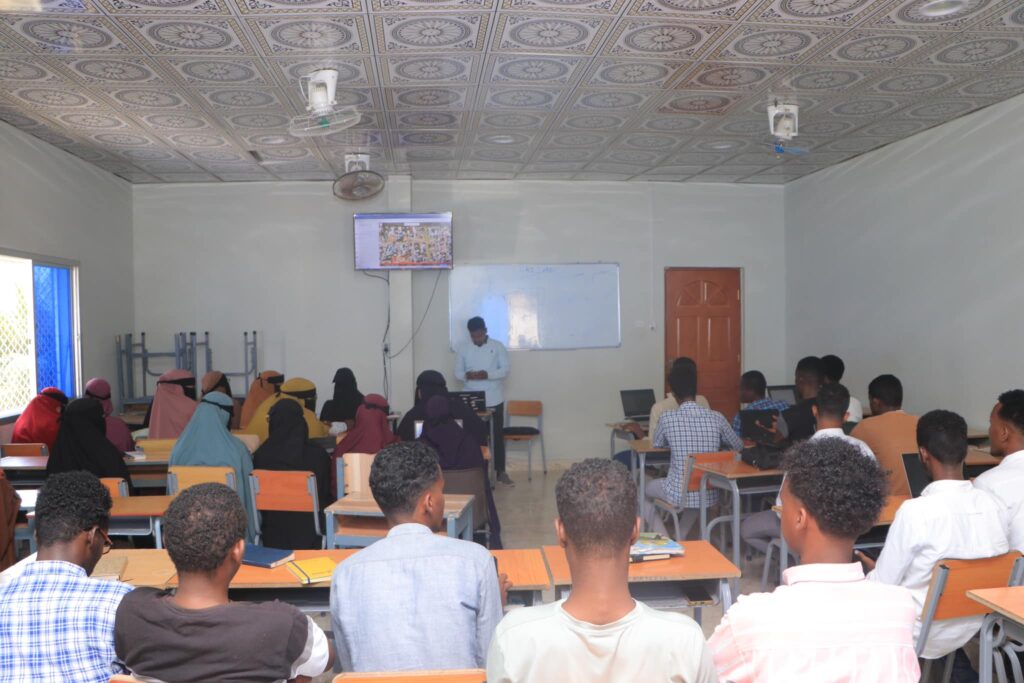
The Open Knowledge Somalia team extends its heartfelt gratitude to all participants and the facilitator for their invaluable contributions to the workshop.
About Open Data Day
Open Data Day (ODD) is an annual celebration of open data all over the world. Groups from many countries create local events on the day where they will use open data in their communities.
As a way to increase the representation of different cultures, since 2023 we offer the opportunity for organisations to host an Open Data Day event on the best date within a one-week period. In 2024, a total of 287 events happened all over the world between March 2nd-8th, in 60+ countries using 15 different languages.
All outputs are open for everyone to use and re-use.
In 2024, Open Data Day was also a part of the HOT OpenSummit ’23-24 initiative, a creative programme of global event collaborations that leverages experience, passion and connection to drive strong networks and collective action across the humanitarian open mapping movement
For more information, you can reach out to the Open Knowledge Foundation team by emailing opendataday@okfn.org. You can also join the Open Data Day Google Group to ask for advice or share tips and get connected with others.
#ODDStories 2024 @ Guatemala City 🇬🇹 / Open Knowledge Foundation
As a winning organisation of an Open Data Day 2024 mini-grant, Diálogos organised the Datos y Tarros event in Guatemala on 7 March. The objective was to open the space to promote data-based initiatives that use machine learning tools, predictive modeling, data mining, natural language processing (NLP), Internet of Things (IoT), among others.
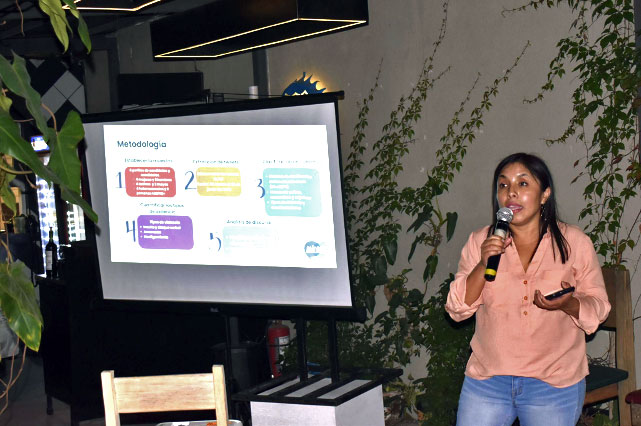
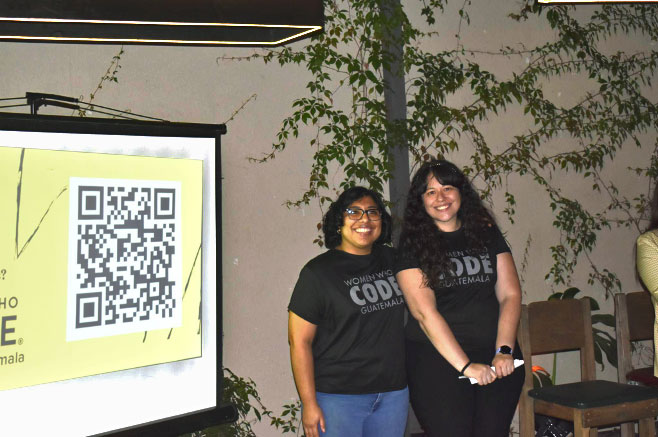
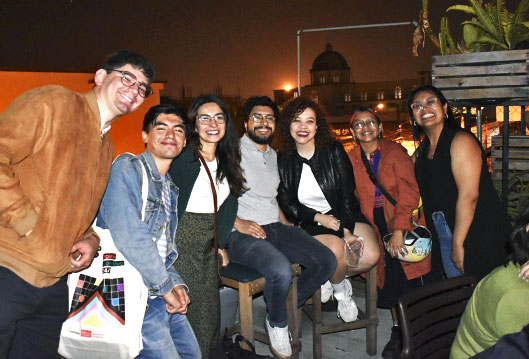
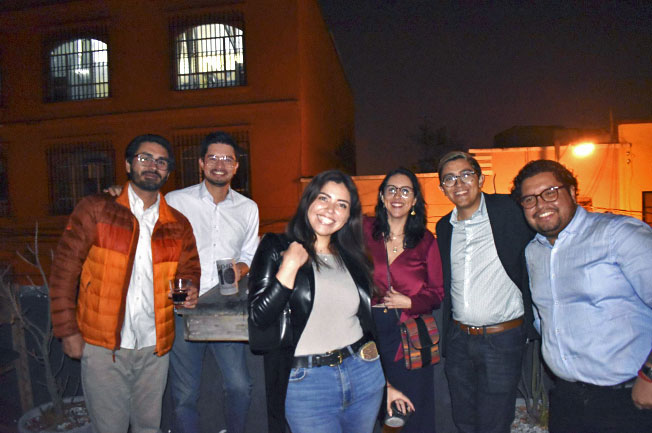
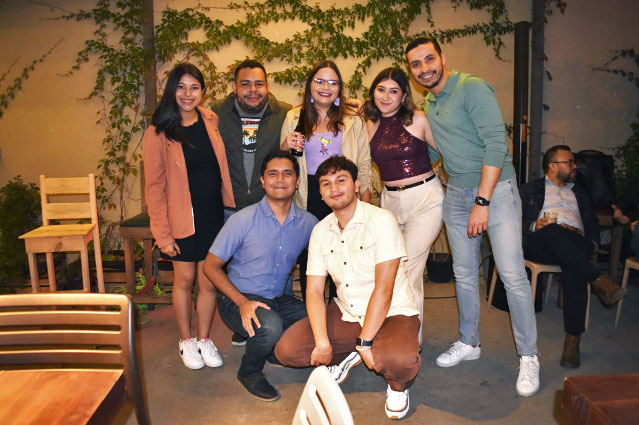
For this edition, within the framework of Open Data Day and the commemoration of International Women’s Day, we talked about research, journalism and innovation projects based on data with a gender perspective to bring to the table a conversation about the challenges that exist regarding violence and inequalities that affect women and girls differently.
We held conversations with strategic allies to join efforts and obtain better results. For this reason, we worked in alliance with Proyecto Poporopo, an open space to art, gastronomy and innovation ideas.
We made calls through our social networks to invite research, journalism and innovations projects based on data to present their results, the selection criteria were the following:
- The selected project makes use, analysis or visualization of data related to the theme of the event.
- The organization, media or individual person applies methods and/or tools in a rigorous, systematic and innovative way.
- The data was obtained transparently and through reliable and publicly accessible sources.
- The organization, media or individual respects human rights criteria and international ethical standards on the use of data.
We exchanged experiences for the collection and processing of data from a gender perspective. In addition, each project provided valuable evidence of the challenges that exist regarding violence and inequalities that affect women and girls differently.
The projects we heard about were:
- Without women there is no democracy, a project promoted by Red Ciudadana and Plaza Pública that collects and presents data analysis of the participation of women in the political sphere and shows the differences and challenges faced in achieving parity.
- Living Without Violence, an initiative of Guate Diversa e Inclusiva and launched with the support of Hivos; It consists of a portal that collects data, contains visualizations and analysis blogs on violence against women, girls and the LGBTIQ+ population.
- Women Who Code presented a “Diagnosis about the gender gap in Information Technologies”.
- The Open Justice Observatory from the civil society of the Grupo de Apoyo Xela (GAX), unveiled its web page, which focuses on accessing data from the justice sector in Guatemala to make visible how efficient is the justice system in the country attending women, the LBGTIQ+ population, children and youths, and the migrant population.
- On behalf of Diálogos we presented the “Report about violence in the interactions of the social network X (Twitter) during the electoral campaign of 2023”. This report identifies different manifestations of violence in the comments of the social media X (Twitter) to different candidates during the electoral campaign in 2023. The profiles analyzed were women, people belonging to indigenous peoples, and LGBTIQ+ communities.
About Open Data Day
Open Data Day (ODD) is an annual celebration of open data all over the world. Groups from many countries create local events on the day where they will use open data in their communities.
As a way to increase the representation of different cultures, since 2023 we offer the opportunity for organisations to host an Open Data Day event on the best date within a one-week period. In 2024, a total of 287 events happened all over the world between March 2nd-8th, in 60+ countries using 15 different languages.
All outputs are open for everyone to use and re-use.
In 2024, Open Data Day was also a part of the HOT OpenSummit ’23-24 initiative, a creative programme of global event collaborations that leverages experience, passion and connection to drive strong networks and collective action across the humanitarian open mapping movement
For more information, you can reach out to the Open Knowledge Foundation team by emailing opendataday@okfn.org. You can also join the Open Data Day Google Group to ask for advice or share tips and get connected with others.
Open Data Editor: meet the team behind the app / Open Knowledge Foundation
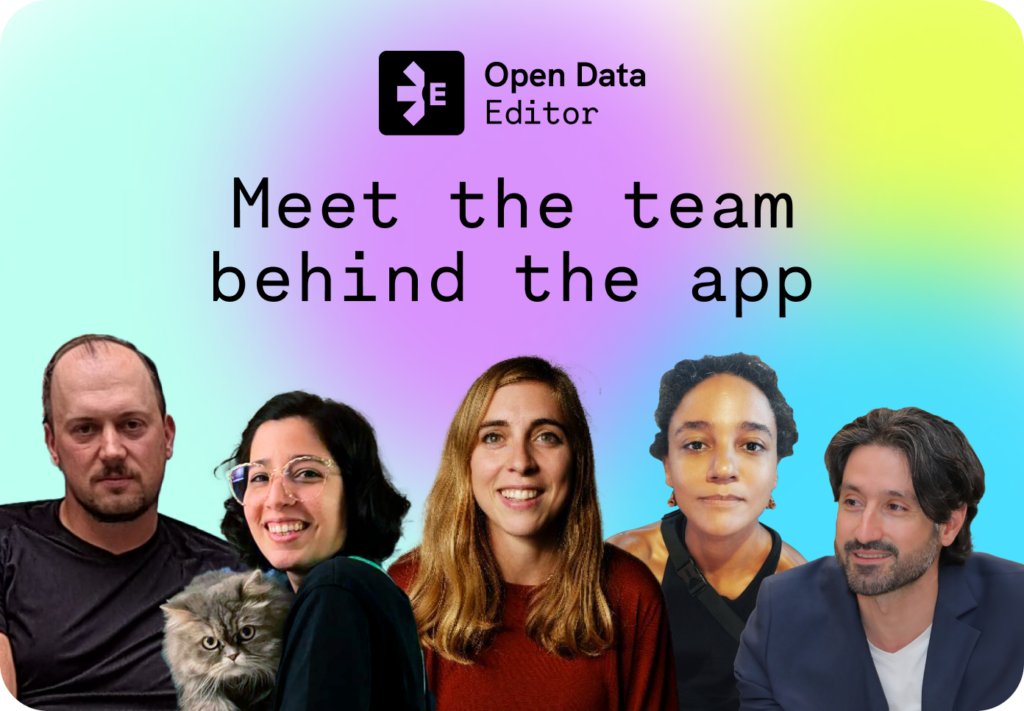
As announced in January, this year the Open Knowledge Foundation (OKFN) team is working to develop a stable version of the Open Data Editor (ODE) application. Thanks to financial support from the Patrick J. McGovern Foundation, we will be able to create a no-code tool for data manipulation and publishing that is accessible to everyone, unlocking the power of data for key groups including scientists, journalists and data activists. (Read more about the Open Data Editor).
Since the beginning of the year, we’ve been busy building the team that will work on developing the app. After meeting and interacting with incredible candidates from all over the world, the team is now formed as follows, including three software developers, a product owner and a project manager. We are happy to announce them to the world today.
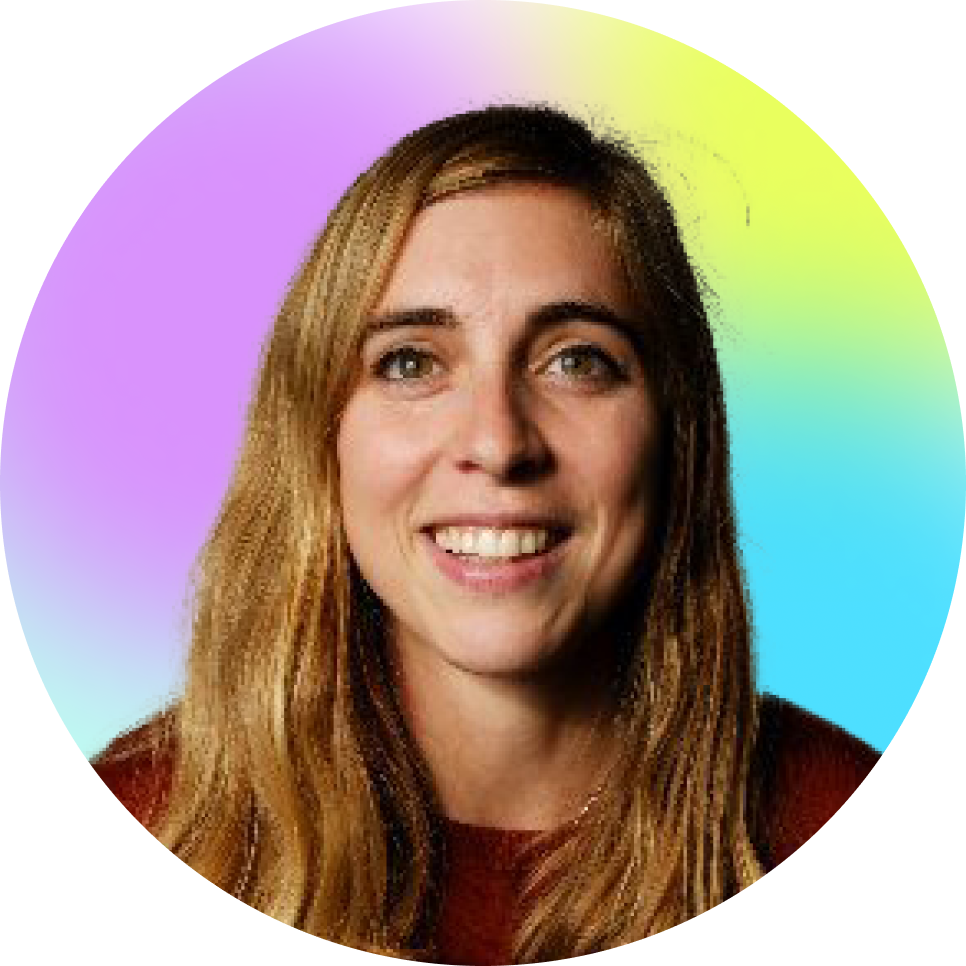
Sara Petti, Project Manager
Sara leads the Open Knowledge Network, which brings together experts of the digital commons globally. The Network’s main focus is the intersection of tech and democracy. At Open Knowledge Foundation Sara also supports open source and open data communities, like the Frictionless Data one, and is passionate about all issues linked to community care and health, like governance. Sara has extensive experience in managing projects. Before joining Open Knowledge Foundation, she was part of a project advocating for public libraries to be on the EU agenda (notably for the review of the Copyright Directive), and was part of the team that developed Khan Academy in French.
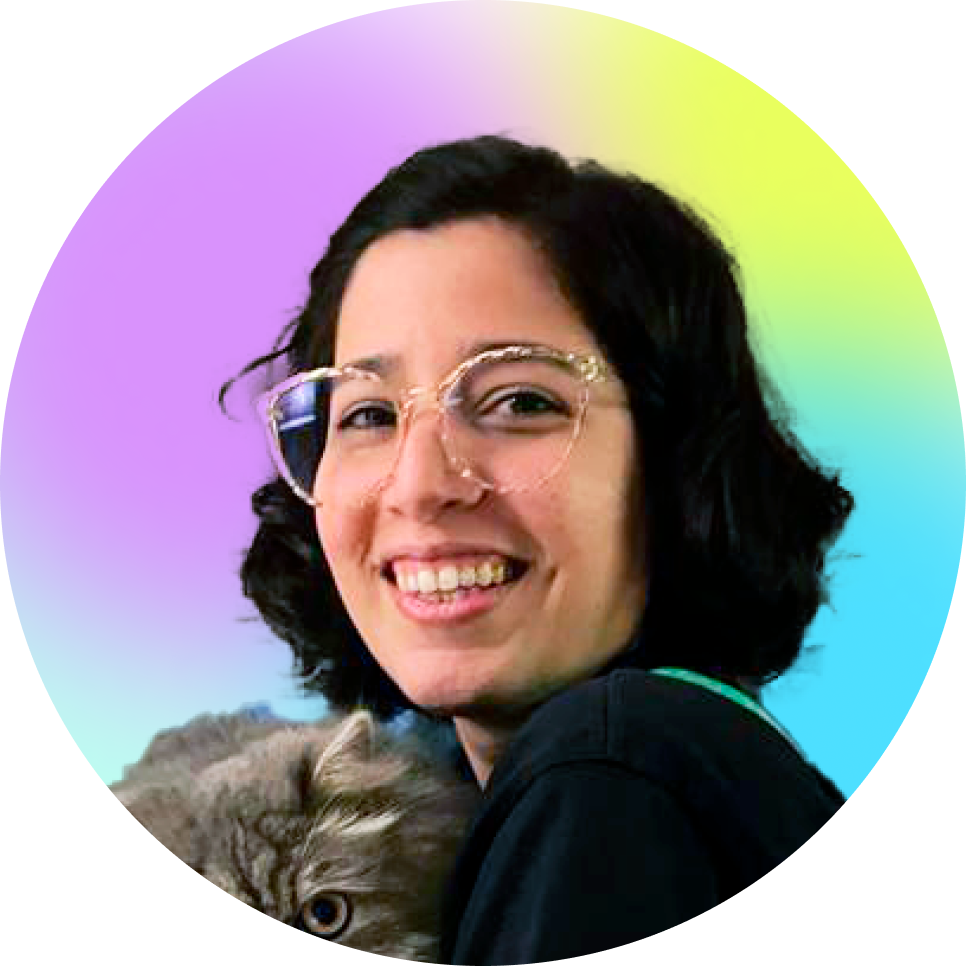
Romina Colman, Product Owner
Romina is a hybrid data journalist working at the intersection of technology and communities of practice. For more than 10 years, Romina has designed and implemented complex data initiatives in close collaboration with journalists and NGOs. Her expertise lies in building bridges between technical and non-technical teams to transform abstract plans into concrete and impactful projects. Romina holds a degree in Communication sciences from the University of Buenos Aires (Argentina) and a master’s degree in Media and Communications (Data and Society) from the London School of Economics and Political Sciences (LSE).
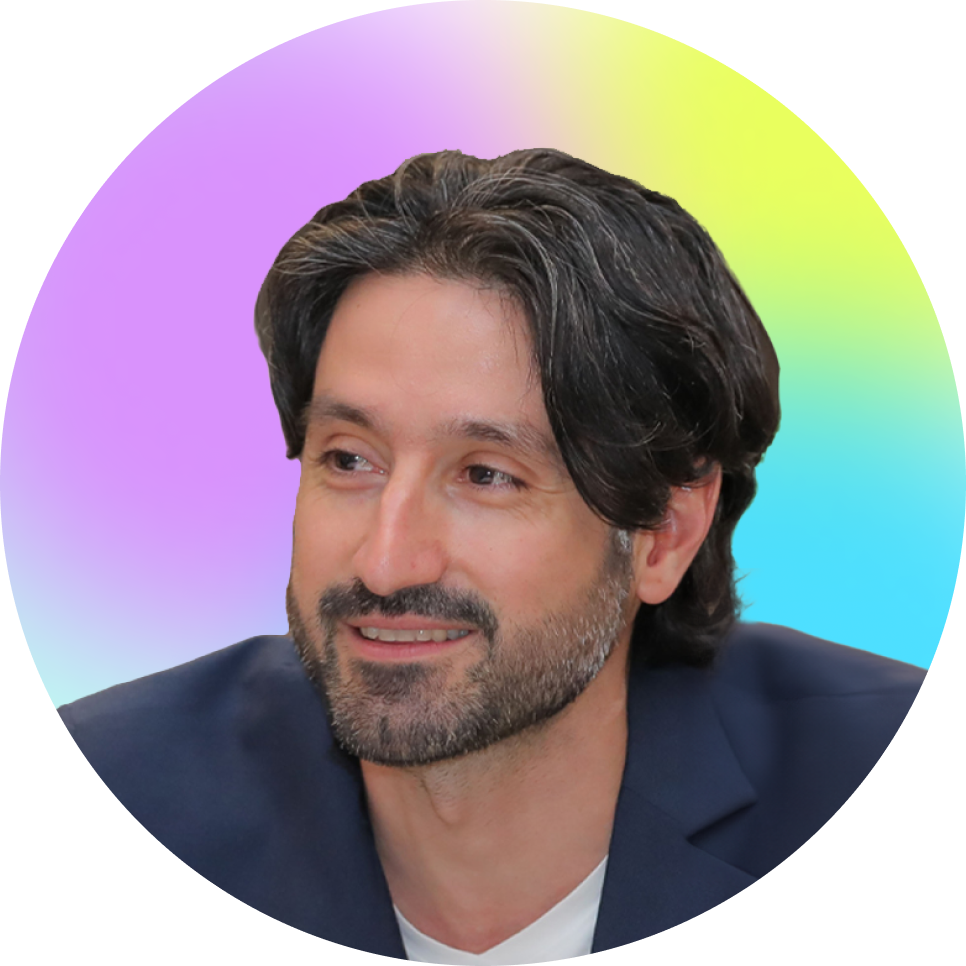
Patricio Del Boca, Tech Lead
Patricio is an Information Systems Engineer with more than 10 years of experience both in the private sector and NGOs. He has also been an activist of the open movement since a young age. He likes to collaborate with different communities to disseminate technical knowledge and participate as a speaker in events to spread the importance of more simple technologies. He loves programming and is always exploring new projects and tools.
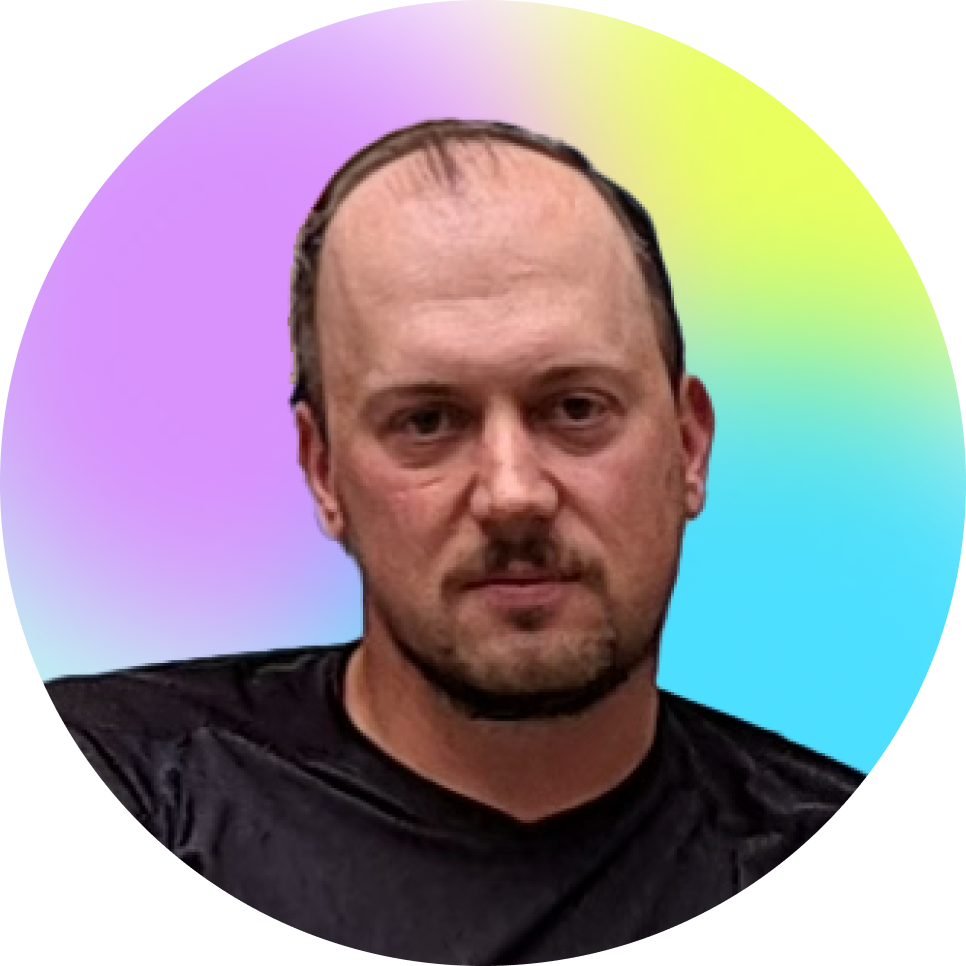
Evgeny Karev, Senior Software Developer
Evgeny is a passionate open-source developer living in Portugal. During his tenure at OKFN, he has led the Frictionless Data project, and designed and partially authored a complete programming stack from low-level data reading to high-level end-user applications like Open Data Editor and services like Frictionless Framework and Livemark. Evgeny participated in many open data empowering projects like CKAN, OpenTrials, and OpenSpending as a Python/JavaScript programmer.
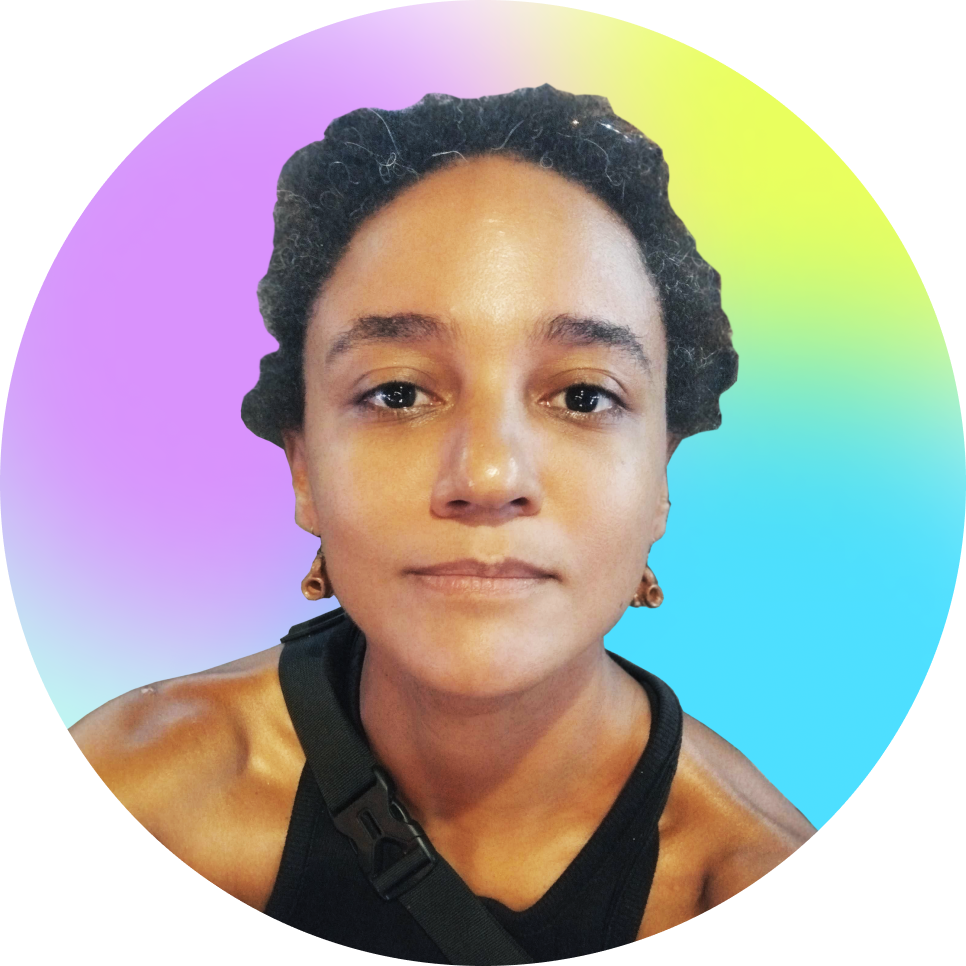
Guergana Tzatchkova, Senior Software Developer
Guergana is a software developer working mainly on free and open source software, educational tools and non-profit organisations. With a Bachelor’s degree in Computer Science, her interest in the creative use of media and technology led her to a Master’s degree in Design of Multimedia and Interactive Systems and later a PhD in Theory and History of Cinema in Barcelona. For several years she has been working on projects that combine audio, video, design and programming. She has also been involved in NGOs working on gender issues in Mexico.
If you want to get more closely involved with the development of the Open Data Editor application, you can express your interest in joining one of the testing sessions by filling this form.
You can also email us at info@okfn.org, follow the GitHub repository or join the Frictionless Data community. We meet once a month.
Read more
Copyright: Best Practices for Academic Libraries / Journal of Web Librarianship
The Republic of the Congo opts for the use of biometrics in multi-party elections / Open Knowledge Foundation
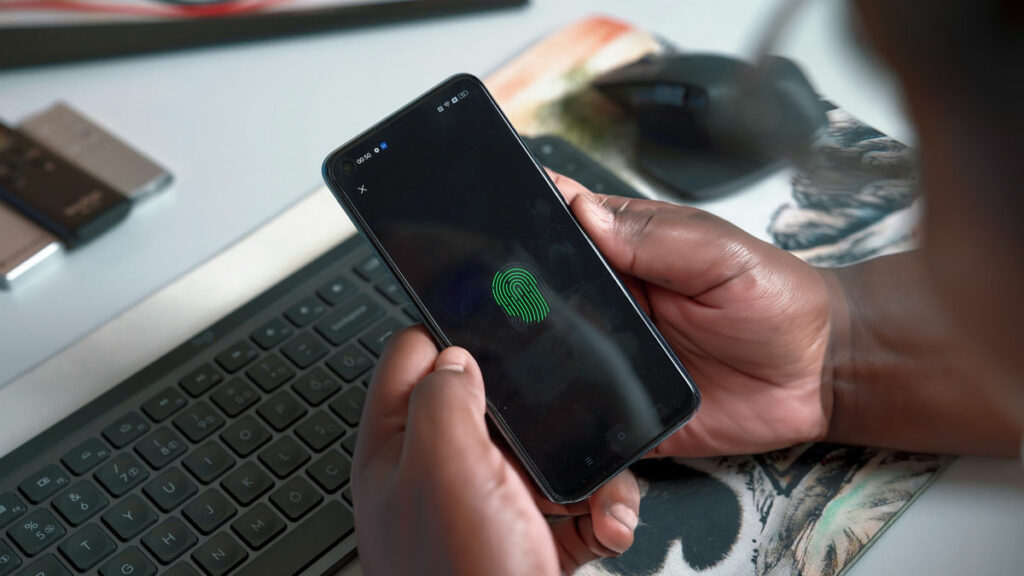
The announcement was made last July by the Prime Minister, Anatole Collinet MAKOSSO. On Tuesday 12 March 2024, the Minister of the Interior and Decentralisation, who is in charge of elections, convened a meeting of election stakeholders, political parties and associations, and representatives of civil society organisations involved in multiparty elections, to officially inform them of the announcement and request their involvement in the process of implementing biometrics for the elections on the horizon: the presidential elections in 2026 and the legislative elections in 2027.
To this end, a political dialogue will be organised to determine the contours and content of the system, which will be used to revise the electoral law, train electoral officials and raise voter awareness.
Biometrics has often been called for by the Congolese opposition, which has always contested the results of previous elections.
In fact, it has become customary for the Congolese government to organise a political dialogue on the eve of each multi-party election to guarantee the organisation and peaceful conduct of the forthcoming electoral process. On this occasion, political parties and associations, as well as civil society organisations, propose solutions for inclusive and peaceful multiparty elections.
It was during the Owando dialogue, organised in 2022, that the use of biometrics in elections was recommended by the participants.
Electoral processes in the Republic of the Congo (Brazzaville), from the first experience of multiparty pluralist elections in 1959, resumed in 1992 after a period of single-party rule, to the present day, have been a source of armed conflict and internal civil war. The bloodiest of these were in 1997, 1998, 2015 and 2016, and several opposition candidates are still in prison.
The use of biometrics in the Republic of the Congo (Brazzaville) could serve as a remedy for the ills that undermine the electoral process, including multiple voting, ballot box stuffing, the inflating of the electorate, etc.
The political will to use biometrics as a means of peacefully resolving political disputes and strengthening the credibility of multi-party elections in the Republic of the Congo (Brazzaville) should be seized as an opportunity for the international community and national and international civil society to propose actions to support the implementation of biometrics in multi-party democratic elections in the Republic of the Congo (Brazzaville).
Advancing IDEAs: Inclusion, Diversity, Equity, Accessibility, 16 April 2024 / HangingTogether
The following post is one in a regular series on issues of Inclusion, Diversity, Equity, and Accessibility, compiled by a team of OCLC contributors.

Reclassifying for religious equity
The 2024 Public Library Association (PLA) Conference featured the program “Increase Religious Equity by Reclassifying Dewey 200’s,” which described how two public libraries implemented the optional arrangement of the Dewey Decimal Classification (DDC) 200 Religion Class. OCLC’s DDC Senior Editor Alex Kyrios explained that the standard arrangement uses the bulk of the 200s for Christianity topics (230-280), leaving the span 290-299 for other religions. The result is books about Islam, Judaism, Buddhism, and other major religions are often shelved very close together with long numbers that do not fit on book spines. For example, Islam, the second largest religion by population, is classed in 297 in the standard arrangement while the optional arrangement uses 281-298. Librarian Emily McDonald from Lawrence Public Library (OCLC Symbol: KSA) in Lawrence, Kansas, described how her library implemented the optional arrangement and created a 220-299 Project Packet to help other libraries considering switching to the optional arrangement. Elizabeth McKinstry and Matthew Jaquith, two librarians at Springfield City Library (OCLC Symbol: WRS) in Springfield, Massachusetts, were inspired by a Tweet from the Lawrence Public Library to implement the optional arrangement in their library system.
This program was inspiring to me in multiple ways. It demonstrates how our profession improves cataloging practices in response to patrons’ needs. It is also a wonderful example of how librarians help each other to provide better service to users. The Lawrence Public Library’s project packet helped Springfield to complete its reclassification project, and the Springfield librarians have provided outreach to other New England public libraries about how they might implement the optional arrangement. Contributed by Kate James.
Book censorship in academic, public, and school libraries
On March 28, 2024, Ithaka S+R, the not-for-profit dedicated to “helping the academic community use digital technologies to preserve the scholarly record and to advance research and teaching in sustainable ways,” issued its research report on “Censorship and Academic Freedom in the Public University Library,” by Senior Analyst Ess Pokornowski and Vice President Roger C. Schonfeld. Interviewing five library leaders from U.S. states with restrictive DEI and gender-issue policies and five from states where such laws have been “tabled or defeated,” they found strong agreement on the goal of defending academic freedom within the context of their institutions of higher education. ALA’s American Libraries Direct for 3 April 2024, appropriately brought the Ithaka S+R report together with Kelly Jensen’s Book Riot roundup of “How Public Libraries Are Targeted Right Now—It’s Not ‘Just’ Books: Book Censorship News, March 29, 2024.” Former librarian Jensen particularly notes how censorship in school libraries tends to differ from that in public libraries.
Both the Pokornowski-Schonfeld research and the Jensen report contain reasons for optimism, but strongly argue against any complacency on the part of library communities. Because they are superficially similar types of organizations operating within dissimilar institutional and political contexts, school libraries, public libraries, and public university libraries face some different challenges, although there are certainly common threads. Perhaps the most obvious and ominous thread is that the challenges are not going away any time soon. Contributed by Jay Weitz.
Arlington Public Library’s Passport Program receives 2024 City Cultural Diversity Award
The City of Arlington, Texas has been honored with the 2024 Cultural Diversity Award for Arlington Public Library’s (OCLC Symbol: AR9) Passport Scholarship program. Presented by the National League of Cities, this award recognizes leadership in developing creative and effective programs that demonstrate quality and innovation in cultural diversity. The Passport Scholarship program was designed to help students in the Arlington community obtain their first passport, eliminating the initial hurdle for those eager to explore other cultures. The program is funded by the Otis and Rosie Brown Foundation and was launched in 2022 to align with the library’s goal of supporting learning and new experiences for residents. “By enriching their own lives, they will ultimately influence, shape, and edify the community they live in.”
As demand for U.S. passports remains high, and in times of reduced funding, many libraries are capitalizing on this need by serving as an official Passport Acceptance Facility (PAF) for the U.S. Department of State. American Libraries shares one library’s experience on running a passport acceptance facility, and the U.S. Department of State provides step by step instructions on becoming a Passport Acceptance Facility. Contributed by Jennifer Peterson.
Reparative Archival Description: The Past, Present, and Future
Yale University’s Reparative Archival Description (RAD) Working Group is hosting an 18 April virtual panel that is focused on reparative archival description, and how practices have evolved over the last five years. Speakers will focus on challenges and opportunities and are from a range of institutions, including Bentley Historical Library at the University of Michigan (OCLC Symbol: BEU), University of North Carolina (OCLC Symbol: NOC), Algoma University (OCLC Symbol: CNALU), UC Berkeley (OCLC Symbol: CUY), and Yale University (OCLC Symbol: YUS).
Because of the nature of archival description, (typically more voluminous and prose based than bibliographic description) there are special challenges inherent in identifying and remediating language used in finding aids and other modes of archival description, especially when those descriptive forms may have been written decades ago. In hosting online sessions like this, Yale’s RAD Working group is helping to contribute to a broad community of practice that continues to grow. Contributed by Merrilee Proffitt.
The post Advancing IDEAs: Inclusion, Diversity, Equity, Accessibility, 16 April 2024 appeared first on Hanging Together.
Elon Musk: Threat or Menace Part 4 / David Rosenthal
It seems the driver thought that it was OK to drive home with a blood alcohol level of 0.26 because he believed Musk's hype that Fake Self Driving would handle it despite having to repeatedly override it on the way out.Now, the team's Faiz Siddiqui and Trisha Thadani are out with In 2018 crash, Tesla’s Autopilot just followed the lane lines. Below the fold I look into what it reveals about Autopilot.
![]() |
Source |
The case involves a fatal crash in March 2018, when a Tesla in Autopilot careened into a highway barrier near Mountain View, Calif., after getting confused by what the company’s lawyers described in court documents as a “faded and nearly obliterated” lane line.Musk's and Tesla's marketing hype conflict with the deposition:
The driver, Walter Huang, 38, was killed. An investigation by the National Transportation Safety Board later cited Tesla’s failure to limit the use of Autopilot in such conditions as a contributing factor: The company has acknowledged to National Transportation Safety Board that Autopilot is designed for areas with “clear lane markings.”
Under oath, however, Tesla engineer Akshay Phatak last year described the software as fairly basic in at least one respect: the way it steers on its own.
“If there are clearly marked lane lines, the system will follow the lane lines,” Phatak said under questioning in July 2023. Tesla’s groundbreaking system, he said, was simply “designed” to follow painted lane lines.
...
In his deposition, Phatak said Autopilot will work wherever the car’s cameras detect lines on the road: “As long as there are painted lane lines, the system will follow them,” he said.
![]() |
Source |
Huang, an engineer at Apple, bought his Tesla Model X in fall 2017 and drove it regularly to work along U.S. Highway 101, a crowded multilane freeway that connects San Francisco to the tech hubs of Silicon Valley. On the day of the crash, his car began to drift as a lane line faded. It then picked up a clearer line to the left — putting the car between lanes and on a direct trajectory for a safety barrier separating the highway from an exit onto State Route 85.It has been evident for a long time that just following the lines doesn't live up to the hype:
Huang’s car hit the barrier at 71 mph, pulverizing its front end, twisting it into unrecognizable heap. Huang was pronounced dead hours later, according to court documents.
...
In the months preceding the crash, Huang’s vehicle swerved in a similar location eleven times, according to internal Tesla data discussed by Huang’s lawyers during a court hearing last month. According to the data, the car corrected itself seven times. Four other times, it required Huang’s intervention. Huang was allegedly playing a game on his phone when the crash occurred.
For years, Tesla and federal regulators have been aware of problems with Autopilot following lane lines, including cars being guided in the wrong direction of travel and placed in the path of cross-traffic — with sometimes fatal results. Unlike vehicles that are designed to be completely autonomous, like cars from Waymo or Cruise, Teslas do not currently use sensors such as radar or lidar to detect obstacles. Instead, Teslas rely on cameras.As usual, Tesla's response to the crash was to do as little as possible:
After the crash that killed Huang, Tesla told officials that it updated its software to better recognize “poor and faded” lane markings and to audibly alert drivers when vehicles might lose track of a fading lane. The updates stopped short of forcing the feature to disengage on its own in those situations, however. About two years after Huang died, federal investigators said they could not determine whether those updates would have been sufficient to “accurately and consistently detect unusual or worn lane markings” and therefore prevent Huang’s crash.The most important thing for Tesla is never to remind the driver of the limitations of their software because doing so would exacerbate the fall in the stock price, currently down 57% from its peak. As I wrote in Autonomous Vehicles: Trough of Disillusionment:
Elon Musk famously claimed that Tesla is worth zero without Full Self Driving. But although this is typical Musk BS, ... unlike some other utterances it contains a kernel of truth. Tesla is valued as a technology company not a car company. Thus it is critical for Telsa that its technology be viewed as better than those of other car companies; anything that suggests it is limited or inadequate is a big problem not just for the company but also for Musk's personal wealth.Liam Denning describes the problem for Musk if doubts emerge about the AIs driving Teslas:
Tesla is, overwhelmingly, a maker of electric vehicles, combining high growth with high margins — until recently anyway. Deliveries increased by 38% in 2023 — below the company’s long-term target of 50% per year — and the consensus for 2024 implies just 21%. Trailing 12-month net profit as of the third-quarter was actually down, year over year.In The Biggest AI Hype Fraud of All Time Michael Spencer writes:
Yet in the most starry-eyed Wall Street financial models, the making and selling of vehicles — generating 92% of Tesla’s current gross profit — accounts for only a fraction of Tesla’s purported valuation. The rest relates to whatever Tesla’s next big thing might turn out to be, usually something related to artificial intelligence, be it robotaxis, licensed self-driving systems, the Optimus humanoid robot or just something else that might spring from the company’s Dojo supercomputing project.
Amorphous as the narrative may be, remove it and the tenuous tether between Tesla’s valuation and something approximating a potential future reality evaporates entirely.
Tesla's FSD costs have tripled since 2019, costing more than $15,000 in the United States. This pumped up, fraudulently, Tesla’s margins on selling vehicles, however Elon Musk’s promises did not come to fruition after many deadlines have passed.Spencer notes that "desperation at Tesla is very noticeable in 2024":
In a push for end-of-quarter sales, Musk recently mandated that all sales and service staff install and demo FSD for customers before handing over the keys.He focuses on Musk's pivot to x.AI:
...
In a recent April 5th Tweet on X, Elon Musk says full level 5 FSD is coming in August, 2024. Tesla’s stock so far in 2024 is down 33%.
The myth that Tesla is a technology or AI company has been very crucial in the false promise marketing around the brand. Elon Musk’s weird response to this failure in 2024 is to poach AI talent from his Tesla to his own x.AI company.Maintaining the illusion of superior technology requires leaps of logic:
This is because x.AI plans to do a huge $3 Billion funding round that would value the AI startup at $18 Billion. This is all more or less breaking news.
The problem is AI frauds have a habit of big declines. Elon Musk may have to make his SpaceX company, valued at around $180 billion as of early 2024, go public with an IPO to raise the funds needed to support his X Corp empire.
Since 2017, officials with NTSB have urged Tesla to limit Autopilot use to highways without cross traffic, the areas for which the company’s user manuals specify Autopilot is intended. Asked by an attorney for Huang’s family if Tesla “has decided it’s not going to do anything” on that recommendation, Phatak argued that Tesla was already following the NTSB’s guidance by limiting Autopilot use to roads that have lane lines.Note how, in Tesla's world, any "roads that have lane lines" are "highways without cross traffic", and that Tesla is not limiting Autopilot's use but asking their customers to limit its use. A significant difference. And Musk's reality distortion field is in full effect:
When asked whether Autopilot would use GPS or other mapping systems to ensure a road was suitable for the technology, Phatak said it would not. “It’s not map based,” he said — an answer that diverged from Musk’s statement in a 2016 conference call with reporters that Tesla could turn to GPS as a backup “when the road markings may disappear.” In an audio recording of the call cited by Huang family attorneys, Musk said the cars could rely on satellite navigation “for a few seconds” while searching for lane lines.This casual attitude to operating in the real world is typical of Tesla:
Phatak’s testimony also shed light on other driver-assist design choices, such as Tesla’s decision to monitor driver attention through sensors that gauge pressure on the steering wheel. Asked repeatedly by the Huang family’s lawyer what tests or studies Tesla performed to ensure the effectiveness of this method, Phatak said it simply tested it with employees.Given Musk's notorious hair-trigger firings in response to disagreement, testing with employees is pretty much guaranteed to discover that the system performs almost perfectly.
The Washington Post team points out that this poor engineering of life-critical systems has real-world impacts:
Tesla’s heavy reliance on lane lines reflects the broader lack of redundancy within its systems when compared to rivals. The Post has previously reported that Tesla’s decision to omit radar from newer models, at Musk’s behest, culminated in an uptick in crashes.Whereas other companies behave responsibly:
Other Tesla design decisions have differed from competitors pursuing autonomous vehicles. For one thing, Tesla sells its systems to consumers, while other companies tend to deploy their own fleets as taxis. It also employs a unique, camera-based system and places fewer limits on where the software can be engaged. For example, a spokesperson for Waymo, the Alphabet-owned self-driving car company, said its vehicles operate only in areas that have been rigorously mapped and where the cars have been tested in conditions including fog and rain, a process known as “geo-fencing.”So that's all there is to Autopilot. No radar, no lidar, no GPS, no map, no geofencing, no proper driver monitoring. It just uses the camera to follow the lines. It doesn't disengage if it can't see the lines, it just keeps going. So much for Tesla's vaunted AI capabilities! I wonder how much more you get for the $15K extra you pay for Fake Self Driving?
“We’ve designed our system knowing that lanes and their markings can change, be temporarily occluded, move, and sometimes, disappear completely,” Waymo spokeswoman Katherine Barna said.
#ODDStories 2024 @ Kibaale, Uganda 🇺🇬 / Open Knowledge Foundation
On 8th March 2024, which also doubled as International Women’s Day, Rural Aid Foundation held a community Open Data Day event titled “Empowering migrant and refugee women to use open data to hold duty bearers accountable for quality sexual reproductive health services“. This event was directly linked to the advancement of SDG 3: Good health and well-being. The event took place in Nyamarunda sub-county, Kibaale district, Uganda and it was aimed at exploring the use of open Sexual Reproductive Health data (readily available and freely accessible) from public health facilities as a tool to hold duty bearers such as humanitarian agencies, refugee leaders and health service providers accountable to deliver quality Sexual reproductive health service for refugee and migrant women in Kibaale.
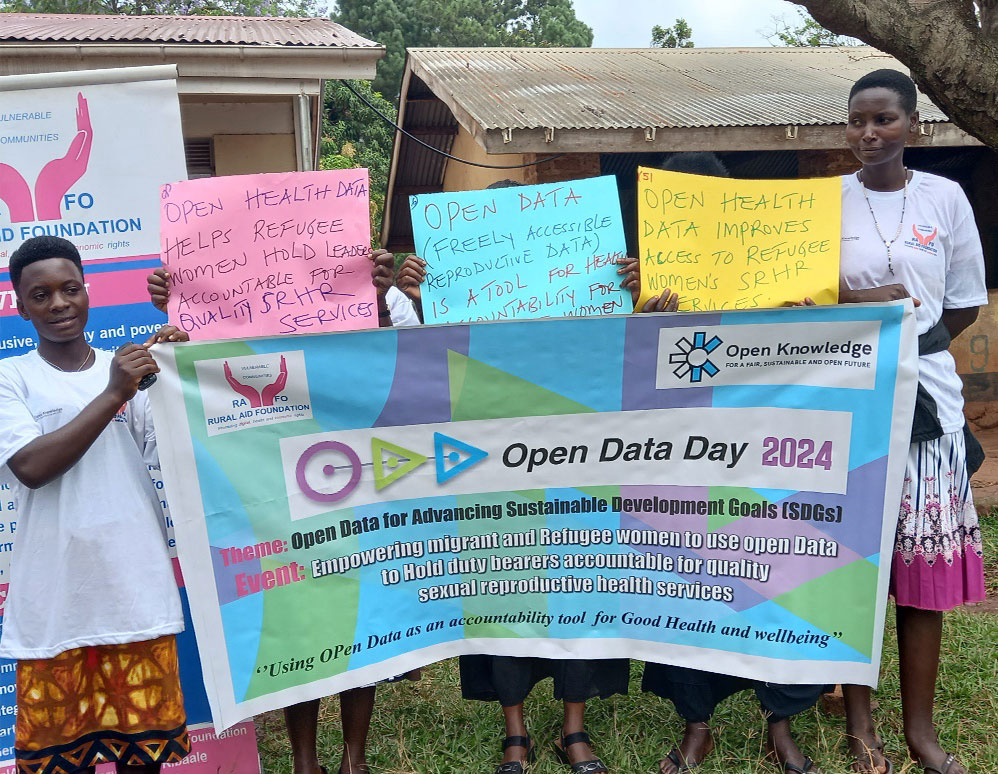
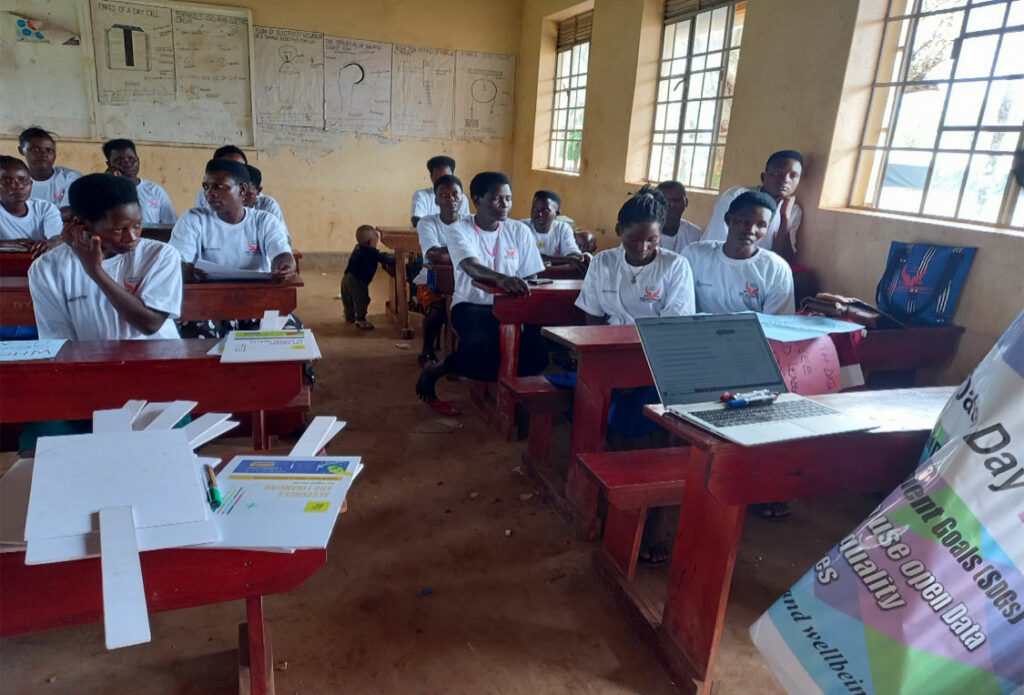
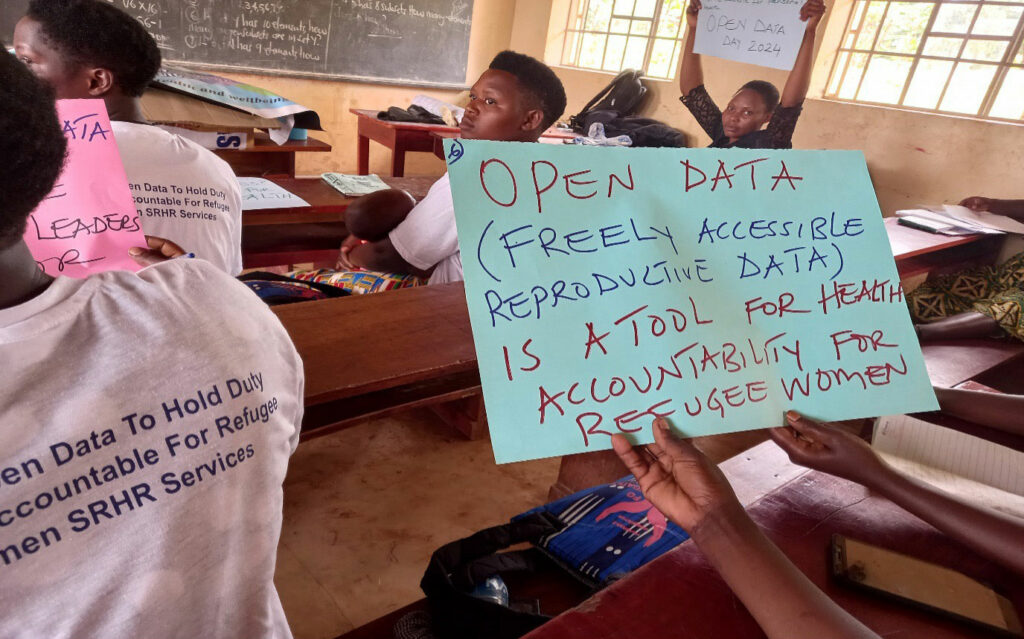
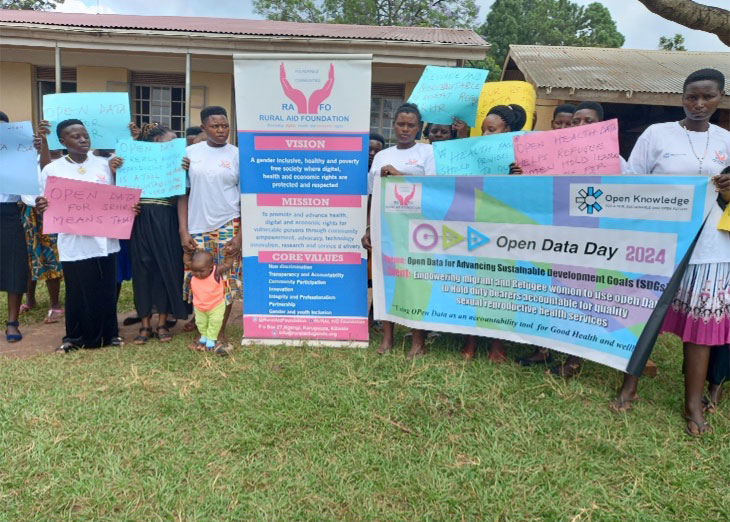
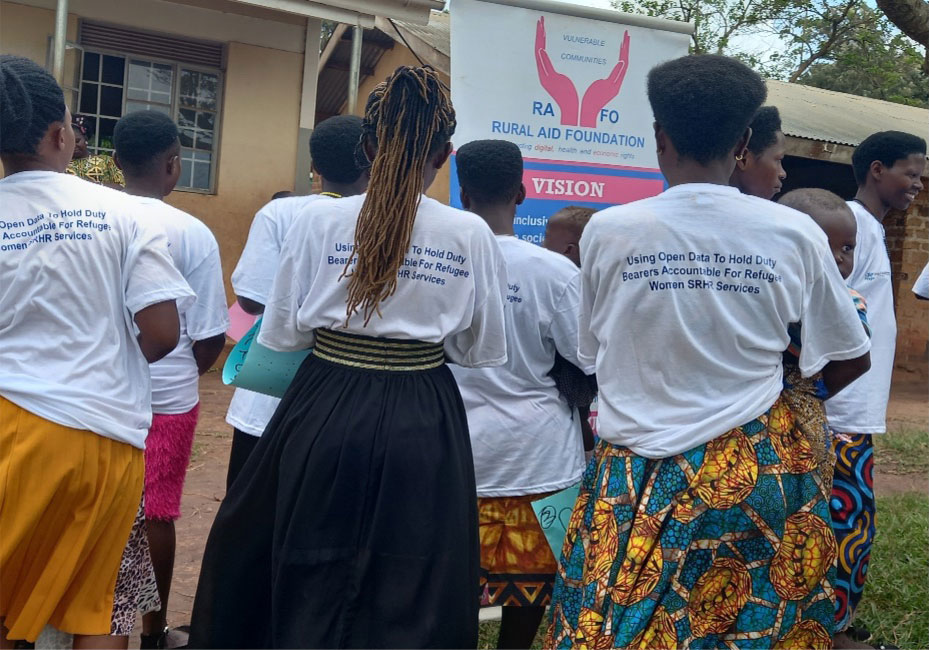
Activity 1: Mobilized and oriented a pool of 60 rural migrant refugee women and girls and 10 women-led community organizations on the concept of open data and how to use open data to hold duty bearers accountable for providing quality SRHR services
Rural Aid Foundation mobilized a pool of 60 rural migrant refugee women and girls and women-led community organizations and oriented them on the concept of open data and how to use open data as a tool to hold duty bearers accountable in providing quality SRHR services for refugees and migrants. The orientation that was conducted by Ms Scovia Mbabazi covered a basic introduction to open data and the dimensions of open data in the context of Sexual Reproductive Health and Rights (SRHR) including;
- Availability and access: That data must be available and accessible as a whole or at a reasonable or affordable cost. In the context of sexual reproductive health data, this means that sexual reproductive health data should be available to refugees and anyone who needs it to improve the quality of health of refugees. Health facilities in refugee settings should ensure the availability and accessibility to data related to maternal and newborn health, contraception, safe abortion and post-abortion care, and Gender-based violence. The data should be available to anyone who needs it in a convenient and modifiable form.
- Re-use and Redistribution: The SRHR data available in the health facilities in the refugee settlement or emergency settings must allow re-use, redistribution, and intermixing with other data sets to enable duty bearers to make decisions to address issues related to the quality of SRHR services provided.
- Universal participation: The data should be available for use, re-use and redistribution across a range of fields and stakeholders, including refugees, migrants and duty bearers such as health service providers to improve the quality of services.
The facilitator further discussed eight open government data principles developed in 2007 by 30 open government advocates and these were discussed in the context of SRHR for refugees noting that public SRHR data shall be considered open if it is made public in a way that complies with the following eight principles:
- Complete: All public SRHR is made available and is not subject to valid privacy, security or privilege limitations.
- Primary: Data is as collected at the source such as health facility registers and health management systems with the highest level of quality and not modified in any form.
- Timely: Data should be provided as quickly as possible when it is needed to enable duty-bearers to use it for decision-making.
- Accessible: Data is available to a range of stakeholders for a range of different purposes.
- Machine processable: Data should be easily processed and used in any form as required by the user.
- Non-discriminatory: Data is available to anyone, with no requirement of authentication or registration required.
- Non-proprietary: Data should be available to everyone without anyone claiming control over the data. It should be publicly available health data. Data is available in a format over which no entity has exclusive control.
- License-free: Data should not be subject to any copyright patent or trade secret regulation. However, reasonable privacy and security to protect the rights of patients.
Due to the sensitive nature of SRHR data, participants recognized some ethical issues that must be upheld to ensure the rights of refugees and migrants are upheld in the use of open data.
‘’Some SRHR data such as HIV test results, post abortion data, cervical cancer test results cannot be open to everyone to access especially if it includes personal information such as the name of the patients. It is important to note that open data usage in refugee settings to hold duty bearers accountable must ensure the rights of refugee patients are protected’’
Health facility in charge, Bjubuli Health Center
To ensure these ethical and human rights issues are understood by all the participants, the facilitator discussed privacy, confidentiality and informed consent issues in accessing open health data.
- Privacy: This refers to the fact that all SRHR data to be accessed, reused and shared must ensure the privacy of the ‘’data owners’’ – the patients and no personal information such as names should be shared along with open data. Health facilities should not provide SRHR data for refugees with personal identifying information such as names.
- Confidentiality: Any health data such as HIV status data should not be put in the public domain provided it holds or contains personal identifying data such as names of a patient.
- Informed consent: Health service providers should always ensure the ‘’data owners’’ are notified why their data is being shared with anyone and the data should be availed for reasons that help to benefit and improve refugee health outcomes or to improve the quality of SRHR services.
Activity 2: Worked with migrant refugee women/girls and organizations to identify and document Sexual Reproductive Health and Rights service quality gaps/issues using publicly accessible data from Kibaale community health facility
Rural Aid Foundation worked with migrant refugee women/girls and organizations to document Sexual Reproductive Health and Rights service quality issues such as lack of SRHR commodities such as condoms, lack of cervical cancer screening services, lack of adequate family planning services using publicly accessible data the community health facility information registers. These were presented to duty bearers for corrective action to be taken.
Activity 3: Conducted a flash mob to create awareness on awareness on open data issues and their implications Good health and wellbeing (SDG3)
Rural Aid Foundation worked with migrant women and girls to hold flash mobs in Kibaale town to create community awareness on open data issues and their implications on achieving SDG 3. Rural women ambassadors held a match and flashmob to further create community awareness of open data as a tool to hold duty bearers accountable for quality SRHR services for refugees to achieve Universal Health Coverage (SDG 3). During the flash mobs, the migrant women and refugees displayed messages calling upon duty bearers to ensure access to data at health facilities as a tool for migrant women and refugees to demand quality SRHR services.
Activity 4: Hold a peaceful match at Kibaale district headquarters to present the open data issues identified by the Kibaale community health facility to the district health officer and other district leaders to seek corrective action.
The migrant women were matched to Kibaale Health Center IV, where they presented issues discussed under Activity 2 to duty bearers for corrective action to be taken. The duty bearers through the district biostatistician committed to ensuring all health facility health data clerks extract and publish sexual reproductive health services and delivery data from the health management information system and avail it to community members via the community note boards.
Conclusion
Open data can be used by community members to hold service providers accountable for improving the quality of sexual reproductive health services in refugee settings.
About Open Data Day
Open Data Day (ODD) is an annual celebration of open data all over the world. Groups from many countries create local events on the day where they will use open data in their communities.
As a way to increase the representation of different cultures, since 2023 we offer the opportunity for organisations to host an Open Data Day event on the best date within a one-week period. In 2024, a total of 287 events happened all over the world between March 2nd-8th, in 60+ countries using 15 different languages.
All outputs are open for everyone to use and re-use.
In 2024, Open Data Day was also a part of the HOT OpenSummit ’23-24 initiative, a creative programme of global event collaborations that leverages experience, passion and connection to drive strong networks and collective action across the humanitarian open mapping movement
For more information, you can reach out to the Open Knowledge Foundation team by emailing opendataday@okfn.org. You can also join the Open Data Day Google Group to ask for advice or share tips and get connected with others.
the not knowing: cage and calvinism / María A. Matienzo
it’s been a while since i’ve been deeply unsettled by the lack of resolution in a film, especially if the film’s conceit is overall preposterous. however, having just experienced the disquieting jouissance of such cinematic bombast last night, here i am, with a need to verbalize and process this tormentand whom else would i have to thank for this but my favorite member of the coppola family, nicolas cage, rumplestiltskin of the dramatic arts that he is. what, then, of the film that originated this long-winded introduction of this disquiet from theological and epistemological perspectives? it would be none other than KNOWING (2009, dir. alex proyas). spoilers follow, so be forewarned, lest ye find not your salvation.
i will not go into the plot in depth, but rather obliquely and nonlinearly. as such, the remainder of my writing assumes familiarity with the movie, and i’ll say up front that i’m providing an unalloyed recommendation. if i were to sum it up, however, its major thematic aspects relate to knowledge, faith, other-worldly forces, and the epistemic uncertainty that undergirds all of them. i’m struck by the movie’s refusal to take a clear stance on its major plot points, and thus places responsibility on the viewer to bring its own interpretation to bear. even in moments of it being at its most clear-cut — namely, the penultimate scene of ἀποκάλυψις, a razing of new york city by fire caused by climate change “solar flares” (i.e. “the wrath of god [that] burns against them” a la jonathan edwards) — an engaged viewer will most notably exclaim “what the actual fuck?” despite this ambiguity, this film is masterfully unsubtle, teeming with intertextual references to christian eschatology across multiple denominations and media, an embarassing use of skepticism as a kind of morality strawman-cum-punching bag, and extremely intense depictions of plausible(!) real-world disasters with mildly sickening CGI.
in terms of its focus on free will, KNOWING initially opens with the conceit of nicolas cage as john koestler, an MIT astrophysicist holding court in an undergrad class opposing free will with some sort of in-between hybrid of nomological determinism and predeterminism. it is here that john, says that he thinks “shit just happens,” and soon after we discover that he’s an atheist academic raised as preacher’s kid that had his latest crisis of faith after his wife died in a horrible hotel fire just days before his birthday. as he becomes obsessed with decoding and identifies the “real life” past and impending catastrophes, we see him bias towards predeterminism, but the as the truth itself is slowly revealed we are supposed to infer that every known cataclysm is delineated as a warning that something is coming for EE — everyone else. (it’s giving “this place is a message and is part of a system of messages; pay attention to it.” real “pick me” vibes.) as john dives into to try to stop or save people from terrible things happening (literally sticking his hands in flames to no avail in a failed attempt to save a plane crash victim), he is reminded and humbled by the great futility of his own existence, and his powerlessness in a cruel universe. why are all these things happening? and why do we know the exact predicted death toll?
as we start to realize this, it’s here that i see that the film begins shifting from predeterminism to predestination, and that perhaps, someone in the film is a messenger who will receive this message from the far beyond. it’s clear that the movie’s precocious child characters – john’s son, caleb, and abby, the granddaughter of lucinda, the girl who wrote the numbers that went into the time capsule – are the recipients of the gift of prophecy. but surprise: they’re also special in that they are the elect, bound to bearing the life of the world to come and imminently transported away by these celestial beings. and yet, are they angels? are they aliens? are they both? where does that leave poor old john? fucked in the end: he is not one of the elect. faced with his own spiritual damnation and physical annihilation, he returns to his ancestral home to be with his mildly estranged parents and heavily queer-coded nurse sister.
what’s fascinating to me about this movie is that it refuses to come out and really say what it’s about, and here’s where i disagree with roger ebert.1 we are supposed to be unsure whether they’re angels or aliens because their depiction is ambiguous. what fascinates me is that the lead writer, ryne pearson, also deliberately plays at that ambiguity.2 just the same, cage also believes it’s up to the viewer what to take from the movie, and expects that it might stimulate discussion.3 4 compare donald barthelme:
this is, i think, the relation of art to world. i suggest that art is always a meditation upon external reality rather than a representation of external reality or a jackleg attempt to “be” external reality.5
pearson is apparently a dedicated Catholic, too.6 these aspects combined make it also all the more fascinating to me that the movie’s themes feel particularly Calvinist: despite our faith and good works, most of us are truly and undeniably bound to suffer. yet as john says goodbye to caleb, and both as foreshadowed by john’s phone conversation to tell his father that the end is nigh and in the koestler family barbecue incineration and damnation, there is a presumption of being ready for that next life and being sure that you’ll be reunited in the world to come based on faith7 – which in some senses is a not-knowing.
however, a good Calvinist epistemologist (yes, i’m side-eying Plantinga) might not say this, and may well lead us down a path of something like the presuppositional apologetics of cornelius van til. in these cases, the world of KNOWING seems to suggest that we need to accept that world’s God that makes it possible for an atheist like john to be so rationally minded in the beginning of the movie. john operates in the discursive frame of science and the academy and thus has to perform rationality to be credible. caleb is disappointed when he realizes (early in the movie) that john doesn’t believe in heaven. despite thinking that “shit just happens” and that “we can’t know for sure” (i.e., that heaven exists), at some point in the past john has accepted a presuppositional mindset, which he slowly regains as he sees the truths in the messages. he specifically notes that he lost a form of faith in knowing what was coming while in the throes of grief, which in turn led him to be more nomologically oriented. however, the list of numbers was an intervention that led him to reconsider his loss of faith, because despite how unlikely it might be to an extremely rational astrophysicist, he was called back to accept the presuppositions that inform all of his underlying complexities.
again, we need to remember this was most likely not intentionally a Calvinist apocalypse film. the statements of pearson and cage don’t jive with that. if anything, KNOWING indeed puts the onus on us to observe and dissect the discursive and epistemological frames we look through to square religion and the world. this is perhaps, indeed, why the movie is so baffling - that not even the angel/iens ever describe how or what ever directly to the audience. one cannot simply anticipate what will happen, and that in itself, leads to the revelatory experience of watching this film itself. without prior knowledge, without that grounding, you really have no fucking clue what you’re getting yourself into. with apologies to barthelme, this is the combinatorial agility of knowledge and belief, the exponential generation of meaning, once they’re allowed to go to bed together… 5 — the liaison where we can experience the epistemic jouissance of KNOWING.
https://www.rogerebert.com/roger-ebert/a-roll-of-whose-dice ↩︎
http://bibchr.blogspot.com/2009/03/interview-with-ryne-pearson.html ↩︎
https://www.independent.ie/regionals/herald/cage-without-a-key/27906163.html ↩︎
https://www.patheos.com/blogs/sisterrosemovies/2009/03/knowing-the-movie-an-interview-with-ryne-pearson-screenwriter/ ↩︎
Wrong on the internet / Hugh Rundle
I recently read Tyson Yunkaporta's Right story, wrong story – his latest book after the one that grew a bunch of new synapses in my brain, Sand Talk.
It was a different experience. Perhaps it seemed less revelatory because I'd already read Sand Talk. Perhaps Yunkaporta just had less time to translate these ideas into something mainstream white Australians would understand. Perhaps the endless grind of trying to survive as an Indigenous person in a settler-colonial capitalist state made it harder to write. I'm not complaining – this is a great book and you should read it. But it's hard not to hope that when one reads an author's first book for a general audience and it utterly changed how one sees the world, that the next one will be just as exhilarating. It's hardly his fault that it didn't rewire my brain a second time.
The book covers a lot of ground, but the primary concept is that when telling a story (the best way to convey information) you can tell it in a way that is "right story", or you can tell it in a way that is "wrong story". The underlying message running through this is that how you convey information and tell stories is just as important as the "content" you are conveying.
In many ways this is not particularly New News. Any educator who has undergone any training in the last 30 years will know about "constructivism" and that the idea of education as simply passing knowledge to learners like one fills a jug with water is a completely inaccurate description of how learning actually works. But I think there are some additional, more subtle things here. It's not just that "active learning" is useful. How we explain things, who is acknowledged and who is silenced, what is noted and what is glossed over – all these things matter even if the subject matter seems very straightforward. Above all, Tyson Yunkaporta teaches me again and again the importance of Noticing. It's not a coincidence that I read Sand Talk in the second year of Melbourne's interminable Covid lockdowns, and that I started noticing things around my neighbourhood soon after – the flowering of different plant species, the changes in the bird populations from month to month, and even how vehicle traffic patterns had shifted. I'd never really paid much attention to any of that previously.
Speaking of noticing: something I noticed after finishing Right story, wrong story was that I suddenly had the urge to draw. It struck me because I had exactly the same compulsion after I read Sand Talk. Yunkaporta makes a carving in an object for each chapter in each of his books. This no doubt influenced me. But there must be more to it than that. Or rather, the way he writes, influenced by the way he carves patterns to record his ideas, influences me to think in patterns too. I don't really know what's going on here but it's definitely a thing. An important thing to understand here is that I am in no way "artistic". I don't habitually draw. Or at least I didn't until I started reading Yunkaporta's work. And I'm not sure that's really the point anyway. These are tools for working out what you're thinking, and recording it as a mnemonic device. I'm not sure about the mnemonic value of my drawings on digital paper, but I do find it helps me think things through and sometimes express things I can't quite articulate.
During lockdown I was surprised to find that the thing I most missed about the office was having a whiteboard to help me think things through. This is of course related, but I'm cautious about linking them too closely because ...well, I guess I don't like connecting what I think of as more wholesome brain-expanding processes with things that are just making me more effective as an Organisation Man. On this note, I recently discovered Abby Covert's Stuck? Diagrams help. via Tracy Forzaglia's MOD Librarian blog. I haven't read it yet but I'm thinking I'll probably splash out and get the ebook since diagramming things out seems to be so helpful for me.
Anyway, there's not some profound point to this post. I just decided to share some thoughts instead of being crippled by anxiety about whether my next blog post would be The Perfect Take. It never will be.
Open Data Editor: what we learned from user research / Open Knowledge Foundation
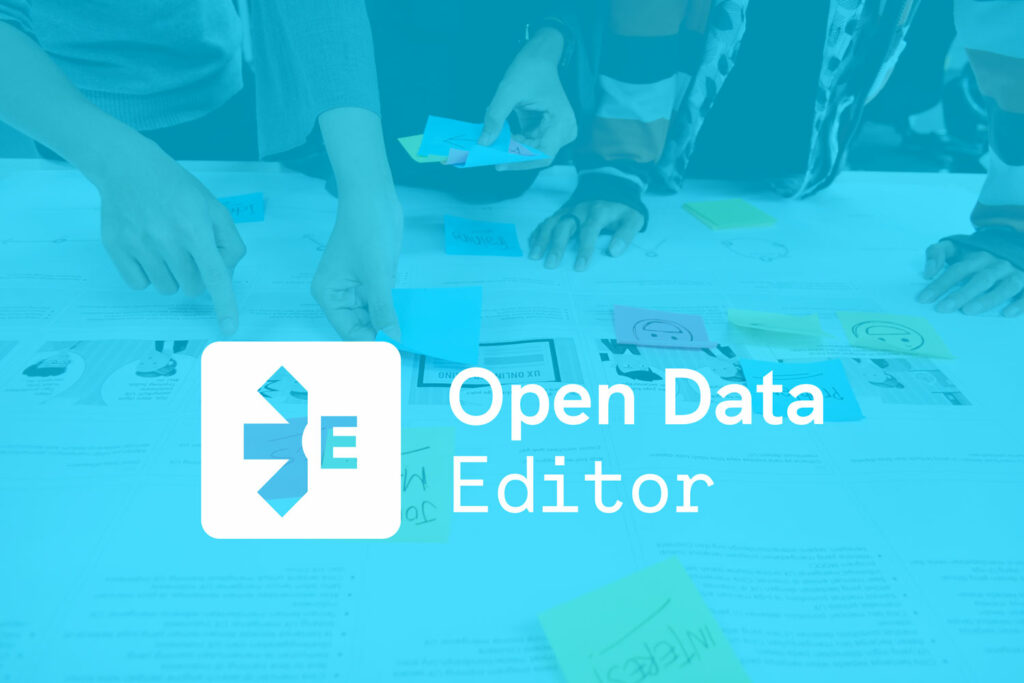
As announced in January, this year the Open Knowledge Foundation (OKFN) team is working to develop a stable version of the Open Data Editor (ODE) application. Thanks to financial support from the Patrick J. McGovern Foundation, we will be able to create a no-code tool for data manipulation and publishing that is accessible to everyone, unlocking the power of data for key groups including scientists, journalists and data activists.
[Disclaimer: Open Data Editor is currently available for download and testing in beta. We are working on a stable version. Updates will be announced throughout the year. Learn more here.]
Since the beginning of the year, we’ve been working on building the ODE team and conducting the first phase of user research. We have interviewed 10 people so far, covering different user profiles such as journalists, people working in NGOs and the private sector, and data practitioners in general.
The Open Data Editor is built on top of Frictionless Data specifications and software, and is an example of a simple, open-by-design alternative to the complex software offered by the Big Tech industry. Developing this type of technology is part of our current strategic focus on promoting and supporting the development of open digital public infrastructure that is accessible to all.
As part of this, we want to open up this process in a series of blogs, sharing with the community and those interested in the world of open data how each stage of the creation of this software is developing.
What have we learned so far?
- Put people first: organisations need to spend more time on user research. Organisations can lose money and spend unnecessary time on things that may not be as useful as they think if they don’t reach out to their community and try to understand their problems before building solutions. This may sound obvious, but it happens all the time.
- Spend more time thinking about the problem you are trying to solve. Whenever you want to improve a tool, you may be tempted to jump in and try to fix it from a technical point of view. This can create a bigger problem. It’s important to take a step back, learn everything you can about the tool, and talk to potential users to understand if what the technology is trying to solve is a real problem for them.
- Build diverse and interdisciplinary teams. The current OKFN team working on ODE includes three software developers, a product owner and a project manager. We all have different expertise and backgrounds, which is key to being able to put ourselves in the shoes of our potential users. Most importantly, we are all data practitioners ourselves!
- Do not reinvent the wheel: check out the resources your community has already made available. This is also a good way to reuse resources that your community has opened up, so that you spend less time on key parts of your work. For example, during our research process we used the amazing Discovery Kit created by the Open Contracting Partnership. Although the toolkit was originally developed to help teams build tools and software using open contracting data, we followed the advice and used some elements, such as their user personas, to adapt it for our specific work.
- Share and iterate your ideas with people outside your organisation. Getting external insights is a very good practice for those building open source products. “Sharing is caring” is good for you and your products
Initial findings
After the first round of user interviews, here are the first conclusions on the difficulties and current state of the art of tabular open data according to data practitioners.
- Same old problems. Data practitioners still spend a lot of time exploring and cleaning data. Analysis is only a small part.
- The struggle with PDFs continues. Some respondents explained how they have to manually copy and paste data or use technologies such as Tabula to extract tables from PDFs.
- Preferred tools for exploring and cleaning data: Spreadsheet tools like Google Sheets, Open Office and Excel.
- Favourite features to start exploring the data: Pivot tables and filters.
- Generative AI “not for data analysis”. Data practitioners, especially journalists, are reluctant to use AI for data analysis or to draw conclusions from the data they’re working with. They don’t want to share their datasets without knowing how they’re being used (privacy concerns), and because it’s impossible to reconstruct what the technology is doing to achieve specific results.
You can also find more details in the following presentation.
If you want to get more closely involved with the development of the Open Data Editor application, you can express your interest in joining one of the testing sessions by filling this form.
You can also email us at info@okfn.org, follow the GitHub repository or join the Frictionless Data community. We meet once a month.
#ODDStories 2024 @ Detroit, USA 🇺🇸 / Open Knowledge Foundation
DETROITography organized an Open Data Day hybrid event on 7 March 2024 at the Purdy/Kresge Library on the Wayne State University (WSU) campus in Detroit, Michigan, USA. The presentation focused on neighborhood data discovery through the relaunched CKAN data portal and catalog called DetroitData, crowdsourced input on community boundaries via Detroit-Neighborhoods.com, and the launch of an open data platform to track the city of Detroit’s progress on the SDGs using OpenSDG.
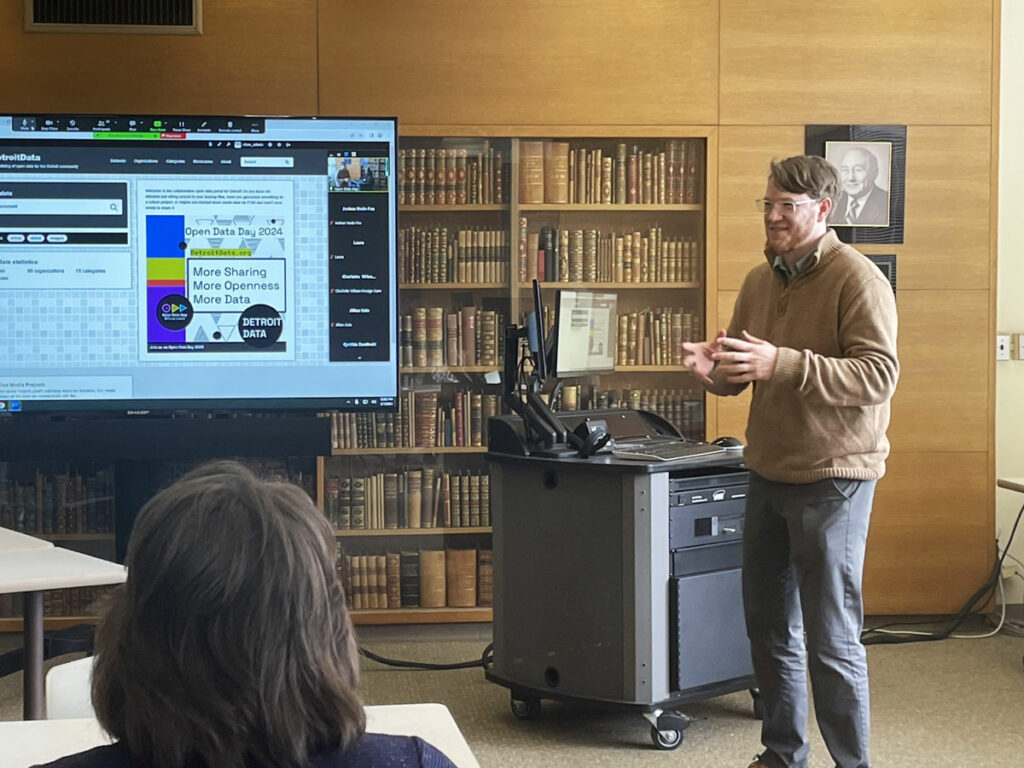
I led the presentation first focusing on the relaunch of DetroitData as the open data catalog for the city. A few city and regional entities have their own open data portals, but nonprofits and community organizations don’t have a place to share and contribute to the city’s data narrative. I also highlighted how DetroitData can serve as an essential resource for journalists who utilize FOIA or “freedom of information act” requests to open up data from local government entities. DetroitData currently compiles over 1,000 datasets shared by more than 50 local organizations.
The next presentation featured the Detroit-Neighborhoods.com tool to collect and analyze community input on neighborhood boundaries. Detroit has a long history of defining neighborhoods without much community engagement or feedback. The tool specifically tallies a submitted response related to how the city government defines a neighborhood area as well as what the degree of agreement there is among those submitting. Alex shared that the hope for the site is that community groups can use it as a data-driven tool to better advocate for their neighborhood boundaries.
Finally, the session ended with a discussion around the Sustainable Development Goals (SDGs) in Detroit and how well they are tracked at the city level. The OpenSDG platform applied to Detroit will slowly be adding new datasets that highlight where the city is at in relation to the global goals. As the only UNESCO City of Design in the USA, Detroit must make concerted efforts to achieve the SDGs for every community and neighborhood. The participants were very excited and engaged with the presentation with detailed questions on community involvement and ensuring clear background information gets shared about datasets. A cohort of WSU Libraries staff were on hand and shared their passion for metadata. Future collaborations are likely as the School of Information Science works to train new librarians on digital tools and metadata maintenance.
About Open Data Day
Open Data Day (ODD) is an annual celebration of open data all over the world. Groups from many countries create local events on the day where they will use open data in their communities.
As a way to increase the representation of different cultures, since 2023 we offer the opportunity for organisations to host an Open Data Day event on the best date within a one-week period. In 2024, a total of 287 events happened all over the world between March 2nd-8th, in 60+ countries using 15 different languages.
All outputs are open for everyone to use and re-use.
In 2024, Open Data Day was also a part of the HOT OpenSummit ’23-24 initiative, a creative programme of global event collaborations that leverages experience, passion and connection to drive strong networks and collective action across the humanitarian open mapping movement
For more information, you can reach out to the Open Knowledge Foundation team by emailing opendataday@okfn.org. You can also join the Open Data Day Google Group to ask for advice or share tips and get connected with others.
Be a Part of the 2024 Virtual DLF Forum: Submit Your Proposal Today / Digital Library Federation
The Council on Library and Information Resources is pleased to announce that we have opened the Call for Proposals for the virtual Digital Library Federation’s (DLF) Forum happening online, October 22-23, 2024.
We encourage proposals from members and non-members; regulars and newcomers; digital library practitioners from all sectors (higher education, museums and cultural heritage, public libraries, archives, etc.) and those in adjacent fields such as institutional research and educational technology; and students, early- and mid-career professionals and senior staff alike. We especially welcome proposals from individuals who bring diverse professional and life experiences to the conference, including those from underrepresented or historically excluded racial, ethnic, or religious backgrounds, immigrants, veterans, those with disabilities, and people of all sexual orientations or gender identities.
Learn more about our event and session formats, view the Call for Proposals, and submit.
The submission deadline is Wednesday, May 15, at 11:59pm Eastern Time.
Curious about submitting a proposal but not sure where to start?
Join the next CFP Office Hours on Tuesday, April 30 at 1pm ET USA to learn more about the virtual DLF Forum and session types for our virtual event. Register in advance.
If you have any questions, please write to us at forum@diglib.org. We’re looking forward to seeing you online this fall.
P.S. Want to stay updated on all things DLF Forum? Subscribe to our Forum newsletter.
The post Be a Part of the 2024 Virtual DLF Forum: Submit Your Proposal Today appeared first on DLF.
Not All Staying is the Same: Unpacking Retention and Turnover in Academic Libraries / In the Library, With the Lead Pipe
In Brief: Although the academic libraries profession recognizes that retention is a complex and important issue, especially for advancing diversity, equity and inclusion (DEI) initiatives and supporting BIPOC librarians, the library literature largely avoids defining or providing a measurement for retention at all. In this paper we propose an original nuanced definition of retention. We draw from existing research on workplace dynamics and library culture and our qualitative exploration of academic librarians who have left jobs before they intended. Our research investigated what it was like for them to stay at those jobs after they realized they didn’t want to stay long-term. We argue that structural aspects of the academic library profession (such as emotional investment in the profession, geographic challenges, and role specialization) can lead to librarians staying with organizations longer than they would otherwise, and that this involuntary staying is not functional retention. We explore the distinction between involuntarily staying and voluntarily staying at an organization, as well as the coping strategies library employees may engage in when they involuntarily stay. Finally, we make the argument that functional retention is a relationship between the organization and the individual employee in which both sides are positively contributing to the workplace culture.
By: Samantha Guss, Sojourna Cunningham, Jennifer Stout
A note on language
The authors applied the language of the American Psychological Association’s Journal Article Reporting Standards for Race, Ethnicity, and Culture (JARS–REC) (American Psychological Association, 2023). We are writing from a North American context and acknowledge the ways that race is defined differently based upon national and cultural contexts. The language and understanding of race are not universal and terms and language usage evolve as norms and practices evolve. We chose to use the terms “Black, Indigenous, and People of Color (BIPOC)” and “underrepresented racial and ethnic groups.” Our participants self-identified their race and ethnicity but in an attempt to maintain strict confidentiality, we made the choice to identify the race of the participants using the terminology of BIPOC and only identified the race of the interviewee when making explicit points about race, ethnicity, and culture.
Introduction
In this paper, we propose a nuanced definition of retention of librarians that distinguishes between functional and dysfunctional retention. We do this by integrating existing research on workplace dynamics and library culture with a qualitative exploration of academic librarians who have left jobs before they intended and what it was like for them to stay at those jobs after they realized they didn’t want to stay long-term. As a result of this exploration, we argue that structural aspects of the academic library profession (such as emotional investment in the profession, geographic challenges, and role specialization) can lead to librarians staying with organizations longer than they would otherwise and that this “involuntary staying” is not functional retention.
The Association of College and Research Libraries (ACRL) and the Association of Research Libraries (ARL) have been interested in strengthening recruitment and retention of academic librarians from underrepresented racial and ethnic groups since at least the early 1990s, and in the early 2000s named this issue a priority for the library profession (Neely & Peterson, 2007). Institutions and professional organizations responded by increasing the number of scholarships, training programs, and postgraduate residency programs aimed at supporting new graduates in finding entry-level positions (Boyd et al., 2017). However, for all of the discourse on “recruitment and retention,” a majority of the emphasis has been on recruitment, which is easier to quantify, with little study of how retention functions in an academic library environment (Bugg, 2016). The profession has focused on recruiting more librarians from underrepresented racial and ethnic groups, but we have not prioritized supporting them to stay (Hathcock, 2015). As a result, the numbers of Black, Indigenous, and people of color (BIPOC) librarians has stagnated or in some cases, actually decreased (ALA Office of Research and Statistics, 2017; American Library Association, 2012; Barrientos et al., 2019). Recruitment and retention are both critical to diversity, equity, and inclusion efforts in academic libraries, and failing to improve retention has and will continue to derail these initiatives (Hodge et al., 2021).
As we looked deeper into the literature on retention in academic libraries, we recognized that the concepts of recruitment and retention have become intertwined, with recruitment as the primary focus of the literature, leaving retention insufficiently studied and defined. Research that addresses retention tends to focus on proposed strategies, such as stay interviews (structured interviews aimed at strengthening employee and employer relationships) and mentorship programs (SHRM, n.d.-a). At the same time, there is no agreed-upon definition of retention that would allow us to assess these strategies. ACRL recently published a toolkit for library worker retention that defines retention as “the ability of an organization to reduce turnover among employees and keep employees for as long as possible” (Nevius, 2023). While this definition is a good starting point for discussion, it is vague and immediately raises questions, namely whether keeping employees “for as long as possible” is truly an appropriate goal for academic libraries.
As academic libraries attempt to define and assess retention, we should recognize that involuntary staying can be just as negative an outcome for the individual and the organization as leaving, setting the stage for legacy toxicity, which persists even through leadership changes (Kendrick, 2023). At the same time, trying to understand these dynamics will help us design policies and programs to encourage work environments that are conducive to individual and organizational goals, and propagate structural solutions across the profession. We argue that functional retention is a positive, engaged relationship between the employee and the organization, where both are contributing to a workplace that is positive, safe, and harmonious.
Toward A Definition of Retention
Most studies on retention within academic library literature reflect “retention management,” defined as strategic initiatives aimed at reducing turnover within institutions, where turnover is defined as employees leaving the organization (SHRM, n.d.-b, 2023). These studies touch on many types of initiatives, including strategies to retain librarians in their current positions (Musser, 2001; Strothmann & Ohler, 2011) and factors that encourage retention such as onboarding (Chapman, 2009; Hall-Ellis, 2014). More specifically, researchers have examined mentoring of librarians from underrepresented racial and ethnic groups (Olivas & Ma, 2009); professional development for librarians from underrepresented racial and ethnic groups (Acree et al., 2001); and inquiry into why librarians leave positions (Heady et al., 2020).
There is clear recognition in the library literature that retention is important and complex, yet we were unable to find a solid definition or agreed-upon understanding of what it means to successfully retain someone prior to ACRL’s definition, which only came online in mid-2023 (Nevius, 2023). Some researchers have acknowledged the complications inherent in retention efforts. Both Bugg (2016) and Musser (2001) state that retention requires long term communication and commitment from multiple actors across the academic libraries profession, not just by individual institutions. Consequently, the profession tends to focus its efforts on recruitment and programs like mentorship to attempt to retain employees and does not address bigger and more complex issues such as workplace culture and environment, job satisfaction, bullying, toxicity, racism, and low morale (Alajmi & Alasousi, 2018; Dewitt-Miller & Crawford, 2020; Freedman & Vreven, 2016; Kendrick, 2017; Kendrick & Damasco, 2019).
Within human resources management (HRM) literature, the definition of retention varies depending on the field in question. In a 2015 scoping review, Al-Emadi and colleagues acknowledge the variety of ways retention is defined within HRM and present a working definition of retention as “initiatives taken by management to keep employees from leaving the organization, such as rewarding employees for performing their jobs effectively, ensuring harmonious working relations between employees and managers, and maintaining a safe, healthy work environment” (Al-Emadi et al., 2015, p. 8). This definition also reflects “retention management” as described above, but is helpful in that it explains retention as an action that an organization takes (rather than a passive state that employees are in) and underscores the importance of the workplace environment and relationships between employees and managers. At the same time, this definition limits potential assessment for academic libraries.
Why Do Librarians Stay?
There are many reasons academic librarians remain with organizations where they are generally satisfied and feel that their personal and professional needs are being met; these reasons have been explored in the Library and Information Science (LIS) literature (Alajmi & Alasousi, 2018; Kawasaki, 2006), as well as the HRM literature (SHRM, 2023). However, we were interested in reasons why academic librarians may stay in organizations when they are not happy.
The research describing the academic librarian job market generally concludes that academic library jobs are difficult to obtain. Tewell points out that historically, the librarianship job narrative has moved between a model of “job scarcity” and a “recruitment crisis” but ultimately, entry level jobs are considered highly competitive (2012, p. 408). Other researchers have examined the perceived necessity of additional educational requirements to be considered competitive for an academic librarian position (Ferguson, 2016), the precariousness of academic librarian positions (Henninger et al., 2020), and the experiences of part time librarians looking for full time employment (Wilkinson, 2015). Ultimately, the research demonstrates that there is a perception of job scarcity within the academic libraries profession, meaning that transitioning to a new job is not as straightforward as in some other fields and may be a significant reason some librarians stay at their current jobs.
Many librarians are bound geographically to their libraries and workplaces (Kendrick, 2021; Ortega, 2017). Library workers who have familial and/or care-taking obligations cannot easily move to a new location for a new position, and may be subject to a limited job market in their current location. Many positions in academic libraries are highly specialized and draw from a national candidate pool, so even if there are several academic libraries available, there is no guarantee that an appropriate position will become available or that any individual can count on being hired. Likewise, career advancement can often require changing organizations or moving away from a home location, especially given that many academic libraries have flat organizational structures and very small staffs (Ortega, 2017). Academic librarians are also subject to the “two-body problem,” where dual-career couples must navigate job markets in a way that accommodates both careers (Fisher, 2015).
Petersen (2023) reinforces this quandary, writing about career paths that force workers to leave their bases of support to maintain their specialized livelihoods and the resulting challenges workers face in creating new support networks as adults. She describes this situation as a type of “job lock,” a term often applied to situations where employees are stuck at jobs because of non-portable benefits (like health insurance in the U.S.) but has also been used to describe other situations where employees feel unable to leave jobs (Huysse-Gaytandjieva et al., 2013, p. 588). Ritter (2023) also discusses the costs of transient careers often required of higher education workers and coins the term “academic stranger,” referring to a mobile-by-default mentality that encourages workers to accept work conditions that are socially destabilizing. Pho and Fife (2023) point to a similar narrative in academic libraries that mobility and the resulting emotional connections and disconnections are expected as librarians move from place to place to support their professional needs. Consequently, it’s natural for academic librarians to build their lives around their jobs and the people at those jobs, which can make it hard to leave an unsatisfying position.
Spencer (2022) describes this phenomenon as professional “hypermobility” or “nomadism” and emphasizes that it has positive and negative aspects, but needs to be more transparent to those considering entering the academic libraries field. Petersen argues that in fields “where jobs are scarce, [geographic] mobility is a privilege” that creates inequality in the job market and reinforces the idea that holding any job is lucky: “you take what you get and the expected posture is gratitude” (2023).
Another reason why librarians might be hesitant to leave is because they feel deeply emotionally connected to their job. Ettarh’s work on “vocational awe” in the library profession, described as “the set of ideas, values, and assumptions librarians have about themselves and the profession that result in beliefs that libraries as institutions are inherently good and sacred, and therefore beyond critique,” sheds light on why librarians may stay in jobs that exploit them (2018). Similarly, Petersen (2022) describes library jobs as “passion jobs,” which she identifies as “prime for exploitation,” because they are often feminized and devalued. She describes how many nonprofit organizations, including libraries, work with as few staff as possible and don’t always fill vacant positions; as a result, individuals may feel guilty for leaving because of likely consequences for their coworkers and/or patrons.
What Pushes Librarians to Leave?
Over the past decade, there has been a fair amount of focus on determining why academic librarians leave their organizations; specifically related to bullying, toxicity, and low morale in academic libraries (for example, Freedman & Vreven, 2016; Heady et al., 2020; Kendrick, 2017, 2021; Ortega, 2017). Although most of these studies assess librarians after they’ve left a job, this body of research offers insight into why a librarian might move toward involuntary staying.
Kendrick discusses the concept of a “trigger event,” which is described as “an unexpected negative event or a relationship that developed in an unexpected and negative manner” (Kendrick, 2017, p. 851). Participants in her study “perceived trigger events as the beginning of a long-term abuse cycle” (p. 853). Most employees don’t leave their job immediately after a trigger event occurs, if ever, but are forced to reevaluate their understanding of the organization and their role there. It is this mindset shift that we will describe as moving toward involuntary staying.
Ortega’s (2017) definition of toxic leadership, characterized by “egregious actions” and “causing considerable and long-lasting damage,” can help us understand traits of toxic workplaces where employees no longer want to stay (p. 35). Ortega’s and Kendrick’s (2017) studies both support the idea that toxicity becomes an ingrained part of the organizational culture. When employees leave toxic library workplaces, they are not leaving because of one bad day or even one bad leader, but because of a pattern and culture of toxicity. Research from Heady, et al. (2020) reinforces that when academic librarians leave, they are “not fleeing their positions, they are fleeing work environments they feel are toxic” (p. 591) and that morale, culture, library administration, and direct supervisors were the top factors in their decisions to leave (p. 585–586).
Understanding Turnover
As we attempt to better understand retention, it’s helpful to begin with a framework for understanding turnover, commonly defined in the HRM field as the “movement of employees out of the organization” (SHRM, n.d.-b). There are different types of turnover, as shown in Figure 1.
Reproduced by permission of the Society for Human Resource Management (SHRM) Retaining talent: A guide to analyzing and managing employee turnover by D. G. Allen, 2008, p. 2 (https://blog.shrm.org/sites/default/files/reports/Retaining%20Talent-%20A%20Guide%20(Q1).pdf). (Alexandria, VA: Society for Human Resource Management). © 2018 by SHRM. All rights reserved.”
Allen divides turnover into involuntary (i.e. an employee is fired or otherwise dismissed at the organization’s discretion) and voluntary (i.e. an employee leaves the organization because they want to). Even considering only voluntary turnover, there are many varied reasons that would require different kinds of attention from an organization. If turnover is measured simply as the total number of workers who left the organization in a set period of time (SHRM, n.d.-b), it’s easy to see why an organization would struggle to understand if they had a retention problem or not, or what they might be able to do about it. Therefore, “reducing turnover” is too simplistic a goal (Nevius, 2023).
The opposite of turnover is staying in an organization, which we argue is not the same thing as true retention. Staying doesn’t automatically mean that an employee is content or that retention initiatives are working. Turnover may reasonably be defined as failed retention, but retention should not be simply the absence of turnover.
Understanding Retention through Staying
Based on Allen’s 2008 classification of turnover, we can also classify different categories of staying, defined as the opposite of turnover, or an employee who has not left the organization. We use the terms voluntary and involuntary to describe staying, similar to Allen’s approach, but in the case of staying, it’s important to note that voluntary staying and involuntary staying are mindsets and, unlike turnover, are not simply classifications of an event. Voluntary staying is a state of mind where an employee is generally satisfied and engaged to the point that they are not looking for other positions or needing coping strategies to survive their current work life. Generally, employees join organizations (i.e. begin new positions) voluntarily staying.
When an employee becomes dissatisfied, disengaged, and starts to use coping strategies to survive their work life, they have moved from voluntarily staying to involuntarily staying. This does not mean that they will necessarily begin looking for another job right away, or ever. While the employee may be experiencing job-lock at the same time (when external factors such as non-portable health benefits prevent someone from leaving their job), involuntary staying describes their mindset and feelings about the job. In our study, we interviewed librarians who had experienced this involuntarily staying state at a previous job.
Our qualitative research described in this paper sought to explore the moments or events that caused librarians to leave jobs before they intended and their experiences once they decided to leave. Given how difficult it is to switch jobs in our field, what was it that tipped the scales for them, making staying untenable? What were their experiences and what could we learn from them about how to define and improve retention of librarians in the future? Through this exploration, we learned about these original questions, but also about the interplay between library culture, management, leaving and staying, and turnover and retention.
Methods
The qualitative data was gathered through 10 semi-structured interviews of academic librarians who left jobs sooner than they’d planned, for job-related reasons as opposed to personal reasons, such as moving to be closer to family. Using an interview guide (See Appendix A), we sought to learn about the interviewees’ experiences as they realized that they could no longer stay at their job and what happened before and after that realization. While we suspected that these experiences would be a little different for everyone, we hoped to expand our general understanding of times when employees were not retained by their organization (that is, when retention failed) and the effects on the individual and the organization in the time period between an employee realizing they wanted to leave and actually leaving.
The interviews, lasting approximately one hour each, were conducted between January 2021–June 2021. Participants were drawn from a pool of 57 responses to a screening survey seeking participants who had left a job before they had planned to. From our pool of respondents, we selected seven participants who identified as BIPOC (a sample that included participants identifying as non-Black POC, but none who identified as indigenous) and three participants who identified as white, using a random number generator to select participants from each strata (see Appendix B). We intentionally oversampled BIPOC librarians, to center their voices as we explored themes related to race and white supremacy culture, and acknowledging that people from historically marginalized groups are often positioned to have unique insight into dominant cultures. Our screening survey did not specify whether respondents should be managers or non-managers themselves, and we learned during our interviews that both were represented in our sample.
Our overall sample size of 10 interviews was relatively small. This was partially for practical reasons, but also because our research team agreed after our 10 interviews that we’d reached sufficient saturation; that is, we believed additional interviews would not yield new themes (Strauss & Corbin, 2014, p. 148).
Interviews were conducted via Zoom by two researchers and audio-recorded with participants’ permission. Interview audio was transcribed using Descript and copyedited and de-identified by the researchers. Consistent with our consent agreement, participants are identified in our writing only by an assigned code number (e.g. “Interviewee 7”), their self-identified gender pronouns, and whether they self-identified as BIPOC or white.
Each transcription was coded by at least two researchers using grounded theory methodology, per Strauss and Corbin (2014), meaning that a codebook was developed during analysis based on recurring themes across interviews. In addition to exploring our research questions, a secondary goal was to provide space for participants to share their experiences, which many had never shared before, and to have these experiences heard and validated as legitimate (see Cunningham et al., 2023).
Results
The majority of our interviewees started out their positions in a state of voluntary staying: they expressed positive feelings about their jobs at the start and described minimal or no red flags during interviews. They discussed things like specific job duties or opportunities that appealed to them, positive feelings about compensation, and a feeling that they could “have a career there” (Interviewee 2).
Over time, interviewees either experienced “trigger events” (“an unexpected negative event or a relationship that developed in an unexpected and negative manner,” as described by Kendrick, 2017, p. 851) or general increasing awareness of things being “off,” specifically related to organizational culture. These trigger events and off feelings led our interviewees to move from a state of voluntarily staying to involuntarily staying.
One example of this was Interviewee 9, who was happily in her job for four years when a radical restructuring of the library departments occurred with no warning. Interviewee 9 noted that after this restructuring occurred, she and her colleagues began engaging in “checked out” behavior.
Oh yeah. We all checked out for sure…Well, one thing you saw was people left immediately at five [and] people didn’t come in early. There was not as much engagement just on the social level. Like before this[–]and this is gonna sound like some kind of weird, perfect land[–but] we went to eat lunch together all at the same time in the lunchroom. And people had a good social relationship. After [the restructuring,] people would avoid each other.
A few months after the initial restructuring, Interviewee 9 had a defining moment in which she received explicit discouragement from her new supervisor:
I was a high achiever. I seemed to be moving forward. And my boss turned to me, my new boss, in a meeting with other people there and said, “there are people in this organization that think that they are moving forward and they will never move forward under me,” while staring at me very directly. So, I mean, I didn’t have a career there anymore, so.
This was the final straw for Interviewee 9 and she began looking for a new job immediately. This example highlights that “involuntarily staying” is more of a frame of mind than a specific period of time and also that an employee can be involuntarily staying while not actively looking for another job. In the case of Interviewee 9, she went from happy in her job to one of many “checked out” people and finally to actively looking for a new job once it became clear that she was on her new supervisor’s “shit list.”
Interviewee 5 presents a contrasting example in which the Interviewee stayed in his position for a longer period of time:
I had trigger events, yes. Now having said that, it still took me five years to leave that job. And there’s a lot of factors in that.
Interviewee 5 described two trigger events in which he clashed with library leadership and was written up. He was at the job for a total of five years, but notes that his job search was “four years long,” indicating a very long period of involuntarily staying. Ultimately, he left without a job offer when he recognized that his health was suffering:
When I finally left that institution, I left without having a job offer at all. So it was important that I needed to get out of there and it was tak[ing] tolls on my health and everything else. My coping mechanisms had went [sic] from healthy to unhealthy, so it was time to go.
A third example is from Interviewee 1 who was on a three-year contract with her institution and noted, “I can live through anything for three years.” However, this interviewee experienced a trigger event where her boss changed a positive work review to a negative one after she refused to provide him with personal information. She realized that she could not stay, but was also conflicted about leaving colleagues and patrons:
I just felt like that just killed it. Like there was no resurrecting that relationship. After that, I worked out the rest of that contract because […] you know, I did like the students, we were very close to several of them and my colleagues and I didn’t want to leave them in the lurch because [if] I left, someone else would pick up the slack and there wasn’t enough of them to go around in the first place. So I was very mindful of that, but that was the event where I’m like, you know, can this marriage be saved? No, that was it.
As explained earlier, “involuntarily staying” is a mindset rather than a specific period of time. In our interviews, the time period between the interviewee realizing they could no longer stay in a job and them actually leaving ranged from nearly immediately to years. Their sense of immediacy also ranged from relatively casual to dire.
What all our interviewees had in common is that they moved from voluntarily staying to involuntarily staying and engaged in a range of coping mechanisms to survive once they realized that staying was untenable and until they were able to leave. This is congruent with Kendrick’s stages of low morale when onset of coping strategies eventually follows trigger events and exposure to workplace abuse or neglect (Kendrick, 2021, p. 12).
Borrowing again from Allen (2008), we use the terms “functional” and “dysfunctional” to classify the coping mechanisms described by our interview participants. One important point of departure is that Allen’s terminology views outcomes from the organization’s perspective, while our study is interested in outcomes for the employee and the organization. Therefore, we classify coping strategies as functional, or, congruous with the health of the individual and the organization, or dysfunctional, or unhealthy for the individual and/or the organization (see Figure 3).
Some examples of functional coping strategies recounted by interviewees:
- Regularly validating yourself and your feelings:
[I’d say:] ‘You’re not crazy. You’re not imagining this. They’re treating you as poorly as you think they are.’ And I think a lot of times some of these things flourish because people are made to feel like they’re exaggerating or overly sensitive, and they don’t have the support system to know that what they’re feeling is entirely valid. (Interviewee 1)
- Seeking perspective from outside the organization:
I would say you know, find your mentor, who are [sic] hopefully outside of your institution, because you know, if you’re in a toxic workplace and your mentor is inside, if they’ve been there for a while, it can look like it’s normal when it’s really not normal. So you need someone on the outside to help you to kind of navigate bad experience[s]. (Interviewee 10)
- Regularly reminding oneself that “it’s not personal”:
I [would tell someone in a similar situation] to remember that it is not personal… And the reason that it’s important people know that is, one, it feels a lot worse if it feels personal. But the other thing is, that part of that feeling of personalness makes it harder for you to decide ‘screw this, I’m going.’ You know, you recognize that you are a cog in a capitalist machine. You can take your little cog-self somewhere else where they will pay you more. (Interviewee 9)
- Prioritizing physical and mental health.
- Finding healthy ways to release anger and frustration.
- Finding validation and support from ally colleagues, especially among BIPOC colleagues.
- Setting and practicing strong boundaries around work and work interactions.
Examples of dysfunctional coping strategies described by interviewees:
- Warning new folks not to try too hard and to “stay in their lanes.” This was practiced by both interviewees and their colleagues and was described by multiple interviewees as having both functional and dysfunctional aspects.
[I was told:] If you just do what everybody tells you to do and stay away from the riff-raff…and stay below the fray you’ll do well [and] move up here. (Interviewee 5)
[The advice I was given was:] ‘Stay under the radar. Don’t try to do too much.’ (Interviewee 3)
- Participating in a culture of resentment and grudge-holding.
[There were] existing tensions and grudges and resentment, that’s been building over years and it’s created such a hostile environment where you can’t necessarily stay neutral. (Interviewee 3)
- Disengaging with meaningful work or “checking out”.
I just put the rest on autopilot, right. I stopped sharing ideas…I devoted myself to my job search. (Interviewee 6)
- Creating or reinforcing organizational silos.
It’s also important to note that the line between functional and dysfunctional coping strategies is thin and often not very meaningful without context. A coping mechanism that is functional in one scenario may be dysfunctional in another. Likewise, a coping mechanism that is functional from an individual’s perspective may sometimes be counterproductive from an organizational perspective, or vice versa. Often interviewees described using functional and dysfunctional coping strategies simultaneously. Additionally, we are not arguing that all employees who engage in harmful behaviors are doing so because they have moved into a state of involuntary staying.
Discussion
The library literature has established that toxic leadership and organizational culture can cause or contribute to individuals leaving their organizations. Our study suggests that organizational culture also influences whether an employee is voluntarily or involuntarily staying at the organization, and whether their coping strategies are functional or dysfunctional. We can also see, just as all employees participate in organizational culture in some way, that employees’ dysfunctional coping strategies can feed into and reinforce toxic culture, creating a feedback loop.
Further, academic librarianship as a profession has structural features, described earlier, that can prolong an employee’s period of involuntary staying at an organization and therefore exacerbate the situation for all involved. Our findings about dysfunctional coping mechanisms complicate the ACRL Toolkit’s definition of retention that includes a goal of keeping employees “for as long as possible” (Nevius, 2023). In some scenarios, keeping employees is not in the best interest of the individual or the organization.
It’s important to note, though, that this does not mean that leaders of an organization should force an employee to leave. While managers and leaders should proactively address any disruptive behaviors from employees (many of which we’ve described here as dysfunctional coping strategies), the onus is on the organization to confront toxic culture and not punish individual employees for the ways they try to survive toxic work environments. It should also be recognized that disruptive behavior lies on a continuum; there are certain actors in libraries who would label calling out racist or homophobic practices as disruptive. This can be particularly fraught for employees who are expected to be “library nice,” a framework describing a highly “racialized and gendered form of workplace oppression,” where being perceived as nice is “more important than [their] knowledge, skill, or effectiveness” (Kendrick, 2021, p. 18).
Instead, we propose that retention in academic libraries is about building and maintaining a relationship between the organization and its employees that supports employees to voluntarily stay. Retention studies and strategies should take into account the different varieties of turnover and staying to help organizations honestly assess how they are building cultures that encourage employees to voluntarily stay, with the active acknowledgement that those from underrepresented racial and ethnic groups are often structurally situated to be the most negatively impacted by toxic library culture. Initiatives to improve retention should center employees’ well-being and agency, recognizing that employees and organizations both have much to gain from improving functional retention, but responsibility for bringing retention forward as an organizational focus area ultimately lies with library leaders.
Functional retention requires good leadership, but is also dependent on building and solidifying a culture of accountability, transparency, open discussion, anti-racism, vulnerability, safety, and courtesy that is resilient to legacy toxicity and where most or all librarians are staying voluntarily. While leaders can set the stage for this kind of culture, all employees have an influence on the culture and responsibility for its success. To reiterate, functional retention is a positive relationship between the employee and the organization, with both sides contributing to a workplace that is positive, emotionally and physically safe, and harmonious.
Recommendations for organizations:
- Engage in a regular cycle of assessment.
- Be proactive about retention. Recognize that functional retention is built through consistent, deliberate actions.
- Seek to understand turnover at your organization. This is important even in job markets where employers have the upper hand over job-seekers (as is true for many academic librarian positions).
- Seek to understand retention at your organization. When measuring retention, aim to learn whether your employees are staying voluntarily or involuntarily.
- Strategies such as “stay interviews” (a corollary to exit interviews) and “intent to stay” instruments (developed in the field of nursing) may be useful, but it isn’t clear how often either have been deployed in academic libraries thus far and how effective they will be in this environment (Kosmoski & Calkin, 1986; Nevius, 2023).
- Recognize that not all employees may feel safe answering truthfully.
- Ask employees what could improve their experience and listen to their answers.
- Use their answers to design or transform your retention strategies.
Reflections & Future Directions
We were able to learn a lot about these issues from our literature review, interviews, and analysis, and we hope that our research opens many doors for future study.
Our small sample size, though appropriate for qualitative inquiry, means that we can’t say anything meaningful about differences between demographic groups (e.g. whether BIPOC librarians might experience longer periods of involuntary staying), the potential impacts of other intersecting identities beyond race, or whether our findings are truly generalizable. It would be desirable in the future to have quantitative evidence and a representative sample. Additionally, future study of involuntary staying and its implications for functional retention should also include experiences of librarians who are involuntarily staying and have not left their organizations.
We continue to be curious about many of the threads unearthed in our data: for example, the role of training for managers, the responsibilities of professional organizations, and the roles of human resources professionals or ombudspeople.
Finally, the COVID-19 pandemic was responsible for igniting new questions across all sectors about employees’ relationships to work and their employers. While we believe that there are structural aspects of higher education and academic libraries that distinguish our profession, it will be essential to link studies of library workplaces more closely with research and thinkers from many fields outside our own. For example, how did academic libraries’ responses to the pandemic change the way librarians felt about their organizations and their roles, and how might this impact whether they are voluntarily or involuntarily staying? How does the framing of “quiet quitting,” or doing the minimum requirements for a job, impact involuntarily staying? How should changing societal attitudes about mutual aid and collective care factor into our approaches to measuring functional retention? These are important questions that we hope will be incorporated into future explorations.
Acknowledgments
We would like to express our thanks to Jessica Schomberg, Brittany Paloma Fiedler, and Kaetrena Davis Kendrick for their time and flexibility in guiding us through the publishing process. We are genuinely grateful for their constructive and insightful feedback and suggestions at every stage.
This article represents several years of workshopping and sharpening our ideas through conversations with each other, colleagues, and with audience members from presentations at several conferences; we appreciate all of these contributions.
We would also like to recognize the grant from the Friends of Boatwright Library at the University of Richmond that allowed us to compensate our interviewees for their time.
Finally, we are sincerely grateful to our interviewees, who each generously shared their stories, even when they were painful, so that others may feel less alone and in service of building a better profession for all of us.
Appendix A: Semi-structured interview guide
However you answer, we aren’t here to judge you. We are coming from a place of research and empowerment. Your experiences are valid and are being heard.
Before we begin, we want to review a piece of the Informed Consent Statement you signed — as stated there, public reports of our research findings will invoke participants by a pseudonym and job title only, and that you will be given the chance to designate your own job title. This could be more specific (like “social sciences liaison librarian”), less specific (“liaison librarian”), or very general (“academic librarian”) depending on your preference. You are also welcome to choose your own pseudonym if you’d like.
- What appealed to you about Job 1?
- What was your interview process like for Job 1? Did you feel any red flags? In retrospect?
- If someone was working at this organization, what would you like them to know?
- How was the library structured administratively? Where did you fit in the hierarchical structure of the library? Who did you directly report to? Did you have any direct reports?
- What aspects did you like about your daily job duties?
- What aspects did you dislike about your daily job?
- Did you have colleagues you trusted?
- How were they able to navigate their jobs?
- Did you have support?
- How did that support manifest?
- Kendrick (2017) defines trigger events as “an unexpected negative event or a relationship that developed in an unexpected and negative manner.” Was there a trigger event for you?
- How did you begin your new job search?
- Did you feel that the environment was personal, or was it part of a larger cultural problem?
- Did HR ever seem like an option?
- Were attempts made to retain you*? Is there anything that could have been done to retain you? How would you have liked to see this event handled?
- How could this have been made right?/How would you have preferred supervisors/admin to have addressed the issue?
- In retrospect, would you have done anything differently?
- Do you think that the problems were specific to that library, or library culture in general?
- What would you tell someone else who is in a similar situation?
- What would you tell someone who is interested in applying to your (old) position?
*Note: We used the term “retain” in our interview guide referring to a situation when an organization incentivizes an employee to stay after they’ve indicated that they’re planning to leave (for example, with a salary counter-offer). We acknowledge that this usage of the term and concept is different from how we came to understand retention over the course of this project.
Appendix B
Interviewee # | Race/Ethnicity and Pronouns (self-identified by interviewee) |
1 | BIPOC (she/her) |
2 | White (she/her) |
3 | BIPOC (he/him) |
4 | BIPOC (she/her) |
5 | BIPOC (he/him) |
6 | BIPOC (she/her) |
7 | BIPOC (she/her) |
8 | White (she/her) |
9 | White (she/her) |
10 | BIPOC (she/her) |
References
Acree, E. K., Epps, S. K., Gilmore, Y., & Henriques, C. (2001). Using professional development as a retention tool for underrepresented academic librarians. Journal of Library Administration, 33(1–2), 45–61. https://doi.org/10.1300/J111v33n01_05
ALA Office of Research and Statistics. (2017). 2017 ALA demographic study. ALA Office of Research and Statistics. https://alair.ala.org/handle/11213/19804
Alajmi, B., & Alasousi, H. (2018). Understanding and motivating academic library employees: Theoretical implications. Library Management, 40(3/4), 203–214. https://doi.org/10.1108/LM-10-2017-0111
Al-Emadi, A. A. Q., Schwabenland, C., & Wei, Q. (2015). The vital role of employee retention in human resource management: A literature review. IUP Journal of Organizational Behavior, 14(3), 7–32.
Allen, D. G. (2008). Retaining talent: A guide to analyzing and managing employee turnover (SHRM Foundation’s Effective Practice Guidelines Series). SHRM Foundation. https://blog.shrm.org/sites/default/files/reports/Retaining%20Talent-%20A%20Guide%20(Q1).pdf
American Library Association. (2012). Diversity counts 2009-2010. American Library Association. http://www.ala.org/aboutala/offices/diversity/diversitycounts/2009-2010update
American Psychological Association. (2023, October). Journal article reporting standards for race, ethnicity, and culture (JARS–REC). APA Style. https://apastyle.apa.org/jars/race-ethnicity-culture
Barrientos, Q., Kendrick, K. D., & Flash, K. (2019). Roundtable discussion: Institutional vagaries of retention and Recruitment and the actuality of emotional labor in diversity initiatives. ACRL 2019, Cleveland, OH. https://usclmedford.omeka.net/items/show/51
Boyd, A., Blue, Y., & Im, S. (2017). Evaluation of academic library residency programs in the United States for librarians of color. College & Research Libraries, 78(4), 472–511. https://doi.org/10.5860/crl.78.4.472
Bugg, K. (2016). The perceptions of people color in academic libraries concerning the relationship between retention and advancement as middle managers. Journal of Library Administration, 56(4), 428–443.
Chapman, C. (2009). Retention begins before day one: Orientation and socialization in libraries. New Library World, 110(3/4), 122–135. https://doi.org/10.1108/03074800910941329
Cunningham, S., Guss, S., & Stout, J. (2023). #NotAllLibraries: Toxicity in academic libraries and retention of librarians. In D. M. Mueller (Ed.), Forging the Future: The Proceedings of the ACRL 2023 Conference, March 15–18, 2023, Pittsburgh, Pennsylvania (pp. 125–136). Association of College & Research Libraries. https://www.ala.org/acrl/sites/ala.org.acrl/files/content/conferences/confsandpreconfs/2023/NotAllLibraries.pdf
Dewitt-Miller, E., & Crawford, L. (2020, March 10). Tackling toxicity: Predicting, surviving, & preventing toxic workplaces [Poster]. Electronic Resources & Libraries Conference, March 8-11, 2020. Austin, Texas, United States. https://digital.library.unt.edu/ark:/67531/metadc1637689/
Ettarh, F. (2018). Vocational awe and librarianship: The lies we tell ourselves. In the Library with the Lead Pipe, 10. https://www.inthelibrarywiththeleadpipe.org/2018/vocational-awe/
Ferguson, J. (2016). Additional degree required? Advanced subject knowledge and academic librarianship. Portal: Libraries and the Academy, 16(4), 721–736. https://doi.org/10.1353/pla.2016.0049
Fisher, C. D. (2015). Embracing the “two-body problem”: The case of partnered academics. Industrial and Organizational Psychology, 8(1), 13–18. https://doi.org/10.1017/iop.2014.4
Freedman, S., & Vreven, D. (2016). Workplace incivility and bullying in the library: Perception or reality? College & Research Libraries, 77(6), 727–748. https://doi.org/10.5860/crl.77.6.727
Hall-Ellis, S. D. (2014). Onboarding to improve library retention and productivity. The Bottom Line, 27(4), 138–141.
Hathcock, A. (2015). White librarianship in blackface: Diversity initiatives in LIS. In the Library with the Lead Pipe. https://www.inthelibrarywiththeleadpipe.org/2015/lis-diversity/
Heady, C., Fyn, A. F., Kaufman, A. F., Hosier, A., & Weber, M. (2020). Contributory factors to academic librarian turnover: A mixed-methods study. Journal of Library Administration, 60(6), 579–599. https://doi.org/10.1080/01930826.2020.1748425
Henninger, E., Brons, A., Riley, C., & Yin, C. (2020). Factors associated with the prevalence of precarious positions in Canadian libraries: Statistical analysis of a national job board. Evidence Based Library and Information Practice, 15(3), 78–102. https://doi.org/10.18438/eblip29783
Hodge, T., Winters Palacio, C., King, A., & Lilton, D. (2021, June 22). Why I left the library profession III: DEI perspective. Association of Southeastern Research Libraries (ASERL) DEI Task Force webinar. https://www.aserl.org/event/why-i-left-the-library-profession-iii-dei-perspective/
Huysse-Gaytandjieva, A., Groot, W., & Pavlova, M. (2013). A new perspective on job lock. Social Indicators Research, 112(3), 587–610. https://doi.org/10.1007/s11205-012-0072-2
Kawasaki, J. L. (2006). Retention–-After hiring then what? In Recruiting, Training, and Retention of Science and Technology Librarians. Routledge.
Kendrick, K. D. (2017). The low morale experience of academic librarians: A phenomenological study. Journal of Library Administration, 57(8), 846–878. https://doi.org/10.1080/01930826.2017.1368325
Kendrick, K. D. (2021). Leaving the low-morale experience: A qualitative study. Alki: The Washington Library Association Journal, 37(2), 9–24.
Kendrick, K. D. (2023). The cornered office: A qualitative study of low-morale experiences in formal library leaders. Journal of Library Administration, 63(3), 307–338. https://doi.org/10.1080/01930826.2023.2177924
Kendrick, K. D., & Damasco, I. T. (2019). Low morale in ethnic and racial minority academic librarians: An experiential study. Library Trends, 68(2), 174–212. https://doi.org/10.1353/lib.2019.0036
Kosmoski, K. A., & Calkin, J. D. (1986). Critical care nurses’ intent to stay in their positions. Research in Nursing & Health, 9(1), 3–10. https://doi.org/10.1002/nur.4770090103
Musser, L. R. (2001). Effective retention strategies for diverse employees. Journal of Library Administration, 33(1–2), 63. https://doi.org/10.1300/J111v33n01_06
Neely, T. Y., & Peterson, L. (2007). Achieving racial and ethnic diversity among academic and research librarians: The recruitment, retention, and advancement of librarians of color—A white paper. College & Research Libraries News, 68(9), 562–565. https://doi.org/10.5860/crln.68.9.7869
Nevius, E. (2023, June 14). ACRL library worker retention toolkit: Guide. Association of College & Research Libraries (ACRL). https://acrl.libguides.com/c.php?g=1321674&p=9723675
Olivas, A., & Ma, R. (2009). Increasing retention in minority librarians through mentoring. Electronic Journal of Academic and Special Librarianship, 10(3).
Ortega, A. (2017). Academic libraries and toxic leadership. Chandos Publishing.
Petersen, A. H. (2022, May 1). The librarians are not okay [Substack newsletter]. Culture Study. https://annehelen.substack.com/p/the-librarians-are-not-okay
Petersen, A. H. (2023, April 2). You’d be happier living closer to friends. Why don’t you? [Substack newsletter]. Culture Study. https://annehelen.substack.com/p/youd-be-happier-living-closer-to
Pho, A., & Fife, D. (2023). The cost of librarianship: Relocating for work and finding community. College & Research Libraries News, 84(6), 191–194. https://doi.org/10.5860/crln.84.6.191
Ritter, K. (2023, October 30). Want to be a college professor? Get ready to move. Slate. https://slate.com/human-interest/2023/10/faculty-mobility-academic-job-market.html
SHRM. (n.d.-a). How to conduct stay interviews: 5 key questions. SHRM. Retrieved March 21, 2024, from https://www.shrm.org/topics-tools/news/employee-relations/how-to-conduct-stay-interviews-5-key-questions
SHRM. (n.d.-b). How to determine turnover rate. SHRM. Retrieved January 19, 2024, from https://www.shrm.org/topics-tools/tools/how-to-guides/how-to-determine-turnover-rate
SHRM. (2023, February 21). Managing for employee retention. SHRM. https://www.shrm.org/resourcesandtools/tools-and-samples/toolkits/pages/managing-for-employee-retention.aspx
Spencer, K. (2022, December 13). Uprooted, nomadic, and displaced: The unspoken costs of the upward climb. Information Today. https://newsbreaks.infotoday.com/NewsBreaks/Uprooted-Nomadic-and-Displaced-The-Unspoken-Costs-of-the-Upward-Climb-156012.asp
Strauss, A., & Corbin, J. (2014). Basics of qualitative research: Techniques and procedures for developing grounded theory (4th ed.). Sage. https://us.sagepub.com/en-us/nam/basics-of-qualitative-research/book235578
Strothmann, M., & Ohler, L. A. (2011). Retaining academic librarians: By chance or by design? Library Management, 32(3), 191–208. https://doi.org/10.1108/01435121111112907
Tewell, E. C. (2012). Employment opportunities for new academic librarians: Assessing the availability of entry level jobs. Portal: Libraries and the Academy, 12(4), 407–423. https://doi.org/10.1353/pla.2012.0040
Wilkinson, Z. T. (2015). A human resources dilemma? Emergent themes in the experiences of part-time librarians. Journal of Library Administration, 55(5), 343–361. https://doi.org/10.1080/01930826.2015.1047253
In the Library with the Lead Pipe welcomes substantive discussion about the content of published articles. This includes critical feedback. However, comments that are personal attacks or harassment will not be posted. All comments are moderated before posting to ensure that they comply with the Code of Conduct. The editorial board reviews comments on an infrequent schedule (and sometimes WordPress eats comments), so if you have submitted a comment that abides by the Code of Conduct and it hasn’t been posted within a week, please email us at itlwtlp at gmail dot com!
The Little Garden / David Rosenthal
![]() |
Source |
The reason I'm now able to tell this story is that Tom Jennings, the moving spirit behind the ISP has two posts describing the history of The Little Garden, which was the name the ISP had adopted (from a Chinese restaurant in Palo Alto) when I joined it in May 1993. Tom's perspective from the ISP's point of view contrasts with my perspective — that of a fairly early customer enhanced by information via e-mail from John Gilmore and Tim Pozar, who were both involved far earlier than I.
Jennings starts his story:
Once upon a time, three little businesses wanted a connection to the ARPAnet/internet. The year was 1990 or 1991. John Gilmore, John Romke[y], and Trusted Information Systems (TIS) split the $15K or so it took to get a leased-line and 3COM Brouters to Alternet, with what today you'd call fractional T1. An additional 56K leased line and Brouter brought the 'net up to Gilmore's house, Toad Hall, in San Francisco.The three little businesses were Cygnus Support (John Gilmore), Epilogue Technology (John Romkey) and Trusted Information Systems (Steve Crocker). AlterNet was run by Rick Adams, whom Wikipedia justly describes as an "Internet pioneer". He founded UUNET Technologies:
In the mid-1990s, UUNET was the fastest-growing ISP, outpacing MCI and Sprint. At its peak, Internet traffic was briefly doubling every few months, which translates to 10x growth each year.John Gilmore, a truly wonderful person, had many friends. So what happened was:
As time went on, friends of theirs wanted in on this rare and exciting 'net connection, resulting in Tim Pozar putting an old PC running Phil Karn's KA9Q/NOS program, an amateur radio router capable of TCP/IP, onto Toad Hall's ethernet. Tim installed a pair of modems, then dialed in once and stayed connected 24 hrs/day (Pacific Bell never said you couldn't do that...)Once Tim showed that it was possible, this idea took off:
Eventually the NOS box was full, and more friends wanted in, but everyone was too busy to deal with the hassle.In 1989 Gilmore had co-founded Cygnus Support, whose tagline was "Making free software affordable". TLG got started in August 1990 with the three businesses' nodes on a 56K leased line. One was at Cygnus first office in an apartment complex on University Avenue in Palo Alto. Gilmore and other Cygnus employees had apartments there, so they used 10BASE2 coaxial cable Ethernet to distribute the Internet around the complex. Gilmore notes that they used "nonstandard thin 50-ohm coax in the expansion joints across the driveways when needed". Pozar notes that they paved over the coax!
Somehow, in September 1992, Pozar and Gilmore and I worked out a deal where, I would maintain the thing, collect money to build more NOS boxes and contribute to the monthly Alternet bill, install more people, and get (1) a free connection to the internet and (2) a slice off the top after it exceeded N connections.
By that December, there were enough connections in place that I was pocketing $420/month. By March 1993 there were 11 modem-connected members (as we fancied ourselves).
Gilmore was paying more than $300/mo for modem phone lines supporting the Alt Usenet groups, and realized that for less than that he could have a 56K line from Cygnus to his basement in SF. That led to Pozar and Rich Morin's Canta Forda Computer installing the old PC and becoming the first to use the permanent local call idea.
I knew Gilmore from the early days of Sun Microsystems (he was employee #5), so I first found out about the Point of Presence (PoP) in his basement in late 1992 and really wanted to join in. Alas, there was a snag — the reason the idea worked was that local phone calls were free. From my home in Palo Alto to Toad Hall was a toll call, making it impossibly expensive. But in May 1993 I found out about the PoP on University, 8 blocks from my apartment.
I purchased:
- A phone line from Pacific Bell at the PoP.
- A second phone line at my apartment.
- A pair of Zyxel 9.6Kb modems, one for the PoP and one for my apartment.
![]() |
SparcStation SLC |
![]() |
SparcStation 1+ |
As I recall it ran happily until I passed the apartment on to my step-daughter's family in summer 2000. Seven years of impeccable service. By that time I was working on the LOCKSS program at Stanford, and we had DSL service from Stanford IT. So I went from an ISP with great tech support to an ISP with great support. Then as I relate in ISP Monopolies in September 2001 Palo Alto's Fiber-to-the-Home trial went live and I had 10Mbit bi-directional fiber with great support from Palo Alto Utilities. Since the trial ended our ISP has been Sonic, first over 3/1Mbit DSL and now over gigabit fiber. So we are really used to having great support from our ISP.
TLG was an astonishing success. From something like $2000/month in December 1992 it grew "an average of 12% per month from Jan94 through July96" when it had "a monthly gross of about $125,000.00 until:
Luckily we were bought by Best Internet Communications, Mountain View; they had money, marketing, and a non-burned-out management; we had a solid locked-in customer base and positive cash flow.Best turned out to be a pretty good ISP too.
Jennings' explanations for TLG's success are interesting. First, technical competence:
Edgar Nielsen almost single-handedly built the technical infrastructure that TLGnet ran on. He designed much of the network and routing structure, all of the security (with some help from Stu Grossman), wrote a complete, queryable, shared and remotely-accessible database (included every single modem, router, wire, cable, customer, IP (domain names and IP address allocations), and logical link) in standard and portable tools, installed equipment, built and maintained our unix boxes, put SNMP on every single node (hundreds) and automated the entire ISP technical infrastructure from one end to the other. I doubt many small to mid-size ISPs today have the things Edgar wrote by 1995.Second, good HR:
Another thing of crucial importance to me, and to Deke, Edgar and a lesser extent Gilmore, was hiring from our local communities; we hired principled people, punk and queer writers and organizers, and trained and paid them -- pay in scale with effort. Total staff turn-over in three years was probably 20; peak staff was 12. Some 10 of them started out at $8.00/hr, unskilled, ended up with $30,000 salary a year later [1994-1996], and stayed in the industry (at prevailing pay). (And we provided health insurance too. Deke being damned Wobbly may have had some small effect.)Third, an innovative business model starting with their terms and conditions:
...
we treated our staff well, gave them credit for work done, paid them actual money, gave raises and bonuses (upon sale of the business, even some fired employees got small bonus checks). TLGnet wouldn't have existed without its talented staff!
TLGnet exercises no control whatsoever over the content of the information passing through TLGnet. You are free to communicate commercial, noncommercial, personal, questionable, obnoxious, annoying, or any other kind of information, misinformation, or disinformation through our service. You are fully responsible for the privacy of, content of, and liability for your own communications.Jennings explains the business model:
The result was:Essentially, other ISPs restricted use and resale of their connections, in a sort of zero-sum approach. By concentrating on bulk connectivity we at once created a market for our customers to provide the vertical services we didn't want or couldn't afford to provide, and built a hard-to-beat solid rep that for a long while locked out direct competitors to our core business; having our prices online and breaking down the leased-line costs and equipment gave us a major one-up economically, technically, and in credible reputation over nearly all other ISPs, big or small.
- Concentrate on bulk, fulltime internet access (leased-line and Frame Relay)
- Keep prices low by providing connectivity only
- Unrestricted use of TLG connectivity
- Encourage resale and vertical-market services
- Full, up-front disclosure of all pricing
- No lock-in contracts
- Unbundle installation costs and eliminate padding
- Full technical disclosure of technical information
Some thought us insane; but in fact our customers didn't "compete" with us, they provided vertical services we couldn't or wouldn't (I guess we did have a business plan). And in fact we set further standards of behavior and policies that other ISPs, including MCI and SprintLink, were obliged to match. Though some, like Alternet and PSI, never did; they skimmed the high-end deep-pockets customers, and we got all the new growth.Gilmore writes:
I would add to the "Busines Model" discussion, that communication costs per-bit dropped dramatically with volume. When you upgraded from 56k bit/sec leased lines to T1 (1,500k bit/sec), you got 24x the bandwidth but it only cost about 4x as much. An upgrade to T3 (45 megabit) provided 30x the bandwidth of a T1, and didn't cost anything near to 30x as much. So, as your traffic volume grew because you were adding more and more customers, the cost of your basic connection to the rest of the Internet got significantly cheaper (per bit). That economy of scale meant that ISPs who grew could keep affording to upgrade their backbones to handle the traffic growth. Every ISP knew, or figured out, this economics, and they all depended on it. Remember, this was back when there were 2000 ISP's in the US, mostly local ones. (About 30 of them were getting their Internet service from TLG when we sold it to Best.)There is a fascinating October 29 1996 interview entitled Tim Pozar and Brewster Kahle CHM Interview by Marc Weber. The first part of the interview is all about TLG. In it Brewster Kahle sums up the story (I cleaned up his stream of conciousness a bit):
it took six months of a full-time person to get us on the DARPA net in 1985 ... but The Little Garden basically made it so that any old person [could connect] and more than that not just themselves but ... enabling other people to create their own ISPs and I don't know there are 400 ISPs now in the Bay Area in large part because of The Little Garden.
#ODDStories 2024 @ Bandung, Indonesia 🇮🇩 / Open Knowledge Foundation
On March 2 to 4 2024, UPI Youthmappers successfully held the Open Data Day 2024 event, with the theme “Bus-friendly: mapping participation guiding blocks & halt for equality of public transportation users and engaging disabled voices”. This event lasted for 3 days with a total of 15 participants.
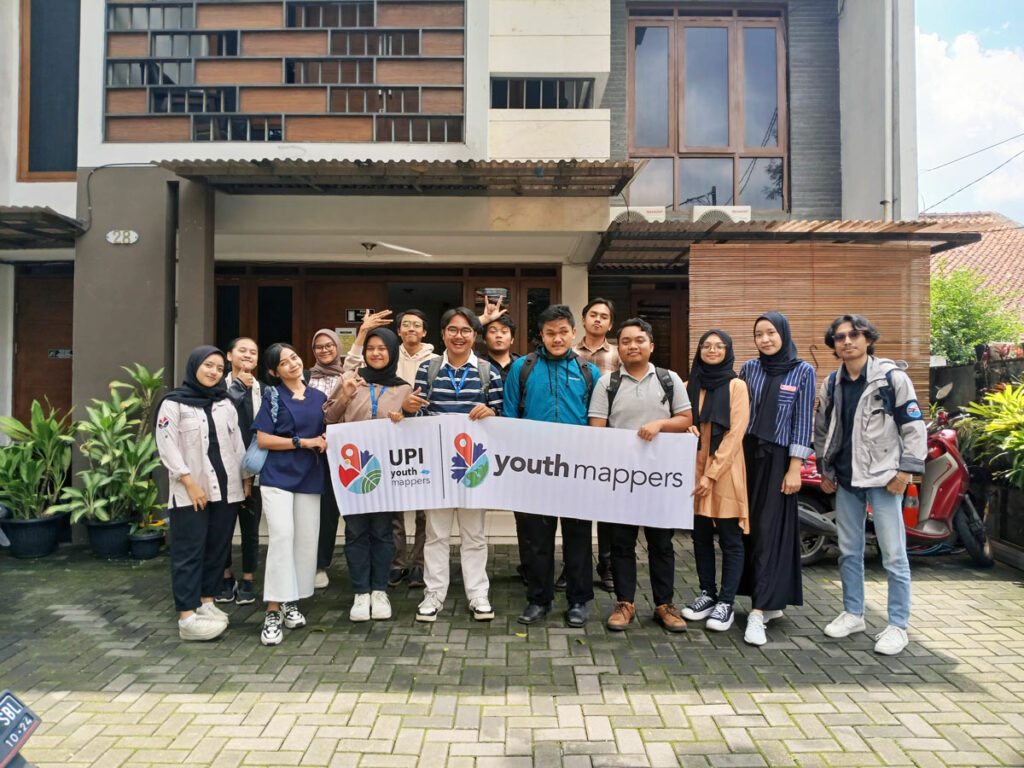
On the first day, UPI Youth Mappers succeeded in making the event a success with a series of activities carried out in the Reka Working Space. First, there was a Hands-on activity using Open Street Maps and Wikipedia. Then presented experienced speakers who shared information regarding “Public Awareness of the Importance of Access for Disabled People in Public Transportation”. Apart from that, UPI Youth Mappers also invited friends who had disabilities, so the sharing session felt more interesting. On the first day, there were also prizes for active participants, but apparently, all participants actively took part in the event on the first day.
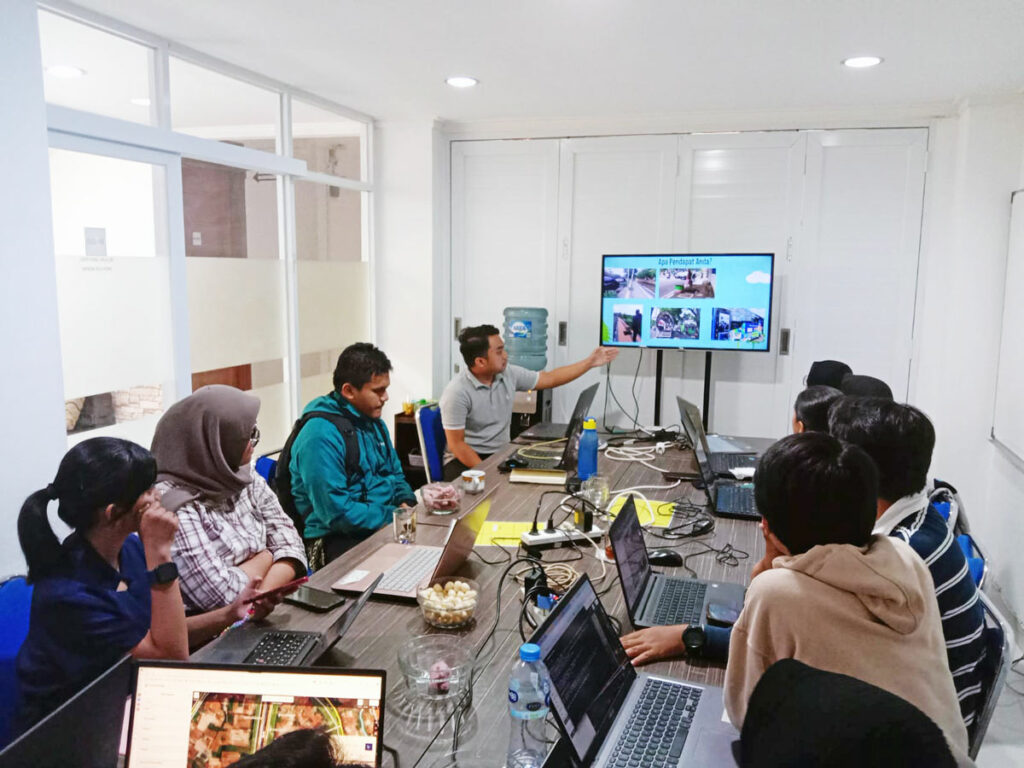
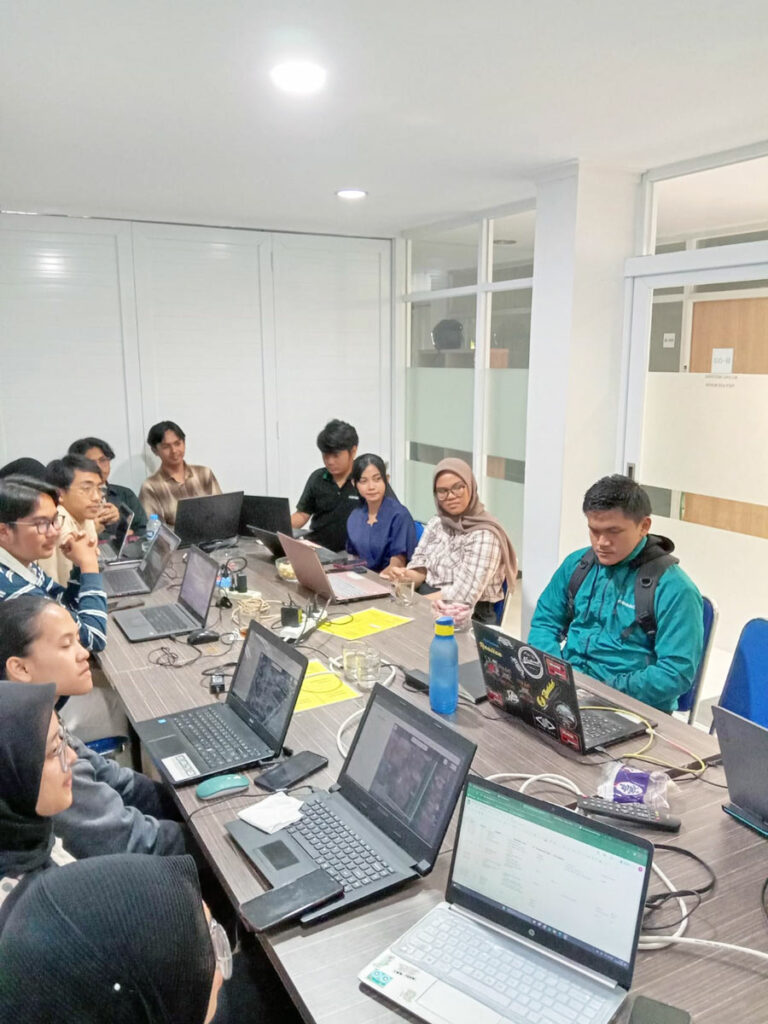
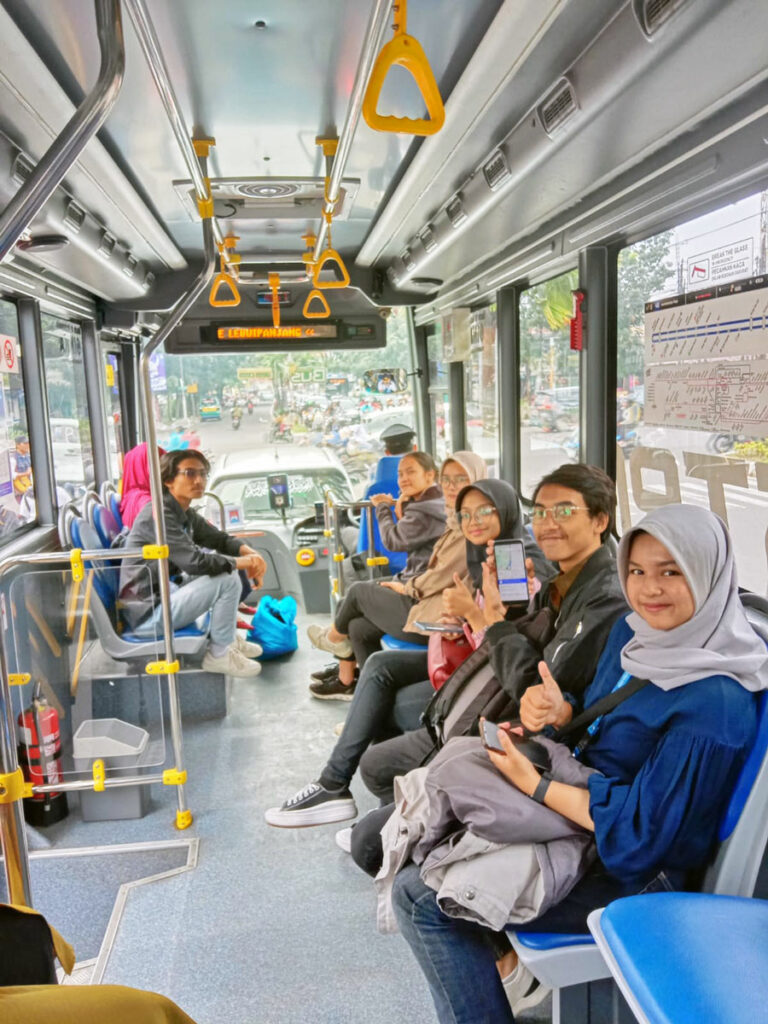
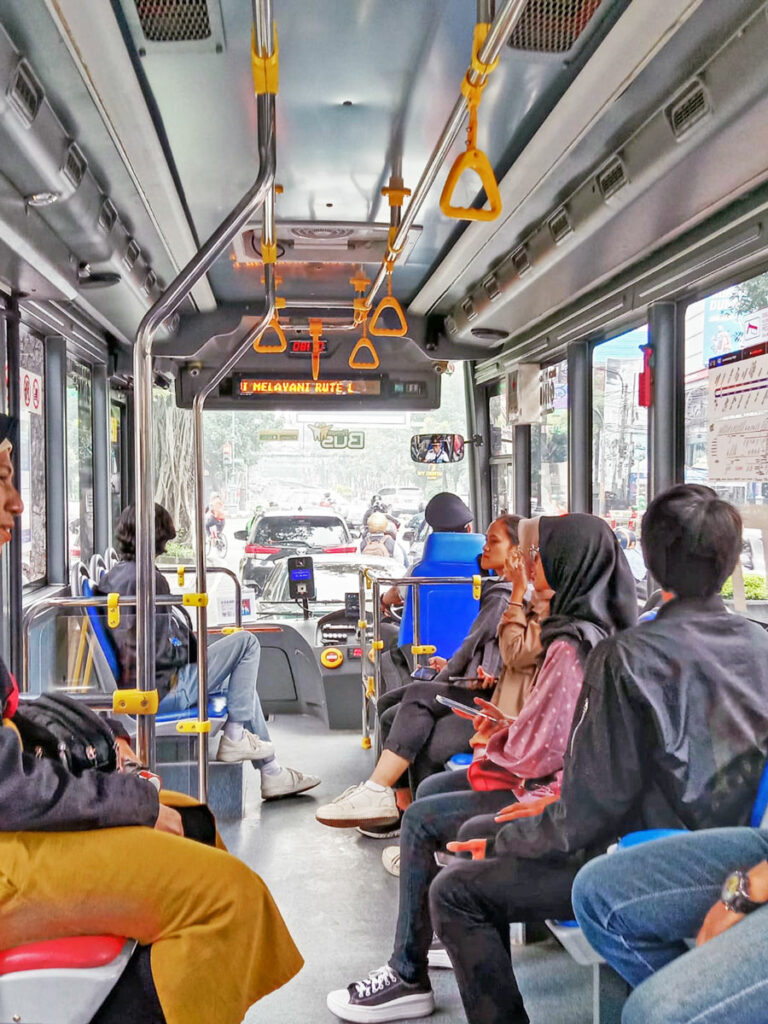
On the second day, it was the day when the participants and committee collected data at every stop in Corridor 4, namely Dago-Leuwi Panjang Terminal, Bandung. This data collection activity was very exciting and all participants succeeded in collecting data to later provide information regarding accessibility for disabled users who use bus transportation, namely Trans Metro Pasundan. The second day’s activities start at 9 am until 2 pm.
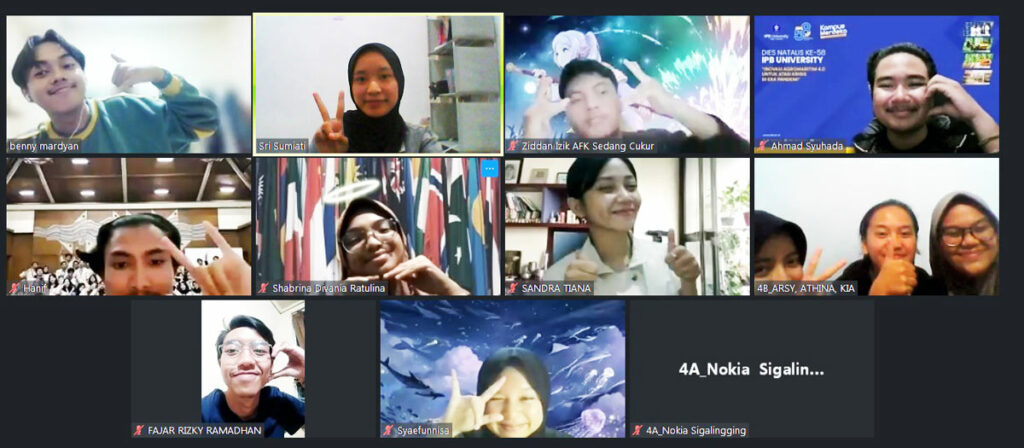
Then the final day was held on March 4 2024 online via Zoom Meeting. The event on the third day was data input into Open Street Maps (OSM) obtained during the field survey. The data entered into OSM is data related to disabled users who use the Pasundan Trans Metro Bus, supplemented with photos of the condition of all bus stops. Each participant inputs data independently in their respective OSM accounts, and is directed by UPI Youth Mappers. The third day of activities closed with the announcement of the most active participants and the distribution of prizes. And with a group photo session.
Our Open Data Day 2024 event was expected to open up knowledge related to our awareness that people with disabilities need to pay attention when using public transportation, namely buses and the availability of good and disability-friendly facilities. By obtaining a number of data and information on OSM, we hope that it will be useful. Thank you for participating in making the 2024 Open Data Day event a success by UPI Youth Mappers!
About Open Data Day
Open Data Day (ODD) is an annual celebration of open data all over the world. Groups from many countries create local events on the day where they will use open data in their communities.
As a way to increase the representation of different cultures, since 2023 we offer the opportunity for organisations to host an Open Data Day event on the best date within a one-week period. In 2024, a total of 287 events happened all over the world between March 2nd-8th, in 60+ countries using 15 different languages.
All outputs are open for everyone to use and re-use.
In 2024, Open Data Day was also a part of the HOT OpenSummit ’23-24 initiative, a creative programme of global event collaborations that leverages experience, passion and connection to drive strong networks and collective action across the humanitarian open mapping movement
For more information, you can reach out to the Open Knowledge Foundation team by emailing opendataday@okfn.org. You can also join the Open Data Day Google Group to ask for advice or share tips and get connected with others.
Private LLMs: Maximize AI Returns, Minimize Data Risks / Lucidworks
Tailor AI solutions with private LLMs to maintain data control and maximize business impact.
The post Private LLMs: Maximize AI Returns, Minimize Data Risks appeared first on Lucidworks.
The Shallows / Ed Summers
I didn’t read Nicholas Carr’s The Shallows when it came out in 2011. I was working as a software engineer at the Library of Congress helping put historical newspapers on the web, and felt indicted by the thesis of the book, which, it seemed to me, boiled down to the idea that the Web was making us stupid. I had built a career around web technology and I wasn’t interested in reading anything that questioned whether the web was a net positive.
Recently, in light of all that’s going on with AI at the moment, and my critical takes on it, I thought perhaps I had dismissed Carr’s book too quickly. Just how long has this project been going on? Did Carr see where things were headed? I mentioned the idea, and a few other people agreed to do a popup bookclub about it.
I’m glad I did get around to reading it finally. I got Carr wrong, he was (is?) a fan of the web, just like I was at the time. But noticing a decline in his ability to focus for long periods prompted him to research and write The Shallows. He draws on the history of the book, media studies and the history of technology more generally to illustrate how technologies like clocks, maps, writing, and the book shaped how we remember and thought itself. In the second half of the book he talks about how the invention of the universal machine (the computer) has effectively absorbed prior forms of media into computers, as hypermedia. I don’t think Carr explicitly mentions the concept of Remediation (Bolter & Grusin, 1996) here, but I thought it was interesting to see how he connects media to the computer’s ability to simulate other machines. He also brings in neurology and psychology research literature to explain how different phases of memory formation are disturbed by rapid attention shifts that browsing the web affords:
The Web provides a convenient and compelling supplement to personal memory, but when we start using the Web as a substitute for personal memory, bypassing the inner processes of consolidation, we risk emptying our minds of their riches. (Carr, 2011, p. 192)
I thought this line of critique was especially interesting in light of the recent popularization of AI in the form of “chatting” with Large Language Models (LLM) like ChatGPT. ChatGPT’s interface provides a smooth surface where you conversationally interact with a computer to obtain information. It doesn’t give you citations, or links to things on the web to consult. Instead it gives you an answer, and you either continue the clarify what you are looking for, move on to something else or decide to stop. You don’t see a list of search results, which you need to click on and move laterally into, to see if they contain the answer to your question. These documents could have distracting design components, ads or other boilerplate. You don’t need to read the linked documents. ChatGPT seductively bypasses all that and you read The Answer. Much to my dismay, it seemed like perhaps the affordances of ChatGPT style interaction may not present the same problems as classic web navigation, at least in terms of distractions that lead to quick context shifts, and disturb our ability to form memories? I imagine there are hordes of education and pyschology researchers looking into this as a type, or they already have.
It was bit surreal reading the detailed descriptions in the final chapters about how neurons store memories through repetitive training … which echo the same language that is used to talk about deep learning today. These are powerful metaphors that have been deployed. Almost anticipating recent developments in AI, Carr ends the book talking about the goals of classic AI, and specifically the warnings of Joseph Weizenbaum in his book Computer Power and Human Reason.
What Makes us most human, Weizenbaum had come to believe, is what is least computable about us–the connections between our mind and our body, the experiences that shape our memory and our thinking, our capacity for emotion and empathy. The great danger we face as we become more intimately involved with our computers–as we come to experience more of our lives through the disembodied symbols flickering across our screens–is that we’ll begin to lose our humanness, to sacrifice the very qualities that separate us from machines. The only way to avoid that fate, Weizenbaum wrote, is to have the self-awareness and the courage to refuse to delegate to computers the most human of our mental activities and intellectual pursuits, particularly “tasks that demand wisdom”. (Carr, 2011, pp. 207–208).
Weizenbaum was the creator the original chatbot Eliza. I didn’t realize that his experience of seeing how ELIZA was used prompted him to critique the goals of Artificial Intelligence community. Maybe it’s not surprising because according to Carr Weizenbaum’s book was trashed by leaders in the computer science community at the time. Perhaps digging up a copy of Weizenbaum’s book might be interesting reading in light of AI’s resurgence now.
The Shallows had lots of citations to current states of affairs, demographics and statistics that gave authority to Carr’s arguments. But these got a little bit repetitive at times, but the drudgery drives the points home I guess. It is striking reading it 13 years later how much the web has changed.
In discussing the technologies of literacy and the book I felt a little bit like Carr’s would have benefited at looking at the book and literacy as instruments of power, that mobilized colonialism and capitalist extraction. I found myself thinking a lot about Bernard Stiegler while reading The Shallows, especially for the idea that writing and computational devices are memory prostheses, and that they are a pharmakon (contain both a remedy and a poison). A quick Kagi search and I can see Stiegler had a sequence of lectures about Carr. So I guess they knew of each other?
Overall I enjoyed The Shallows, even though I’m still working as a web developer. Nowadays I’m explicitly interested in the web’s role in memory practices, and what can be done from an architecture and design perspective to work against the grain of the web’s most pernicious features. There are some good threads to tug on in The Shallows.
References
xz / Ed Summers
If you work in the tech sector you’ve almost certainly already seen the story about the planted security vulnerability discovered in xz. Most of the discussion I’ve seen around this so far has been suggesting that this is a problem that open source software presents, implying that for profit, closed source software is different.
Of course this is yet another reminder of how creators and maintainers of open source software tools are not being supported to continue their work, especially by the companies that use their software. But there’s another important dimension: we really only learned about this story because xz is an open source software project.
Andres Freund is a software engineer who discovered this vulnerability seemingly by chance. He is very humble about it, but his expertise as a software developer primed him to notice the problem and to report it in a responsible way. Are large corporations immune to bad actors planting security vulnerabilities in closed source proprietary software? Nope. In fact, these vulnerabilities are valuable.
The difference is when this happens in closed source software we hear about it as a critical vulnerability after the fact. The exploit is patched and life (hopefully) moves on. But we very rarely hear about how the bug was created, or have any window in on how or why it happened.
The difference with open source software is, well, it’s open. Freund and others like Evan Boehs were able to examine the public Github repository, and try to piece together what happened.
Sadly, Microsoft’s reaction to the news was to remove the GitHub repository from the public web. This means that all this evidence is now hidden from view.
Freund’s email to the oss-security discussion list contained links to the GitHub repository:
Now these are now all broken. However it’s heartening to see that someone had the foresight to archive these using the Internet Archive’s Wayback Machine’s Save Page Now feature:
Intersections of Open Educational Resources and Information Literacy / Journal of Web Librarianship
The Left Curve / David Rosenthal
![]() |
@tzedonn |
There is a surprising amount of respect for people who appear to know nothing about the industry. They’re known as the “left curves.”Below the fold I look at the left side of the curve
The nickname comes from a popular meme in crypto that shows a bell curve with investors on the left who know nothing, or very little, and those in the fat middle of the curve who know something about crypto. On the right are investors who seemingly know everything.
![]() |
Source |
For example, a crypto project with pseudonymous co-founders including “Smokey The Bera” and “Dev Bear” has become a unicorn after it raised millions of dollars from institutional investors such as Brevan Howard Digital. Another token with no real utility — only a cute picture of a dog wearing a hat — has increased by more than 1,400 times its value from three months ago. A developer of a sloth-themed memecoin called Slerf claimed they accidentally burned a large amount of the tokens after raising $10 million.
What a great time to be a left curve! In this bull market, forget about highbrow ideas like revamping Wall Street. Give up on dreams of replacing traditional artwork with nonfungible tokens. Instead, don’t overthink it. Just “choose rich.”
![]() |
Source |
How did this happen? How did crypto’s greatest comeback take place so fast, so hilariously and — at times — so stupidly? Why did crypto evangelists give up their dreams? At its core, it’s because the market is still living under the shadows of past catastrophes like FTX’s collapse and TerraUSD’s blowup.The "past catastrophes like FTX’s collapse and TerraUSD’s blowup" may be old news but to their victims they aren't even close to over. The best FTX's creditors can hope for is to get back what their HODL-ings were worth before Bitcoin took off moon-wards, and who knows when that might happen.
In the past bull markets, when Bitcoin went up, everything else went up amid small-scale rotations between major and small-cap coins. But in this bull market, the rotation is more severe: As Solana went up in the past month, the price of Ether went down dramatically — a simple piece of evidence that shows there’s less money being thrown at the crypto market today than three years ago.
Kevin T. Dugan's The Crypto World Has a New Villain starts with the story of one victim of yet another "past catastrophe":
"I had no idea who Barry Silbert was or anything until after November 16, 2022," Eric Asquith told me. That date was when he was pretty sure he had lost his family’s savings of $1,052,000.Asquith wasn't on the Left Curve but just a bit left of the middle:
He didn’t buy bitcoin or other meme tokens. Instead, earlier that year, he moved over cash from his business — just a little at first, then more — and converted it into digital currencies he thought were as good as cash. The digital coins were called GUSD, and each was worth exactly $1 because the company that minted them — Tyler and Cameron Winklevoss’s crypto exchange, Gemini — backed each one with real money and assets.But Asquith's GUSD were deposited into Gemini's Earn program to get its 5.5% interest, far more than banks were paying. But Asquith and the other Earn depositors were far enough left on the curve that they didn't know Earn wasn't like a bank savings account:
What Asquith did not fully understand was that his money was no longer with Gemini. In one sense, Genesis, a crypto company owned by Barry Silbert had it, but even that wasn’t quite true. Soon-to-collapse hedge funds with names like Three Arrows Capital and Alameda Research — Sam Bankman-Fried’s personal fund — were quietly borrowing from Silbert’s shop. Asquith’s money, and that of tens of thousands of others, was being used by SBF and others to make giant bets on some of the highest-flying, most volatile digital tokens.Then Terra/Luna collapsed and things started to fall apart. Amy Castor and David Gerard reported:
One of Genesis’s biggest customers was Three Arrows Capital (3AC), who they’d lent $2.4 billion. After 3AC blew up in May, DCG assumed $1.2 billion of the liabilities to keep the hit off Genesis’ books. Genesis had been the single largest creditor of 3AC.Gemini tried to get the cash:
Genesis also had money on FTX. As FTX was falling apart, Genesis tweeted on November 8 that they had no exposure, and it was fine. Two days later, Genesis admitted they had “~$175M in locked funds in our FTX trading account,” and they were not fine.
Genesis scrambled to find more capital. Genesis and DCG needed $1 billion in emergency credit by 10 a.m. EST on November 14, but didn’t get it. Even Binance turned them down.
So two days later, Genesis suspended withdrawals,
...
One of Genesis’s biggest customers is Gemini Trust, run by the Winklevoss twins, that operated its own “yield” program, Gemini Earn, for retail investors.
Gemini was supposed to be the safe exchange — but it was exposed to risks via Genesis. There’s now $700 million that Gemini Earn customers can’t withdraw — because it’s stuck on Genesis.
On January 8, Gemini terminated the Master Loan Agreement with Genesis and emailed customers accordingly. This “requires Genesis to return all outstanding assets in the program.” Genesis did not return the funds by the end of January 10 — so they were officially in default on the loan. At this point, Genesis can pull the pin and try to put Gemini into involuntary bankruptcy.They did, and there followed a year of legal wrangling between Gemini, Genesis and Barry Silbert's Digital Currency Group, which owns Genesis. The SEC sued both Gemini and Genesis, and so did the New York Attorney General. While the wrangling continued, another of the semi-regular cryptocurrency bull markets took off until in February 2024:
the victims, Silbert’s now-bankrupt crypto-lending operation, the Winklevoss twins, and regulators hammered out a deal to pay everybody back in full. The crypto bull market of 2024 made it possible to pay back Earn customers not some fraction of what they invested but the generally much higher sum of what their holdings would now be worth.Everyone started cele:wbrating;
Except there was Silbert. Earn victims who had been unfamiliar with him would soon learn that he had made his first fortune by studying the ins and outs of the bankruptcy system and using it to his financial advantage. Since February, the billionaire investor has been relying on a controversial interpretation of bankruptcy law to stop Asquith and all the other victims from getting the bigger payout, the one based on current prices. Instead, to simplify a bit, he would prefer to keep that money himself. “DCG cannot support a plan that not only deprives DCG of its corporate governance rights but also violates United States bankruptcy code,” a spokeswoman for the company said.People started talking to the press about Gemini:
The victims have taken to calling it “the Barry Trade”: If Silbert is successful, he would be able to pocket as much as $1 billion in funds that would otherwise be returned to them. At the very least, Silbert may substantially delay the money being returned to Earn customers.
...
Silbert’s legal logic is that the bankruptcy code sets a date to value victims’ claims in U.S. dollars, and in Genesis’ case, it just happened to be around the market’s lows.
Former Gemini employees told The Beast that Gemini Earn’s terms and conditions were highly dubious from the outset. One staffer recalled reading the fine print for the first time, saying, “[We] were like, ‘Holy shit, are you fucking kidding me?’”And Genesis:
Among those terms: Customer assets were loaned out on “an unsecured basis,” which meant that their money would not be safe in the event of a market collapse. The deposits were also not insured, nor were they guaranteed against errors or fraudulent activity.
“Whatever Gemini may or may not have done pales in comparison to what you see at Genesis, which was more than negligent when it came to protecting customer assets and complying with general best practices,” one former employee said. Among those problems, the person said, was not screening clients who were on, say, the Treasury’s blacklists — an allegation that was supported by a separate January suit filed by New York Department of Financial Services.This isn't a "past catastrophe". For the victims there is no end in sight:
A ruling isn’t expected until April. Since the settlement announcement, the victims have resigned themselves to an even longer wait as Silbert continues to fight. “A year ago, there was a deal that was proposed. Everyone was celebrating in a very similar way,” Asquith said. “Now, I’ll believe it when it’s in my account.”This case, like Dickens' Jarndyce v Jarndyce, will run and run.
Update 4th April 2024
![]() |
Source |
A project describing itself as "The world's first memecoin pre-announced as a rugpull" was explicit in its marketing: "do not buy this coin, as it will go to zero."
Despite that, people sent the creator over 8.8 ETH (almost $29,000) for the project's "pre-sale", even as they repeated on Twitter that the project was a scam and that no one should buy it.
#ODDStories 2024 @ Caracas, Venezuela 🇻🇪 / Open Knowledge Foundation
Between March 5 and 6, 2024, Caracas, capital of the Bolivarian Republic of Venezuela, could not be left behind in the celebration of Open Data Day 2024; and the UTOPIX Femicide Monitor was in charge of it. During these two days a workshop was held: “Bootcamp INFOTOPIA version 2.0: Learning to monitor and infographics gender violence in the Capital District”.
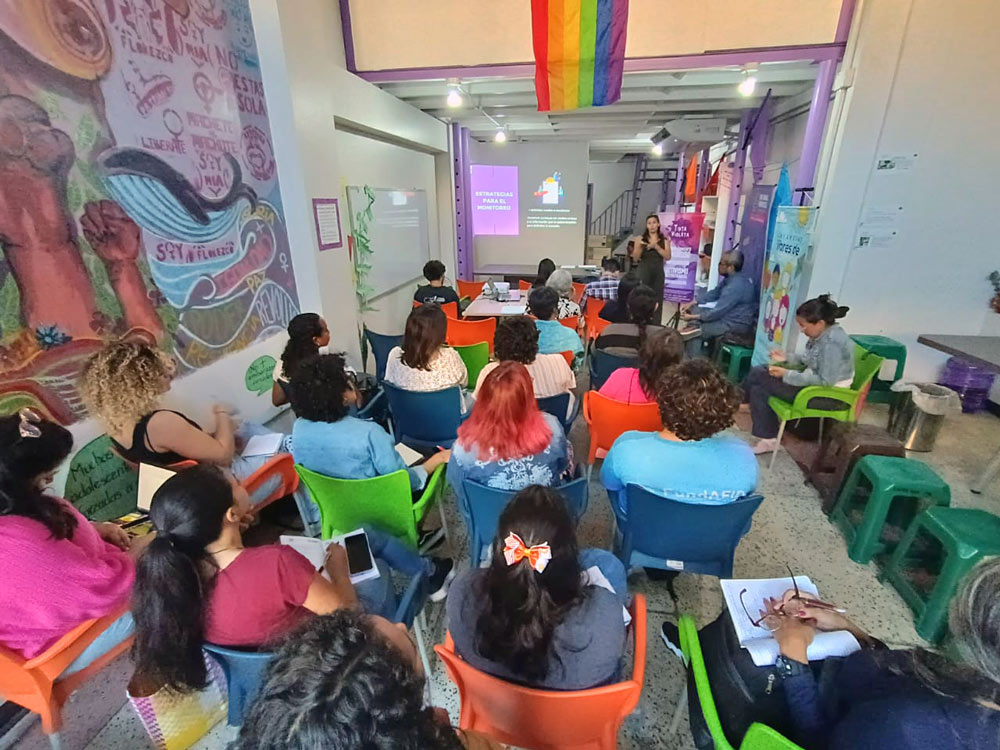
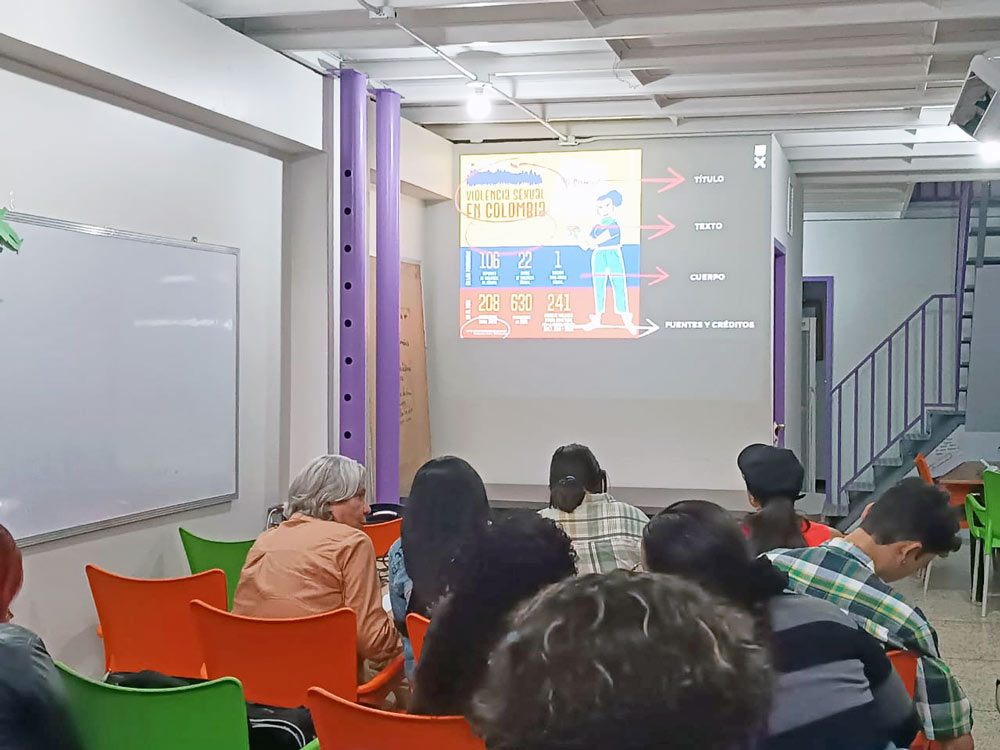
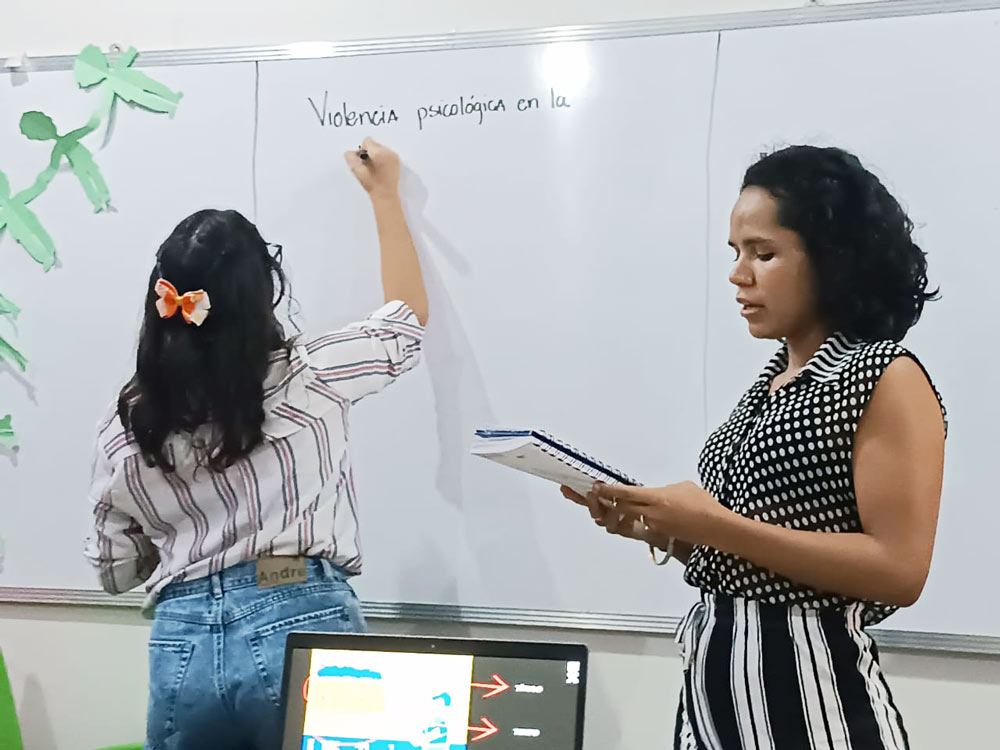
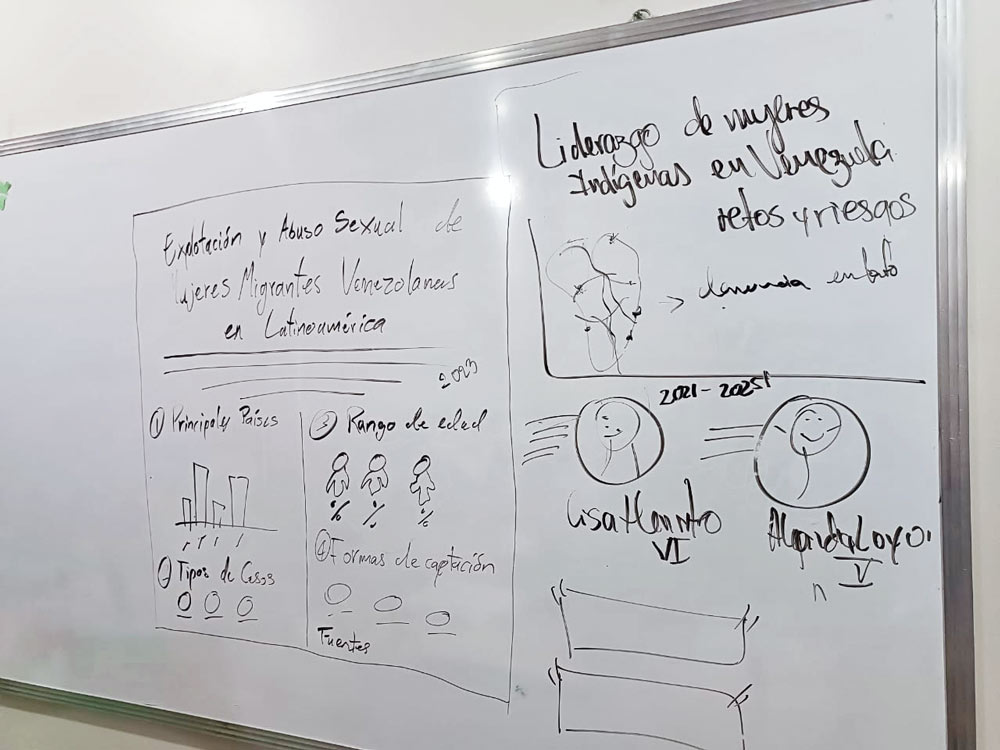
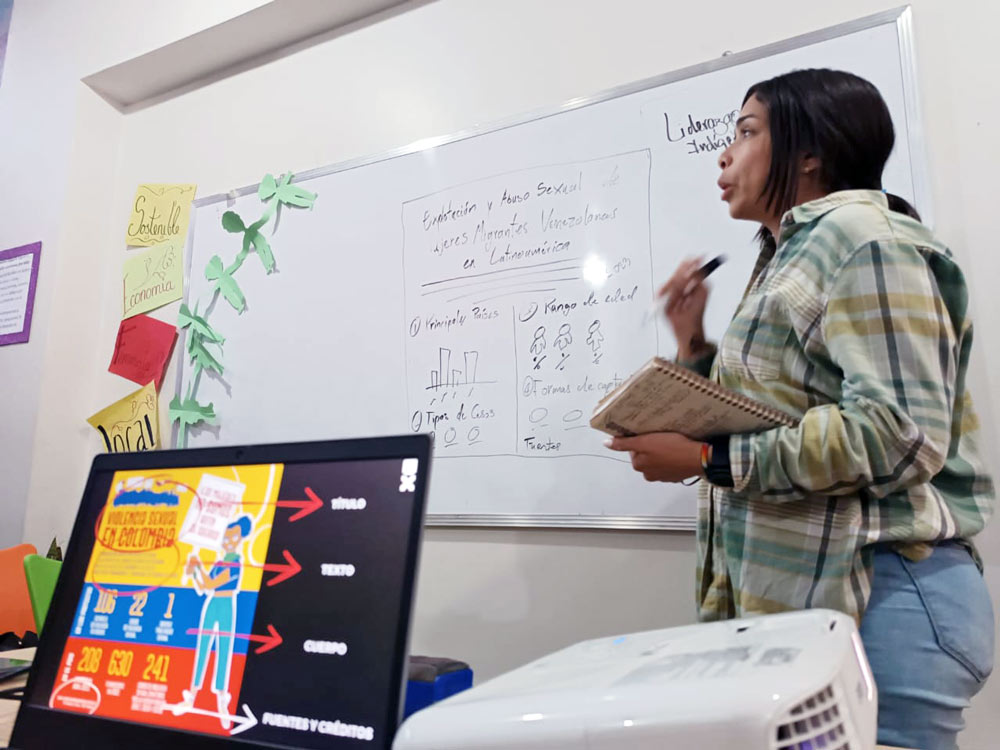
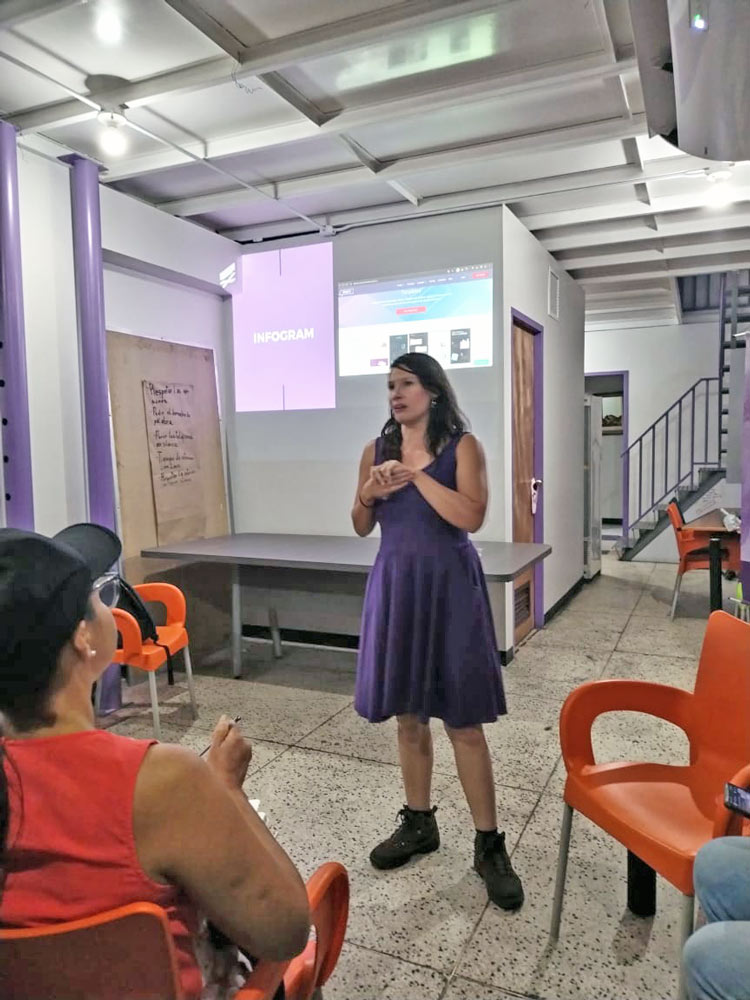
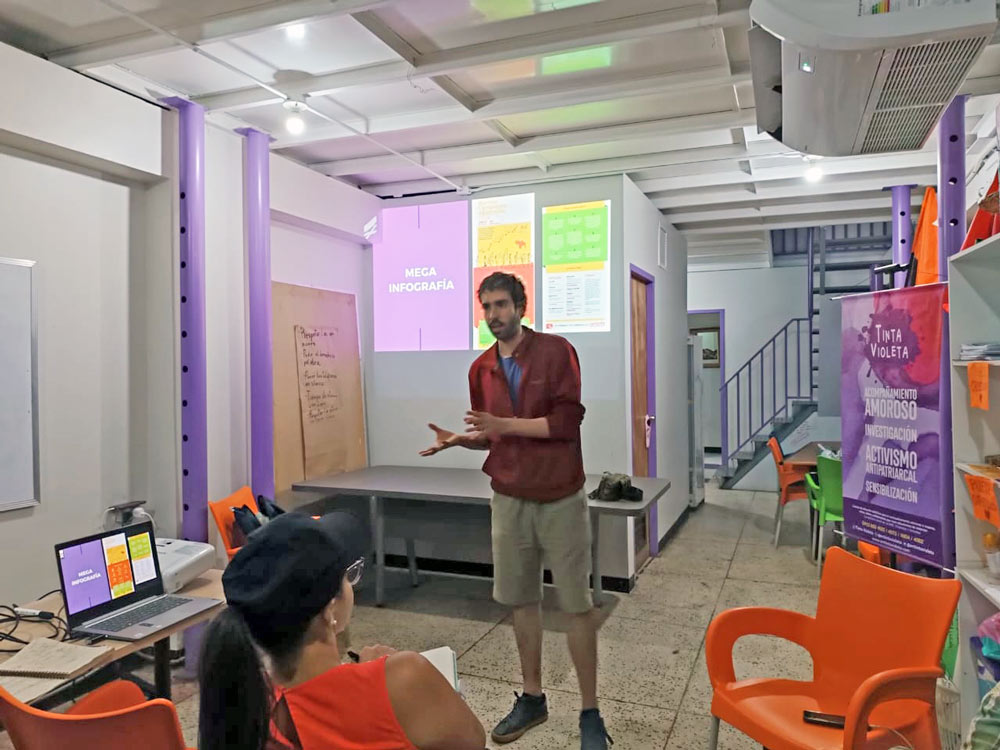
This event was attended by 20 representatives of various feminist and women’s organizations from the states of Distrito Capital, La Guaira, Aragua and Miranda. On the first day, they received an introduction to monitoring data on various types of gender violence through the open source method; and on the second day, they received training on a visual tool for mass dissemination as important as infographics.
The facilitator was myself, Aimee Zambrano, on the first day and on the second day I was accompanied by Kael Abello, both founding members of the UTOPIX community and communication platform.
The main topics addressed during the Bootcamp were:
- Media monitoring and data collection.
- Concepts: the difference between communication and information; information systems;
- Monitoring and follow-up of information;
- Systematization of information;
- Open data analysis: open data and web scrapping;
- Data Monitoring Strategies;
- Some simple tools for data monitoring.
- INFOTOPIA method: it is a collaborative dynamic for the visual processing of information and collective generation of infographic materials.
- Thinking data: a brief review of the fundamental elements of the infographic conception to delimit the criteria, categories and hierarchies of the data.
- From writing to image: A tour of tools that make it possible to infographically display information in a simple way.
Among the main topics addressed by the various organizations as possible projects to be accompanied by the UTOPIX Monitor for their subsequent development into infographics and publication in social networks, the following stand out:
- Women’s autonomy, advances in sexual and reproductive rights.
- Discrimination against Sapphic women in Venezuela during 2023.
- Implicit violence in the experiences of Yukpa indigenous craftswomen and craftsmen.
- Psychological violence in the Altagracia parish: July-December 2023.
- Indigenous women’s leadership in Venezuela, remains and risks.
- Exploitation and sexual abuse of Venezuelan migrant women in Latin America.
The idea is that this Bootcamp is the first of many workshops that we want to dictate in several states of the country, to train various feminist and women’s organizations to start a monitoring network of gender violence in Venezuela.
About Open Data Day
Open Data Day (ODD) is an annual celebration of open data all over the world. Groups from many countries create local events on the day where they will use open data in their communities.
As a way to increase the representation of different cultures, since 2023 we offer the opportunity for organisations to host an Open Data Day event on the best date within a one-week period. In 2024, a total of 287 events happened all over the world between March 2nd-8th, in 60+ countries using 15 different languages.
All outputs are open for everyone to use and re-use.
In 2024, Open Data Day was also a part of the HOT OpenSummit ’23-24 initiative, a creative programme of global event collaborations that leverages experience, passion and connection to drive strong networks and collective action across the humanitarian open mapping movement
For more information, you can reach out to the Open Knowledge Foundation team by emailing opendataday@okfn.org. You can also join the Open Data Day Google Group to ask for advice or share tips and get connected with others.
A modest improvement to WARC-GPT / Harvard Library Innovation Lab
I just made a minor change to WARC-GPT, the tool Matteo Cargnelutti wrote for querying web archives with AI. I’ll explain a little bit of the background, and what the change is for.
What are we trying to do here
The basic idea is that we want to combine web archives with an existing large language model, so that the model will answer questions using the contents of the web archive as well as its inherent knowledge. I have for many years run a wiki for myself and a few friends, which has served variously as social venue, surrogate memory, place to pile up links, storehouse of enthusiasms. When Matteo first announced WARC-GPT, it struck me that the wiki would be a good test; would the tool accurately reflect the content, which I know well? Would it be able to tell me anything surprising? And more prosaically, could I run it on my laptop? Even though the wiki is exposed to the world, and I assume has been crawled by AI companies for inclusion into their models (despite the presence of a restrictive robots.txt), I don’t want to send those companies either the raw material or my queries.
What is a web archive
Briefly, a web archive is a record of all the traffic between a web
browser and a web server, for one or more pages—from which you can
recreate the playback of those pages. My first task was to generate a
list of the 1,116 pages in the wiki, then create an archive using
browsertrix-crawler,
with this
command,
which produced the 40-megabyte file
crawls/collections/wiki/wiki_0.warc.gz
. This is a WARC file, a
standard form of web archive.
Ingest the web archive
We now turn to WARC-GPT; the next step is to ingest the web archive, an optional step is to visualize the resulting data, and the last step is to run the application with which we can ask a question and get an answer.
I installed WARC-GPT by running
git clone https://github.com/harvard-lil/warc-gpt.git cd warc-gpt poetry env use 3.11 poetry install
I copied
.env.example
to .env
and made a couple of changes recommended by Matteo (of which
more later), then copied wiki_0.warc.gz
into the warc/
subdirectory of the repo. The command to process the archive is
poetry run flask ingest
which… took a long time. This is when I started looking at and trying to understand the code, specifically in commands/ingest.py.
What is actually going on here
In the ingest step, WARC-GPT takes the text content of each captured page, splits it into chunks, then uses a sentence transformer to turn the text into embeddings, which are vectors of numbers. It later uses those vectors to pick source material that matches the question, and again later in producing the answer to the question.
This is perhaps the moment to point out that AI terminology can be confusing. As I’ve been discussing all this with Matteo, we continually return to the ideas that the material is opaque, the number of knobs to turn is very large, and the documentation tends to assume a lot of knowledge on the part of the reader.
The first setting Matteo had me change in the .env
file was
VECTOR_SEARCH_SENTENCE_TRANSFORMER_MODEL
, which I changed from
"intfloat/e5-large-v2"
to "BAAI/bge-m3"
. This is one of the knobs
to turn; it’s the model used to create the embeddings. Matteo said, “I
think this new embedding model might be better suited for your
collection…. Main advantage: embeddings encapsulate text chunks up
to 8K tokens.” That is, the vectors can represent longer stretches of
text. (A token is a word or a part of a word, roughly.)
One of the other knobs to turn, of course, is where you run the
ingest process. Matteo has been doing most of his work on lil-vector,
our experimental AI machine, which is made for this kind of work and
is much more performant than a Mac laptop. When I ran an ingest with
BAAI/bge-m3
, the encoding of multi-chunk pages was very slow, and
Matteo pointed out that the parallelization built into the encoding
function must be running up against the limits of my computer. I
turned an additional knob, changing
VECTOR_SEARCH_SENTENCE_TRANSFORMER_DEVICE
from "cpu"
to
"mps"
—this setting is, roughly, what hardware abstraction to use in
the absence of a real GPU, or graphics processing unit, which is where
the work is done on machines like lil-vector—but I didn’t see a big
improvement, so I set out to make the change I
mentioned at the
beginning of this post. The idea is to keep track of encoding times
for one-chunk pages, and if encoding a multi-chunk page takes a
disproportionately long time, stop attempting to encode multiple
chunks in parallel.
This worked; ingest times (on my laptop, for my 1,116-page web archive) went from over an hour to about 38 minutes. Success! But note that I still don’t have a clear picture of how ingest time is really related to all the variables of hardware, settings, and for that matter, what else is happening on the machine. Further improvements might well be possible.
Also note that the pull request contains changes other than those described here: I moved this part of the code into a function, mainly for legibility, and changed some for-loops to list comprehensions, mainly for elegance. I experimented with a few different arrangements, and settled on this one as fastest and clearest, but I have not done a systematic experiment in optimization. I’m currently working on adding a test suite to this codebase, and plan to include in it a way to assess different approaches to encoding.
Coda
You will notice that we have not actually run the web application, nor asked a question of the model.
When Matteo suggested the change to the sentence transformer model, he
added, “But you’ll also want to use a text generation model with a
longer context window, such as:
yarn-mistral“—the point
Matteo is making here that when the sentence transformer encodes the
input in larger pieces, the text generation model should be able to
handle larger pieces of text. The implicit point is that the text
generation model is external to WARC-GPT; the application has to call
out to another service. In this case, where I wanted to keep
everything on my computer, I am running Ollama,
an open-source tool for running large language models, and set
OLLAMA_API_URL
to "http://localhost:11434"
, thereby pointing at my
local instance. (I could also have pointed to an instance of Ollama
running elsewhere, say on lil-vector, or pointed the system with a
different variable to OpenAI or an OpenAI-compatible provider of
models.)
Once Ollama was running, and I’d run the ingest step, I could run
poetry run flask run
and visit the application at http://localhost:5000/. I can pick any of
the models I’ve pulled with Ollama; these vary pretty dramatically in
speed and in quality of response. This is, obviously, another of the
knobs to turn, along with several other settings in the interface. So
far, I’ve had the best luck with mistral:7b-instruct-v0.2-fp16
, a
version of the Mistral model that is optimized for chat
interfaces. (Keep an eye out for models that have been quantized:
model parameters have been changed from floating-point numbers to
integers in order to save space, time, and energy, at some cost in
accuracy. They often have names including q2
, q3
, q4
, etc.) The
question you ask in the interface is yet another knob to turn, as is
the system
prompt
specified in .env
.
I haven’t learned anything earth-shattering from WARC-GPT yet. I was going to leave you with some light-hearted output from the system, maybe a proposal for new wiki page titles that could be used as an outro for a blog post on querying web archives with AI, or a short, amusing paragraph on prompt engineering and the turning of many knobs, but I haven’t come up with a combination of model and prompt that delivers anything fun enough. Stay tuned.
OpenRefine and Wikidata are delicious. So why won’t you try it? / Mita Williams
Advancing IDEAs: Inclusion, Diversity, Equity, Accessibility, 2 April 2024 / HangingTogether
The following post is one in a regular series on issues of Inclusion, Diversity, Equity, and Accessibility, compiled by a team of OCLC contributors.
Webinar on inclusive design in cultural heritage

An 8 April webinar from the Balboa Park Online Collaborative features Dr. Piper Hutson discussing inclusive design, with a focus on sensory processing and neurodiversity. The session’s goal is to empower organizations with the understanding and resources needed to rethink their environments, displays, and activities with inclusivity in mind. Attendees will be introduced to tactics and methods to incorporate neurodiversity-friendly approaches at their institutions, including: understanding the diverse ways people interact with cultural settings; utilizing technology to bolster accessibility; and cultivating an environment of organizational empathy and comprehension.
Museums have been working towards creating spaces and experiences that are more friendly to neurodiverse people. These efforts can offer many lessons to libraries and archives that are pursuing similar goals. Plus, this is a teaser for ALA 2024, when we can meet in person to take in all Balboa Park has to offer! Contributed by Richard J. Urban.
Metadata training materials for DEIA
Like many organizations concerned with issues of Diversity, Equity, Inclusion, and Accessibility, the Music Library Association (MLA) has required members who participate in any part of its administrative structure to keep current with training on implicit bias, anti-racism, and other aspects of cultural competency. MLA’s Cataloging and Metadata Committee (CMC) has compiled a list of recent presentations, webinars, best practices, and other publications that both fulfill MLA’s directive and are of more general interest. “Metadata-Focused DEIA Training” includes links to programming presented at past MLA and other professional conferences and OCLC Cataloging Community Meetings. Several of the recordings also include lists and links to additional related material.
Although the focus of this list is, naturally, on metadata, these freely-available online resources range far beyond cataloging, let alone just music cataloging. Ethical and inclusive description of library materials stands at the center of so much of what libraries do. There is so much work that needs to be done to repair outdated terminology and practices, that an awareness by everyone in the library ecosystem strengthens the institution at large. Contributed by Jay Weitz.
The post Advancing IDEAs: Inclusion, Diversity, Equity, Accessibility, 2 April 2024 appeared first on Hanging Together.
Autistic Students and Academic Library Research: Recommendations for a Neurodiversity-Informed Approach / In the Library, With the Lead Pipe
In Brief: Despite a growing pool of research in library and information science (LIS) authored by autistic librarians (see Lawrence, 2013; Tumlin, 2019), the vast majority of LIS research about autistic students in academic libraries continues to portray autism as a tragedy that students must overcome, a common trope that the autistic community has long rallied against (Sinclair, 1993). In this article, I recommend that those writing about autistic students and academic libraries do so through a neurodiversity lens; those who ascribe to the neurodiversity paradigm generally conceive of variation in neurocognitive functioning as a valuable and natural aspect of human diversity (Walker, 2021). Making this paradigm shift in name alone, however, will not be enough. Effecting true change in LIS research will involve deep reflection on the values and norms of academia as well as those of librarianship. As a profession concerned chiefly with information, and as academic librarians tasked with supporting college students of all neurotypes during their formative years, we must begin to interrogate the ways in which our research and professional practices may themselves create barriers to the inclusion and full participation of autistic students in our libraries.
Due to increased transition supports, greater access to diagnostic services, and increased knowledge about how autism presents in women, people of color, and other marginalized groups, a greater number of autistic students are attending higher education institutions (Bakker et al., 2019). Though autism was once inaccurately conceived of as a childhood phenomenon that a person could outgrow, it is now more accurately understood as a distinct neurotype associated with a diverse range of support needs and strengths that may vary for individuals across contexts and time. Autism-focused research in library and information science (LIS) has become increasingly common in recent years, and academic librarians have begun to address the needs of this growing population of autistic students in higher education through both research and practice (Anderson, 2018; Anderson, 2021; Robinson & Anderson, 2020; Pionke et al., 2019; Remy et al., 2014).
Yet despite increased postsecondary enrollment, autistic students still experience myriad barriers to academic success and graduation, many of which stem from systemic ableism within higher education and society more broadly. Academic libraries have long been recognized as a key resource for college student success, and autistic students in particular may value the library as a place of escape and refuge within the unstructured and overstimulating college environment (Anderson, 2018). With the understanding that academic libraries may be of particular help in supporting autistic students’ success, an increasing number of autism-focused articles have appeared in LIS journals within recent years. Despite the good intentions undoubtedly underlying this line of research, much of what has been written about autistic students in academic libraries serves to perpetuate the same inaccurate and harmful stereotypes that appear in autism research across other disciplines (Botha & Cage, 2022). One potential solution to this ongoing problem is the adoption of the neurodiversity paradigm within library and information science research.
What is the Neurodiversity Paradigm?
Autistic scholar Nick Walker defines the neurodiversity paradigm as a perspective that appreciates neurodiversity as a “natural and valuable form of human diversity,” rejects the socially constructed notion that there is a “normal” and correct kind of “neurocognitive functioning,” and recognizes that “social dynamics” and structural inequities related to neurodiversity are similar to those experienced by other marginalized groups (2021, p. 36). E.E. Lawrence, an autistic LIS scholar, called for the adoption of the neurodiversity paradigm in LIS research and practice in his 2013 essay “Loud Hands in the Library: Neurodiversity in LIS Theory & Practice.” To my knowledge, Lawrence is the first LIS scholar to problematize the way in which research by and for library professionals has failed to adopt the neurodiversity paradigm, resulting in LIS research that dehumanizes and pathologizes autistic people. In his essay, Lawrence argues that librarians have “a special obligation to generate theory, policy, and practice that is consistent with neurodiversity” (2013, p. 99). While Lawrence notes that library professionals may view this call for adopting the neurodiversity paradigm as a violation of librarians’ supposed professional neutrality, he adamantly disagrees, arguing that embracing and affirming neurodiversity is essential to our ability to provide equitable and effective library services to all patrons.
In what follows, I argue that despite multiple calls from autistic LIS professionals like Lawrence (2013), the vast majority of researchers in the field have failed to truly adopt the neurodiversity paradigm. With a focus on literature published since 2013, I explore the ways in which academic librarians have written about autistic people, highlighting examples that are in tension with the neurodiversity paradigm and those that suggest progress toward affirming neurodiversity in our research and practice. In particular, I problematize the paradoxical nature of research that purports to better support a patron population while simultaneously dehumanizing those same people. Finally, I contend that this paradigm shift within our profession would necessitate a reckoning with our espoused values and our behavior as researchers and practitioners, especially in relation to evidence, authority, and credibility.
Reviewing the Literature
The majority of publications regarding autistic students in academic libraries within the last ten years have addressed the need for increased staff training, the adoption of universal design, and the creation of specific programs to support autistic students (Anderson, 2018; Anderson, 2021; Boyer & El Chidiac, 2023; Layden et al., 2021; Pionke et al., 2019; Remy et al., 2014; Robinson & Anderson, 2022; Walton & McMullin, 2021). Many conceptual pieces describe the support needs of autistic students, typically drawing upon autism research from medicine, psychology, and education, and applying findings from this body of research to recommend approaches to academic library services (Carey, 2020; Cho, 2018; Everhart & Anderson, 2020; Shea & Derry, 2019). Despite the fact that this research is undoubtedly rooted in the goal of helping library staff to better support autistic students, much of the recent LIS scholarship about autistic college students continues to reinforce deficit-based views about autism, in part due to the (sometimes unacknowledged) use of the medical model of disability as a lens.
Models of Disability
At present, there is no clear consensus within the neurodiversity community, or the disability community at large, regarding a universally preferred model of disability. The various models of disability each conceptualize disability and its origins differently, with the medical and social models often conceived of as being particularly at odds. While the medical model defines disability as an individual phenomenon, where disabled people are inherently abnormal, deficient, and in need of remediation (Botha & Cage, 2022), the social model identifies societal barriers and a lack of accommodations as the factors that render people disabled (Shakespeare, 2013). Critics of the medical model cite its focus on fixing the individual as problematic, while critics of the social model argue that a primary focus on societal barriers overshadows the reality of impairments and the challenges that may arise from them. When it comes to embracing the neurodiversity paradigm, however, Walker (2021) claims that the medical model of disability is incompatible, and she argues for the use of the social model by those who adopt a neurodiversity lens for their research and practice. Though I recognize the potential shortcomings of the social model for some disability experiences, I find Walker’s argument convincing in the context of neurodiversity and autism, which I believe to be natural and neutral examples of human diversity.
The description of autistic college students through a medical model lens in LIS research is a common and problematic practice, but what is especially troubling about this practice is that many authors whose work aligns with the medical model claim to be proponents of the social model. Several authors of recent LIS publications about autistic college students describe different models of disability (Remy et al., 2014; Robinson & Anderson, 2022), or even recommend the adoption of the social model in future studies (Shea & Derry, 2019). Still, the majority of researchers continue to describe the challenge autistic students face as arising from their autistic traits, rather than from the ways in which institutions of higher education are designed for neurotypical students, who are also frequently inadequately supported by our systems.
One of the often-cited barriers to academic success for autistic students is the fact that many disabled students, including autistic students, choose not to disclose their disability to their college or university (Cho, 2018; Everhart & Escobar, 2018; Remy et al., 2014). While lack of disclosure and a corresponding lack of support services are noted in these works, few, if any, acknowledge the systemic issues that would lead a disabled person to avoid disclosing their diagnoses and support needs. There is a wealth of research regarding the discrimination disabled students face when attempting to navigate the academic accommodations process, including social stigma, disbelieving faculty, and stringent documentation requirements (Lindsay et al., 2018) that these LIS publications do not acknowledge. Instead, some researchers have attributed an avoidance of disclosure as a hesitance to be “classified by their disability and labeled ‘disabled’” (Everhart & Escobar, 2018, p. 270) or “receive undue attention” (Remy et al., 2014) without any discussion of the social contexts, systems, and individual behaviors that create an environment in which the disability label becomes problematic.
This same framing is often used to describe the higher rates of attrition among autistic students in higher education as compared to neurotypical peers. For example, Robinson and Anderson (2022) cite a “number of factors that contribute to this attrition, including difficulty transitioning to higher education, mental health difficulties, and low socioeconomic status” (p. 164). The authors go on to discuss common challenges autistic students face, including struggles with time management and self-advocacy, noting that these students “consequently…have a graduation rate that is significantly lower than neurotypical students” (Robinson & Anderson, 2022, p. 161). While these statements are not objectively false, and autistic students do often struggle with executive functioning, self-advocacy, and mental health, the manner in which these challenges are framed does not contextualize autistic students’ struggles as occurring within a larger social and academic context that is designed with nondisabled students in mind.
These rhetorical choices exist in direct tension with the authors’ description of the social and medical models of disability, as well as their recommendation that the neurodiversity framework could be useful in explaining challenges neurodivergent students face in higher education. This tension is present in a number of publications, many of which advocate for greater staff training about the needs of disabled patrons (Anderson, 2018; Remy et al., 2014) while still framing the challenges autistic students face as existing primarily due to their autism, rather than arising from a mismatch between expectations and supports, or an interaction between impairment and context.
In adopting a neurodiversity lens, I recommend that LIS scholars thoughtfully examine the theoretical underpinnings of the ways in which they explain the challenges autistic students face in higher education. While the social model may not be appropriate framing for the experiences of every disabled person, I agree with Walker (2021) that the social model is the most appropriate option for neurodiversity-informed research. The social model does not deny the existence of impairment or the need for supports, as some critics suggest, but it does distinguish between an impairment, such as low vision, and the disablement that comes from not being able to participate fully in society due to systemic barriers (Shakespeare, 2013). Adopting the neurodiversity paradigm, which engages with the constructed nature of disability alongside the reality of individual impairment, will mean finding ways to describe needed supports without dehumanizing those who would benefit from them. This shift will require making less harmful and more accurate choices regarding the language used to describe autistic people in LIS research.
The Impact of Language
An unfortunate consequence of the medical model lens used in much of autism research in LIS is that much of the language used to describe autistic people and their traits is dehumanizing and pathologizing in nature. Among the most jarring inconsistencies is the use of the term “deficit” in articles that claim to be aligned with the social model of disability, which primarily understands disability is a socially constructed phenomenon arising from barriers to full participation by disabled people (Lawrence, 2013). By claiming that autistic people are deficient in certain skills or traits, a comparison is made to an unspoken, undefined norm, casting autistic people as inherently inferior and abnormal. Some authors even go so far as to claim that autistic students face challenges in higher education and academic libraries “because of these deficits” (Shea & Derry, 2019, p. 326, emphasis added), which is in alignment with a medical model understanding of autistic students’ experiences.
The choice to use such pathologizing language is often defended as the expectation for scientific research. Cho (2018), for example, notes that their work is grounded in “the scientific understanding of ASD as a disorder” (p. 329). In their recommendations for avoiding ableist language in autism research, Bottema-Beutel and colleagues (2021; 2023) note that researchers often justify deficit-based language choices as scientific, citing concerns that less ableist terminology could be construed as inaccurate and unscientific. Despite this reasoning, many authors utilize deficit-based phrasing that in itself is vague and often inaccurate, including functioning labels. Functioning labels are a hotly debated issue in autism research, though “few autistic adults, family members/friends, or health care professionals endorse the use of functioning-level descriptors” (Bottema-Beutel et al., 2021, p. 23). While many of the articles cited here use the term “high-functioning” to describe autistic college students (Anderson, 2018; Cho, 2018; Everhart & Anderson, 2022; Everhart & Escobar, 2018; Remy et al., 2014), few actually articulate what this means within the context of their research or for the autistic students their readers will support in their own libraries. This is not a LIS research-specific phenomenon; functioning labels tend to be extremely vague across autism research in a variety of disciplines, and often serve only to “assign expectations,” reducing autistic “people to ‘human doings’ instead of human beings” (Tumlin, 2019, p. 13). Despite the dehumanizing nature of these language choices, authors of these same publications often insist on using person-first language to describe autistic people.
Though person-first language (i.e. person with autism) was once touted as the gold standard in special education, it has now come to be understood as less universally preferred among the highly heterogeneous disability community (Bottema-Beutel et al., 2021). Many autistic people, in particular, have come to prefer identity-first language (i.e. autistic person), recognizing autism as a key part of their identity, inseparable from their selfhood (Sinclair, 2013). Proponents of person-first language insist that acknowledging autistic people’s personhood is paramount, but autistic scholars have noted that the need to be reminded that autistic people are human beings, as if it is not automatically apparent, is dehumanizing in itself (Sinclair, 2013). Despite a critical mass of the autistic community advocating for a shift in language to affirm and validate autistic identities, research has lagged behind, overwhelmingly tending to favor person-first language, often in combination with the word “disorder” (Bottema-Beutel et al., 2021; Lawrence, 2013; Sinclair, 2013).
Interestingly, multiple LIS scholars have acknowledged a preference in the autistic community for identity-first language, but explicitly choose to align their own language use with mainstream autism research (e.g. Anderson, 2018; Shea & Derry, 2019). Even more troubling, some authors who use person-first language throughout their text also refer to autistic people as “ASDs,” an odd term based on the initialism for Autism Spectrum Disorder that feels uniquely dehumanizing among the many possible language choices at hand (e.g. Braumberger, 2021; Remy et al., 2014; Robinson & Anderson, 2022).
The language used in these publications reflect the ongoing lack of engagement with the neurodiversity paradigm in our field, despite the occasional references to neurodiversity-inspired terminology in this same research. Terms developed by neurodiversity scholars appear occasionally in LIS research focused on autistic students in academic libraries, but few of these publications use the language consistently, accurately, and in a way that reflects the values of neurodiversity advocates. Autistic scholar Nick Walker (2021) describes a common pitfall regarding neurodiversity and language: using “neurodiverse” and “neurodivergent” interchangeably. While neurodiversity refers to the diversity of human minds and cognition, neurodivergence refers to those who diverge from the socially constructed neurotypical norm, including autistic people and those with ADHD, dyslexia, etc. (Walker, 2021). Others misuse the term neurotypical, generally understood to be those who are able to align with the socially constructed cognitive norm (Walker, 2021), claiming it to refer to allistic, or non-autistic, people (Everhart & Escobar, 2018) or failing to describe the ways in which ‘typical’ is a non-objective social construct (Walton & McMullin, 2021).
These misrepresentations, whether intentional or not, suggest that many LIS scholars have not engaged thoughtfully with the work of neurodiversity scholars. This is especially troubling when library researchers cite the work of the few known autistic LIS scholars, whose work calling for neurodiversity-informed LIS research is quite clear in its language and recommendations (Lawrence, 2013; Tumlin, 2019). Though some LIS scholars have indicated that they are aligning their language with professional and scientific standards, the language these authors often use is often vague and inaccurate (Bottema-Beutel et al., 2021) and “good science does not require derogatory language or dehumanization of autistic people” (Bernard et al., 2023, p. 683).
The neurodiversity framework conceives of the diversity of human cognition as natural, and even valuable, rather than as a deviation from a more desirable, “normal” brain. The adoption of such a mindset would require more precise language choices, particularly when the terminology we employ is linked to issues of power and positioning. For example, LIS researchers must acknowledge that “neurodiverse” is not a euphemism for “neurodivergent,” a term that does not mean abnormal, but a deviation from the socially constructed norm for human cognition (Walker, 2021). Explicit recognition of the ways in which unspoken, undefined (and often undefinable) norms are used to Other marginalized groups, including neurodivergent people, is a key feature of neurodiversity-informed research. Language choices that pathologize certain ways of perceiving, thinking, and being in the world are incompatible with this framework.
It is imperative that the LIS field recognize the powerful nature of the language choices we make, both for the understanding of colleagues we hope to share our research with, and for the well-being autistic people (both patrons and colleagues) who come into contact with our work. Deficit framings of autistic people contribute to the ongoing marginalization of a group whose voices are already woefully underrepresented in research, and contribute to power dynamics in the field that prevent meaningful engagement with autistic collaborators and participants (Bernard et al., 2023), an essential feature of neurodiversity-informed LIS research.
Representation of Autistic Voices
Though some authors have cited the work of autistic LIS scholars Lawrence (2013) and Tumlin (2019) in their own work pertaining to autistic patrons in libraries, few have adopted the neurodiversity framework that both authors call for, and even fewer engage with autistic participants as part of their research. In one of the few LIS publications to report on findings from autistic participants, Pionke and colleagues (2019) note that much of the library literature published about autism does not actually draw upon the autistic experience. Other authors note that if the neurodiversity movement is to make headway in the field of library and information science, LIS scholars will need to interact with neurodivergent patrons to conduct their research, “as it is important not to speak for a population without gathering their input” (Everhart & Anderson, 2020, p. 4).
Several LIS studies within the past ten years, including the work of Pionke and colleagues (2019), have drawn upon autistic participants’ perspectives and offer examples of how neurodiversity-informed research might amplify autistic voices. In their 2019 study, Pionke and colleagues engaged directly with autistic students in their analysis of library support for Project STEP, a program for autistic college students. Anderson’s (2018) study of online communication among autistic students regarding their use of academic libraries, a publication from her dissertation data, is one of the first to acknowledge the importance of autistic people’s lived experiences and articulated preferences regarding library resources, spaces, and services. Were more LIS researchers to engage with the autistic students on their own campuses, there would likely be a greater number of studies that challenge outdated and harmful assumptions about autistic people, leading to more relevant and useful findings that will allow us to better serve our patrons.
A 2020 study of disabled students’ perceptions of academic library accessibility webpages (Brunskill, 2020), though not focused solely on autistic participants, is a prime example of the ways in which a researcher might not only engage with disabled participants, but also intentionally design a neurodiversity-informed study. Brunskill collaborated with disability studies faculty, including disabled faculty members, to design their interview protocol in a way that would avoid causing harm to their participants. Following a pilot of the interview protocol, Brunskill offered students control over the interview modality, a choice that not only aids in transferring some power to participants, but also reflects a neurodiversity- and disability-affirming approach, as different modalities may meet different participants’ needs and preferences.
Epistemic Injustice
While the issue of little engagement with autistic participants and colleagues in scholarship is not unique to our field, it is still troubling, and reflects a larger trend of failing to prioritize the voices of the marginalized groups we aim to support. Autistic scholar Remy Yergeau (2018) describes the way in which the narrative about autism and autistic people is one of non-agency and non-rhetoricity; autistic people are constructed as non-actors in their own lives, and non-speakers about their own experiences. Those who attempt to speak about the expertise derived from their lived experience are silenced on the basis of being either too autistic or not autistic enough to speak for the autistic community (Botha, 2021), a strategic and effective dismissal experienced by members of many marginalized groups. By prioritizing the preferences and voices of so-called autism ‘experts’ in LIS scholarship, we not only perpetuate harmful stereotypes about the people we seek to serve, but also discount the experts who could make our research and practice significantly more effective.
Autistic scholar Monique Botha (2021) describes the ways in which autism research routinely causes harm through such characterizations, which reinforce the dehumanizing portrayals of autistic people while ignoring very real evidence from autistic participants and scholars. Botha calls this “epistemic injustice,” a phenomenon in which autistic voices are continuously silenced and marginalized in favor of supposedly neutral and scientific research (p. 6). In short, “we do not trust, nor want autistic people to talk about autism” (Botha, 2021, p. 6). Until we, as a profession, begin to meaningfully collaborate with autistic students in our research, we will continue to perpetuate and affirm this harmful message.
This epistemic injustice also helps to explain why LIS scholars are not engaging with the work of autistic scholars to the degree that they engage with mainstream autism research. Several LIS studies mention, for example, that autistic students may struggle in social situations due to deficits in social communication skills (Anderson, 2018; Everhart & Escobar, 2018; Shea & Derry, 2019). These characterizations of communication challenges arising from autism alone disregard the well-known work of autistic scholar Damian Milton (2012), who coined the term the “double empathy problem” to describe the interactional communication challenges between autistic and non-autistic interlocutors, arising from a communication mismatch rather than from autistic deficits. Crompton and colleagues (2020) confirmed through experimental research that autistic individuals communicate effectively with one another, lending further credence to Milton’s theory of the double empathy problem.
Milton’s work, alongside the work of other autistic autism researchers, is often uncited in LIS publications, which tend to favor more traditional and conservative notions of expertise and authority. While these choices may seem neutral and aligned with science, as many claim them to be, I argue that no choice is apolitical, especially when scholars hold the power to contribute to the problematic positioning of a marginalized group they believe their research supports. To begin the journey toward adopting a more humanizing, neurodiversity-informed approach to LIS research, we must first ask ourselves why this change has not already been made.
A Professional Reckoning
Ten years after Lawrence’s (2013) “Loud Hands,” a key question remains: why have academic librarians failed to heed our colleagues’ calls to adopt the neurodiversity paradigm as a framework for our research about autistic students? I contend that this ignorance, and in some cases, outright refusal, to engage with neurodiversity is in direct tension with our espoused professional values and the information literacy dispositions we aim to cultivate in our students. This issue also reflects a larger tension within our profession regarding who we deem to be an authority, and whether we are willing to critically engage with the ways in which power and systemic oppression impact who is perceived as authoritative.
As academic librarians, we regularly teach students that “authority is constructed and contextual” (Association of College and Research Libraries (ACRL), 2015), and that credibility, evidence, and authorship are not always to be taken at face value. Though we employ the ACRL Framework (2015) to foster a critical stance toward authority in our students, thus far, our field has failed to take this same stance in our own research about autistic students in academic libraries. Instead, the majority of this research perpetuates the notion that autistic people are, at best, abnormal and in need of remediation, and at worst, inhuman and non-rhetorical (Yergeau, 2018), unable to serve as experts on our own experience.
Andrea Baer (2023) recently problematized the ways in which the LIS field has uncritically accepted the “dominant narrative” of a post-COVID world, a narrative that is contradicted by reputable data about disease transmission. She notes how the rhetoric surrounding the ongoing death toll of COVID has been described as occurring predominantly in the elderly and people with pre-existing conditions, and those who continue to take precautions to protect themselves and others are viewed as illogically, even pathologically, anxious (Baer, 2023). The lack of critical information literacy demonstrated by many in our own profession regarding COVID-related information is also apparent in the way that we engage with information about autistic people, and I second Baer’s call for information professionals to consider the impact of power and privilege in relation to information literacy.
Fully adopting the neurodiversity paradigm would require that we examine our implicit biases about who is permitted to be an authority on a subject, and how structural inequities have positioned non-autistic experts and their often deficit-based scholarship as the ultimate authority on autistic people. As educators, we have helped students develop an understanding of how issues of power and privilege impact supposedly authoritative information sources in other fields, such as when we note that the majority of medical textbooks have long pictured dermatological conditions on only white skin, significantly impacting physician knowledge and health outcomes for people of color. Just as systemic racism has led to white bodies historically being constructed as the default in medical information, systemic ableism has portrayed non-autistic ways of being as normal, therefore casting autistic and other neurodivergent people in the role of the deviant Other.
It is particularly concerning, and not in alignment with our professional values, that LIS research about autistic college students fails to critically examine the way autistic people have been positioned and continue to be positioned in scholarship, including our own. Embracing the neurodiversity framework would mean addressing our implicit biases about who is a reliable information source about autism, and acknowledging that the mainstream storying of autistic lives has been constructed by professionals with deficit views, marginalizing the voices of autistic participants and scholars (Yergeau, 2018). Though engaging in such reflection and challenging these systems will be uncomfortable, such a shift will make our field safer for autistic participants and colleagues, resulting in more valuable and humanizing research.
Acknowledgements
My sincere thanks to Jessica Schomberg, Kieren Fox, and Ryan Randall for their insightful comments and guidance throughout the publication process – your time and thoughtfulness are much appreciated. Many thanks also to Dr. Kristen Bottema-Beutel, whose feedback and mentorship were invaluable as I drafted this piece.
References
Anderson, A. (2018). Autism and the academic library: A study of online communication. College & Research Libraries, 79(5). https://doi.org/10.5860/crl.79.5.645
Anderson, A. (2021). From mutual awareness to collaboration: Academic libraries and autism support programs. Journal of Librarianship and Information Science, 53(1), 103–115. https://doi.org/10.1177/0961000620918628
Association of College and Research Libraries (ACRL). (2015). Framework for Information Literacy. Retrieved from http://www.ala.org/acrl/sites/ala.org.acrl/files/content/issues/infolit/Framework_ILHE.pdf
Baer, A. (2023). Dominant COVID narratives and implications for information literacy education in the “post-pandemic” United States. In The Library With the Lead Pipe. https://www.inthelibrarywiththeleadpipe.org/2023/covid-narratives/
Bakker, T., Krabbendam, L., Bhulai, S., & Begeer, S. (2019). Background and enrollment characteristics of students with autism from secondary education to higher education. Research in Autism Spectrum Disorders, 67, 1–12. https://doi.org/10.1016/j.rasd.2019.101424
Bernard, S., Doherty, M., Porte, H., Al-Bustani, L., Murphy, L.E., Russel, M.C., & Shaw, S.C.K. (2023). Letter to the editor: Upholding autistic people’s human rights: A neurodiversity toolbox for autism research. Autism Research, 16, 683-684. https://doi.org/10.1002/aur.2907
Botha, M. (2021). Academic, activist, or advocate? Angry, entangled, and emerging: A critical reflection on autism knowledge production. Frontiers in Psychology, 4196.
Botha, M., & Cage, E. (2022). “Autism research is in crisis”: A mixed method study of researcher’s constructions of autistic people and autism research. Frontiers in Psychology, 13, 7397.
Bottema-Beutel, K., Kapp, S.K., Lester, J.N., Sasson, N.J, & Hand, B.N. (2021). Avoiding ableist language: Suggestions for autism researchers. Autism in Adulthood, 3(1), 18-29. http://doi.org/10.1089/aut.2020.0014
Bottema-Beutel, K., Kapp, S.K., Sasson, N., Gernsbacher, M.A., Natri, H., & Botha, M. (2023). Anti-ableism and scientific accuracy in autism research: a false dichotomy. Frontiers in Psychiatry, 14, 1-8. http://doi.org/10.3389/fpsyt.2023.1244451
Boyer, A., & El-Chidiac, A. (2023). Come chill out at the library: Creating soothing spaces for neurodiverse students. Journal of New Librarianship, 8(1), 41–47.
Braumberger, E. (2021). Library services for autistic students in academic libraries: A literature review. Pathfinder: A Canadian Journal for Information Science Students and Early Career Professionals, 2(2), Article 2. https://doi.org/10.29173/pathfinder39
Brunskill, A. (2020). “Without that detail, I’m not coming”: The perspectives of students with disabilities on the accessibility information provided on academic library websites. College & Research Libraries, 81(5), 768-788. https://doi.org/10.5860/crl.81.5.768
Cho, J. (2018). Building bridges: Librarians and autism spectrum disorder. Reference Services Review, 46(3), 325–339. https://doi.org/10.1108/RSR-04-2018-0045
Carey, F.C. (2020). Communicating with information: Creating inclusive learning environments for students with ASD. In The Library With The Lead Pipe. https://www.inthelibrarywiththeleadpipe.org/2020/communicating-with-information/
Crompton, C. J., Ropar, D., Evans-Williams, C. V., Flynn, E. G., & Fletcher-Watson, S. (2020). Autistic peer-to-peer information transfer is highly effective. Autism, 24(7), 1704-1712.
Everhart, E. & Anderson, A.M. (2020). Research participation and employment for autistic individuals in library and information science: A review of the literature. Library Leadership and Management, 34(3), 1-6. https://doi.org/10.5860/llm.v34i3.7376
Everhart, N., & Anderson, A. (2020). Academic librarians’ support of autistic college students: A quasi-experimental study. The Journal of Academic Librarianship, 46(5), 102225. https://doi.org/10.1016/j.acalib.2020.102225
Everhart, N., & Escobar, K. L. (2018). Conceptualizing the information seeking of college students on the autism spectrum through participant viewpoint ethnography. Library & Information Science Research, 40(3), 269–276. https://doi.org/10.1016/j.lisr.2018.09.009
Lawrence, E. (2013). Loud hands in the library: Neurodiversity in LIS Theory & Practice. Progressive Librarian, 41, 98–109.
Layden, S.J., Anderson, A., & Hayden, K.E. (2021). Are librarians prepared to serve students with autism spectrum disorder? A content analysis of graduate programs. Focus on Autism and Other Developmental Disabilities, 36(3), 156-164. https://doi.org/10.1177/1088357621989254
Lindsay, S., Cagliostro, E., & Carafa, G. (2018). A systematic review of barriers and facilitators of disability disclosure and accommodations for youth in post-secondary education. International Journal of Disability, Development and Education, 65(5), 526–556. https://doi.org/10.1080/1034912X.2018.1430352
Milton, D. E. (2012). On the ontological status of autism: The ‘double empathy problem’. Disability & Society, 27(6), 883-887.
Pionke, J. J., Knight-Davis, S., & Brantley, J. S. (2019). Library involvement in an autism support program: A case study. College & Undergraduate Libraries, 26(3), 221–233. https://doi.org/10.1080/10691316.2019.1668896
Remy, C., Seaman, P., & Polacek, K. M. (2014). Evolving from disability to diversity: How to better serve high-functioning autistic students. Reference & User Services Quarterly, 54(1), 24–28.
Robinson, B., & Anderson, A. M. (2022). Autism training at a small liberal arts college: Librarian perceptions and takeaways. Public Services Quarterly, 18(3), 161–176. https://doi.org/10.1080/15228959.2021.1988808
Shakespeare, T. (200X?). The social model of disability. In L.J. Davis (Ed.) The disability studies reader (4th ed., pp. 214-221). Routledge.
Shea, G., & Derry, S. (2019). Academic libraries and autism spectrum disorder: What do we know? The Journal of Academic Librarianship, 45(4), 326–331. https://doi.org/10.1016/j.acalib.2019.04.007
Sinclair, J. (2013). Why I dislike ‘person first’ language. Autism Network International, 1(2).
Tumlin, Z. (2019). “This is a quiet library, except when it’s not:” On the lack of neurodiversity awareness in librarianship. Music Reference Services Quarterly, 22(1–2), 3–17. https://doi.org/10.1080/10588167.2019.1575017
Walker, N. (2021). Neuroqueer heresies: Notes on the neurodiversity paradigm, autistic empowerment, and postnormal possibilities. Fort Worth, TX: Autonomous Press.
Walton, K., & McMullin, R. (2021). Welcoming autistic students to academic libraries through innovative space utilization. Pennsylvania Libraries: Research & Practice. https://doi.org/10.5195/palrap.2021.259
Yergeau, M.R. (2018). Authoring autism: On rhetoric and neurological queerness. Duke University Press.
In the Library with the Lead Pipe welcomes substantive discussion about the content of published articles. This includes critical feedback. However, comments that are personal attacks or harassment will not be posted. All comments are moderated before posting to ensure that they comply with the Code of Conduct. The editorial board reviews comments on an infrequent schedule (and sometimes WordPress eats comments), so if you have submitted a comment that abides by the Code of Conduct and it hasn’t been posted within a week, please email us at itlwtlp at gmail dot com!
#ODDStories 2024 @ Marsabit, Kenya 🇰🇪 / Open Knowledge Foundation
The workshop titled “Empowering Young Changemakers: Utilizing Open Data and Indigenous Knowledge for Climate Action” held on March 2nd, 2024, at the Shamz Hotel boardroom was a resounding success. It provided a pivotal platform for young individuals, driven by their determination to combat the effects of climate change within the pastoralist communities. The event, organized by the Pastoralist Peoples’ Initiative in commemoration of Open Data Day 2024, was a half-day workshop that ran from 9:00 am to 02:30 PM.
The event commenced with an opening address by myself, Mr. Basele Stephen, Director of Programs and Partnerships at the Pastoralist Peoples’ Initiatives. I’m an open data expert with extensive experience in open data protocols, and I’ve been at the forefront of mapping exercises in Northern Kenya with open street maps since 2014. My presentation expounded on the core principles of open data and its potential as a potent tool in the battle against climate change.
In my address, I elaborated on the extensive collection of existing open-source data resources related to climate change and its reporting. This equipped the young changemakers with valuable tools to use in their volunteer work and empowered them with a deeper understanding of the potential impact of open data in their efforts to combat climate change.
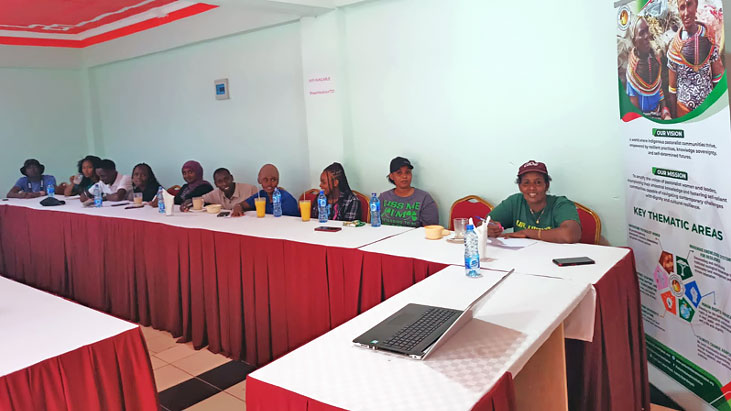
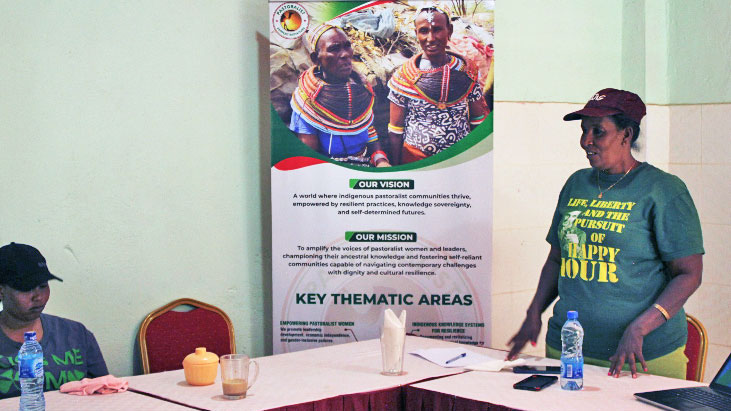
The workshop went beyond the realm of open data by emphasizing the crucial role of indigenous communities in preserving traditional knowledge and incorporating it into climate action. Mr. Isaiah Lekesike and Margaret Super shared their invaluable insights on traditional weather forecasting methods and showcased how these time-tested practices can be seamlessly integrated with modern data analysis techniques, demonstrating the potential for young people to bridge the gap between ancestral knowledge and cutting-edge data analysis.
My address also highlighted the disproportionate impact of climate disasters on pastoralist communities, which contribute minimally to environmental pollution but bear the brunt of its consequences. This served as a call to action for the young changemakers to take on the role of climate adaptation leaders within their communities, inspiring them to become active participants in the fight against climate change.
The ensuing discussions were marked by insightful exchanges and engaging dialogues. The challenges faced by young people on the ground, such as limited internet access, scarcity of information specific to Marsabit County, and hurdles in data collection, analysis, and dissemination, were openly discussed. However, the prevailing sentiment was one of unwavering optimism.
Key Takeaways
The workshop yielded several key takeaways that are instrumental in shaping the future endeavours of the young changemakers and their impact on climate action:
- Open Data as a Powerful Tool: Understanding and utilizing open data provides young changemakers with valuable insights to address climate challenges within their communities.
- The Invaluable Nature of Indigenous Knowledge: Integrating traditional weather forecasting and other practices with modern data analysis can lead to the formulation of more effective climate action strategies.
- Collaboration is Paramount: By working together, young people can overcome the challenges of internet access, information scarcity, and data analysis, fostering a spirit of cooperation and collective action.
- Empowerment Through Knowledge: Equipping young people with the right tools and knowledge fosters a sense of agency and empowers them to become leaders in climate action within their communities.
Despite the challenges, the dedication and resilience displayed by the young minds gathered at the Hallam Restaurant Boardroom promise a brighter future for Marsabit County and beyond. The workshop served as a source of inspiration, equipping the young changemakers with the knowledge of open data and the wisdom of indigenous practices, empowering them to become catalysts for positive change in their communities.
About Open Data Day
Open Data Day (ODD) is an annual celebration of open data all over the world. Groups from many countries create local events on the day where they will use open data in their communities.
As a way to increase the representation of different cultures, since 2023 we offer the opportunity for organisations to host an Open Data Day event on the best date within a one-week period. In 2024, a total of 287 events happened all over the world between March 2nd-8th, in 60+ countries using 15 different languages.
All outputs are open for everyone to use and re-use.
In 2024, Open Data Day was also a part of the HOT OpenSummit ’23-24 initiative, a creative programme of global event collaborations that leverages experience, passion and connection to drive strong networks and collective action across the humanitarian open mapping movement
For more information, you can reach out to the Open Knowledge Foundation team by emailing opendataday@okfn.org. You can also join the Open Data Day Google Group to ask for advice or share tips and get connected with others.
Advocacy, capacity, and program building: Stewarding born-digital archival collections in the RLP / HangingTogether
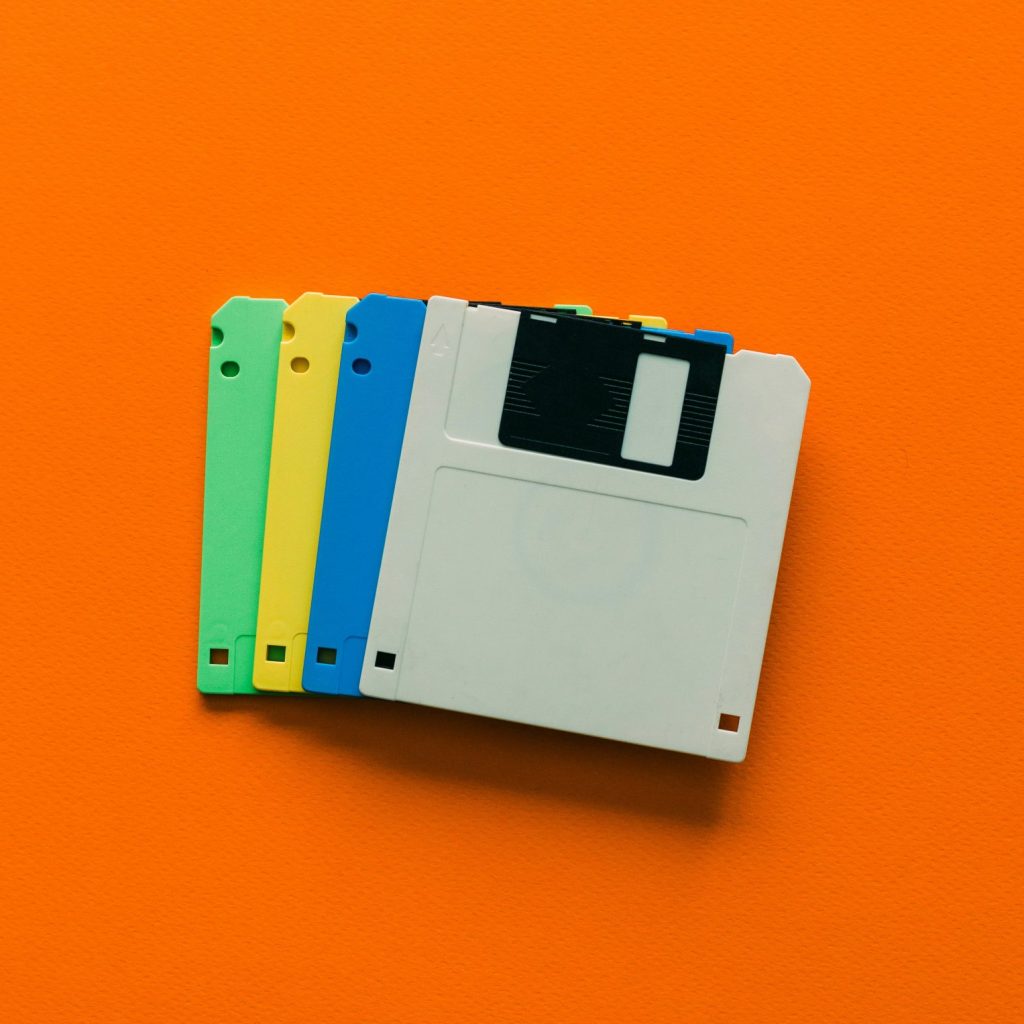
Nearly every archive is grappling with stewarding born-digital archival collections. Practice and programs supporting born-digital archives continue to evolve as we develop increased understanding of needs and available options to address them. The OCLC Research Library Partnership recently held roundtable discussions with special collections leaders that focused on where our programs are now, how we are leading and resourcing them, and how we hope they might shift and grow in the future.
The discussions were illuminating. Thirty-nine people from 36 RLP institutions in three countries attended one of the sessions. The RLP draws from a unique, international mix of independent, academic, national, and museum libraries, with great variety in size and scale of operations. Even with this range, the consistent story across institutions is that this work is still very much in flux and actively being figured out, often with insufficient resources. Good progress is being made in different areas, but nobody reported satisfaction with their ability to handle all the needs of born-digital collections.
Participants were asked to reflect on the following questions to shape the discussion:
- Who has responsibility for born-digital archival collections in your institution? How are you staffing and resourcing support for born-digital collections?
- How are you supporting learning and experimentation for the people in these roles?
- What are your challenges and where do you need support?
The rest of this post summarizes key takeaways and points of commonality from our discussions.
Digital Archivists
Having a role that takes primary responsibility for born-digital collections — whether a digital archivist, digital preservation librarian or archivist, or something similar— was a key indicator, for many participants, of a mature born-digital records program. While many institutions reported having a digital archivist, a significant number of institutions do not. Those that did varied in the length of time the role had existed, ranging from almost a decade to it being a brand-new position. A handful of institutions have a second, more junior role in addition to a digital archivist, either a digital archives technician or a graduate student worker who handled more routine tasks.
Funding and advocacy for such positions was a commonly cited challenge. The stability of funding for such positions varied, with some institutions getting support for a digital archivist through a grant or other time-limited project funding. Several participants described it as a long game: they were slowly but steadily making the case for a permanent role by articulating the need within strategic planning and prioritization discussions. Turnover was an issue, in both leadership and operational roles. This churn in institutional leadership often meant starting advocacy efforts from scratch. And with digital archivist skills in high demand, retention is a challenge as incumbents are lured to a different institution, resulting in slow progress, and necessitating repeatedly advocating for the need to maintain and refill the role.
Participants also spoke of the need for distributed responsibility across the archives for born-digital collections, whether they had a role with primary responsibility for the work or not. For those at institutions with a defined digital archivist role, they did not feel it was scalable to have one person with sole responsibility for stewarding born-digital and expressed concern about employee burnout. One participant described their desired approach: “We need to ramp up teaching the other staff to help them manage more routine materials, saving weird stuff for the specialists.” Others were intentionally working on a model where born-digital was understood as everybody’s job, in the absence of a digital archivist. Those working within a distributed responsibility framework described a need for better understanding of the competencies required for working with born-digital collections across all archival functions and resources for training staff on these competencies.
Matrixed responsibility
In many large institutions, work on born-digital collections spans multiple departments or units. A surprising number of participants described matrixed organizations where this responsibility sits outside of archives and special collections — sometimes in a shared technical services unit, a digitization or reformatting unit, a digital projects or preservation unit, or in an information technology unit.
Discussion participants spoke of the necessity and challenge of working across departments and functions to advocate for needed infrastructure, especially communicating archives-specific requirements that differ from digital materials collected elsewhere in the library. This was especially true when responsibility for born-digital fell in departments more focused on managing faculty or student research outputs, research data, or digitized content.
Digital preservation
An area where almost all participants seemed to be trying to figure things out was addressing digital preservation needs, from tools for initial identification and ingest to ongoing storage. The resources and/or responsibility for this often fell outside special collections. Several participants were using systems initially funded by grants and now needed to find sustainable support. Others described trying to shoehorn born-digital collections into DAMS or other storage systems that weren’t designed to address the needs of archival collections. Those who have been able to implement digital preservation systems talked about what a positive impact it had on their programs. One participant said, “our lives changed when we were able to move to [System].”
Physical versus digital
An interesting thread that ran through the conversations was the difference between digital and physical collections, and the ways the relative invisibility of digital collections impacted work with them. Much of this centered around advocacy and fundraising. When working with donors who are swayed by interesting and engaging collections, it can be hard to entice them to support something they can’t see. Many talked about the challenge of advocating for digital preservation, storage, and other infrastructural needs. As one participant put it, “You can show administration a physical location. You can show them the boxes…and it looks like something to them. They can conceptualize how much is there and what it is. When you’re in a digital environment, and you’re advocating for your collections, it’s harder. … You [can tell them] the terabyte counts, you can tell them about the collection materials, but it’s harder to conceptualize what those collections look like, or how they’re used or what they are. It takes a more advocacy.”
Participants continue to have difficulty estimating the time needed to appraise, accession, and process born-digital collections, something that is relatively well established with physical collections. The lack of reliable metrics for this work makes advocacy even more challenging.
Access and use
Providing access to born-digital collections was another challenge articulated by many participants. Some institutions provide access via a dedicated workstation or laptop in the reading room. A few are providing online access, including authenticated access to materials that can’t be made available on the open web. But most recounted infrastructure limitations that impacted their ability to provide easy access to digital collections. Several institutions manage access requests on a case-by-case basis, which participants recognize as inefficient and inequitable. Other institutions offer separate delivery tools for born-digital and digitized collections, which they characterize as clunky, bifurcated, and not aligned with how researchers understand and want to discover resources.
Participants also discussed the challenge of incorporating born-digital materials into teaching. One observed that students – who lead digital-first lives — don’t connect with electronic material in the same way they do with physical items.
Curatorial challenges
Participants described multiple ways in which born-digital records impact curatorial work. The most common concern was the volume of records coming in and the challenge of performing appraisal on digital materials. With ever-increasing scale of born-digital collections, the high cost and environmental impacts of storage for those collections, and the front-loaded work required to secure and stabilize them, there is an growing realization that more appraisal work is required before collections are brought in. This challenge is two-fold — the learning curve of working with electronic records coupled with a paradigm shift from appraising physical collections. There is a recognized need to build born-digital appraisal skills among those charged with collection building and to integrate appraisal tools into the pre-acquisition process. Along similar lines, one participant voiced the concern that we are not having enough conversations about how born-digital records are being used, which is leading to uninformed collecting. They went on to say, “it feels like we’re headed for [a] conversation in 20 years about corrective appraisal.”
Several institutions described a lot of investment in born-digital collecting “before we really knew what we were doing,” leading to a significant backlog of collections that need baseline control. That stewardship debt continues to accumulate as they continue to collect. Some archives are declining offers of born-digital collections because of lack of stewardship capacity, describing this as a case-by-case decision. There is anxiety that a “great collection is going to come along, and we’re not going to be in a position to handle it.” Some participants were trying to mitigate past missteps by addressing born-digital in their collection development policy, in some cases putting limitations on what they collect. Others had updated acquisitions procedures and deeds of gift to obtain the permissions they need to serve born-digital to researchers.
Conversely, a move to digital formats is also making it harder to acquire materials that previously arrived in physical form. This was especially true for archives charged with collecting university records or administrative records of their parent organization. Participants explained that senior administrators don’t want to transfer their email; things like electronic newsletters must now be sought out, and in general people don’t seek out transfers to the archives now because they haven’t run out of physical space. “It used to be that people would run out of space in their filing cabinets and call the archives, but those days are over.”
Conclusion
All in all, these were interesting and illuminating conversations that illustrated both how much progress archives have made in working with born-digital collections, and how much work remains. Our evaluations indicated that participants found comfort in learning that they weren’t the only ones struggling to do this work responsibly and to address all the various needs of born-digital. This is a great outcome, as our goal with these roundtables is to bring together library leaders to facilitate peer learning, benchmarking of services, and community building among RLP members. My hope is that the roundtables will continue to provide a space to build community, offer support, and share knowledge.
If you are a member of the RLP and don’t have someone participating in our leadership roundtables, either this one focused on archives and special collections and the other on research support, you can find more information here. Please reach out, we’d love to have you join us.
The post Advocacy, capacity, and program building: Stewarding born-digital archival collections in the RLP appeared first on Hanging Together.
DLF Digest: April 2024 / Digital Library Federation
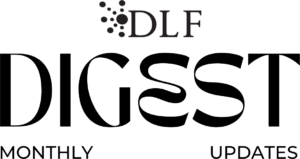
A monthly round-up of news, upcoming working group meetings and events, and CLIR program updates from the Digital Library Federation. See all past Digests here.
Hello and happy April, DLF community! It’s spring, and our community is certainly staying busy. We have many exciting group meetings coming up this month, along with some Forum news we’ll be able to share in the coming weeks. Read on to learn how to be a part of all of the great things happening in our world this month. We’ll see you around soon!
– Team DLF
This month’s news:
- Sponsorship Opportunities Available: Sponsorship opportunities for the 2024 DLF Forum, in person in July and online in October, are now available.
- Application Remain Open: CLIR invites applications from collecting organizations for the digital reformatting of audio and audiovisual materials for the Recordings at Risk grant program now through April 17, 2024.
- Program Now Available: The preliminary program for the IIIF Annual Conference is now available, to be held in Los Angeles, June 4-7. Review the program and register for the conference.
- Registration Remains Open: Registration is still open for the International Internet Preservation Consortium’s (IIPC) General Assembly and Web Archiving Conference in Paris at the end of this month, April 24-26.
- New Publication: The Journal of eScience Librarianship (JeSLIB) and the Responsible AI in Libraries and Archives project have published a Special Issue on Responsible AI in Libraries and Archives; read it here.
This month’s open DLF group meetings:
For the most up-to-date schedule of DLF group meetings and events (plus NDSA meetings, conferences, and more), bookmark the DLF Community Calendar. Can’t find meeting call-in information? Email us at info@diglib.org. Reminder: Team DLF working days are Monday through Thursday.
- Born-Digital Access Working Group (BDAWG): Tuesday, April 2, 2pm ET / 11am PT.
- Digital Accessibility Working Group (DAWG): Wednesday, April 3, 2pm ET / 11am PT.
- Assessment Interest Group (AIG) Cultural Assessment Working Group (CAWG): Monday, April 8, 2pm ET/11am PT.
- AIG Cost Assessment Working Group: Monday, April 8, 3pm ET/12pm PT.
- AIG Metadata Assessment Working Group: Thursday, April 11, 1:15pm ET / 10:15am PT.
- AIG User Experience Working Group: Friday, April 19, 11am ET / 8am PT.
- Committee for Equity and Inclusion (CEI): Monday, April 22, 3pm ET / 12pm PT.
- Climate Justice Working Group: Wednesday, April 24, 12pm ET / 9am PT.
- DAWG Policy & Workflows subgroup: Friday, April 26, 1pm ET / 11am PT.
DLF groups are open to ALL, regardless of whether or not you’re affiliated with a DLF member organization. Learn more about our working groups on our website. Interested in scheduling an upcoming working group call or reviving a past group? Check out the DLF Organizer’s Toolkit. As always, feel free to get in touch at info@diglib.org.
Get Involved / Connect with Us
Below are some ways to stay connected with us and the digital library community:
- Subscribe to the DLF Forum newsletter.
- Join, start, or revive a working group and browse their work on the DLF Wiki.
- Subscribe to our community listserv, DLF-Announce.
- Bookmark our Community Calendar.
- Learn more about becoming a DLF member organization.
- Follow us on Instagram, Facebook, LinkedIn, YouTube, and X.
- Contact us at info@diglib.org.
The post DLF Digest: April 2024 appeared first on DLF.
5 Knowledge Management Best Practices for Better CX / Lucidworks
Key insights on optimizing CX through knowledge management technology, search solutions, personalization, and human-centric design.
The post 5 Knowledge Management Best Practices for Better CX appeared first on Lucidworks.
New Endeavors at the Library Innovation Lab / Harvard Library Innovation Lab
This month, the Library Innovation Lab celebrated the full and unqualified release of the Caselaw Access Project data. We took the opportunity to gather and look to the future at Transform: Justice on March 8th. The event reminded us of what we already knew: the open legal data movement is alive and well.
In addition to hearing the story of CAP, librarians, vendors, and advocates had conversations about future paths for accessing the law. Noted open-access activist Carl Malamud made his pitch for a Full Faith and Credit Act.
As we see CAP move on to a new chapter, LIL will be bringing the same experimental mindset that allowed for such an ambitious project into other endeavors.
Here’s some of what’s coming up next at the Library Innovation Lab:
Facilitating open, equitable access to knowledge
COLD Cases: We’re excited to be collaborating with the Free Law Project on a couple of projects. First, we worked with FLP to create COLD Cases, a pipeline for standardizing and sharing bulk legal data.
Teraflop & CAP: It’s great to see work by the AI community in processing the CAP dataset for legal understanding, such as this work by Teraflop to generate text embeddings and search indexes for the data.
Future Collaborations: We see potential for Harvard to be a neutral platform for releasing data that helps the whole industry. We are having conversations with companies like Westlaw, LexisNexis, and vLex that see potential in this kind of partnership, and we hope to make more connections soon.
Empower everyone – AI, understanding, access to justice
Library Innovation Lab Artificial Intelligence Fund: LexisNexis is the first funder of our AI fund, which has been set up to support exploration at the edge of law, AI technology, libraries, and society.
Open Legal AI Workbench: Our recently released Open Legal AI Workbench is a simple, well-documented, and extensible framework for legal AI researchers to build services using tool-based retrieval augmented generation.
Keep your eyes peeled for more news about the Institutional Data Initiative, a plan to create a trusted conduit for legal data for AI. By pairing high-quality corpora and collection expertise with industry resources, it aims to scale the collaborations between knowledge institutions and industry partners that we discussed at Transform: Justice. The goal is to grow both knowledge and the public interest.
#ODDStories 2024 @ Milan, Italy 🇮🇹 / Open Knowledge Foundation
On March 4th, 2024, the Geodetic and Photogrammetric Laboratory (LabMGF) of the Department of Civil and Environmental Engineering at Politecnico di Milano (Italy) hosted the webinar Mapping Climate Change in 4D: Belvedere Glacier’s Open Geo Data for Education and Research. This event was held in celebration of OpenDataDay 2024.
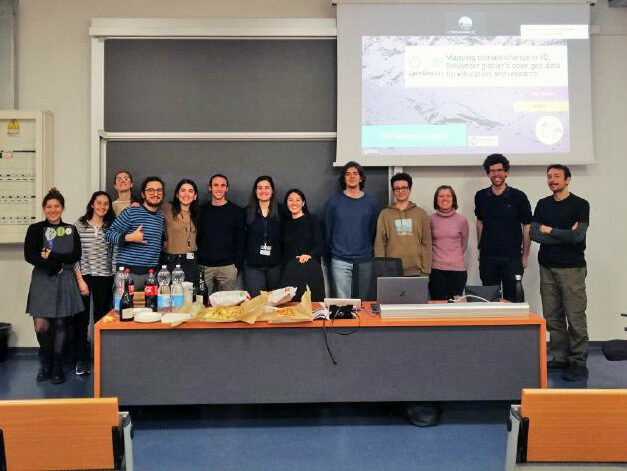
Goal
The webinar showcased the extensive geospatial open data collected on the Belvedere Glacier (Italian Alps). This comprehensive dataset was generated through long-term monitoring using innovative UAV and aerial photogrammetry techniques, a collaborative effort between Politecnico di Milano’s DICA department, Politecnico di Torino’s DIIATI department, and the Alta Scuola Politecnica. The event emphasized the value of this open data for research and educational purposes, readily accessible on Zenodo.
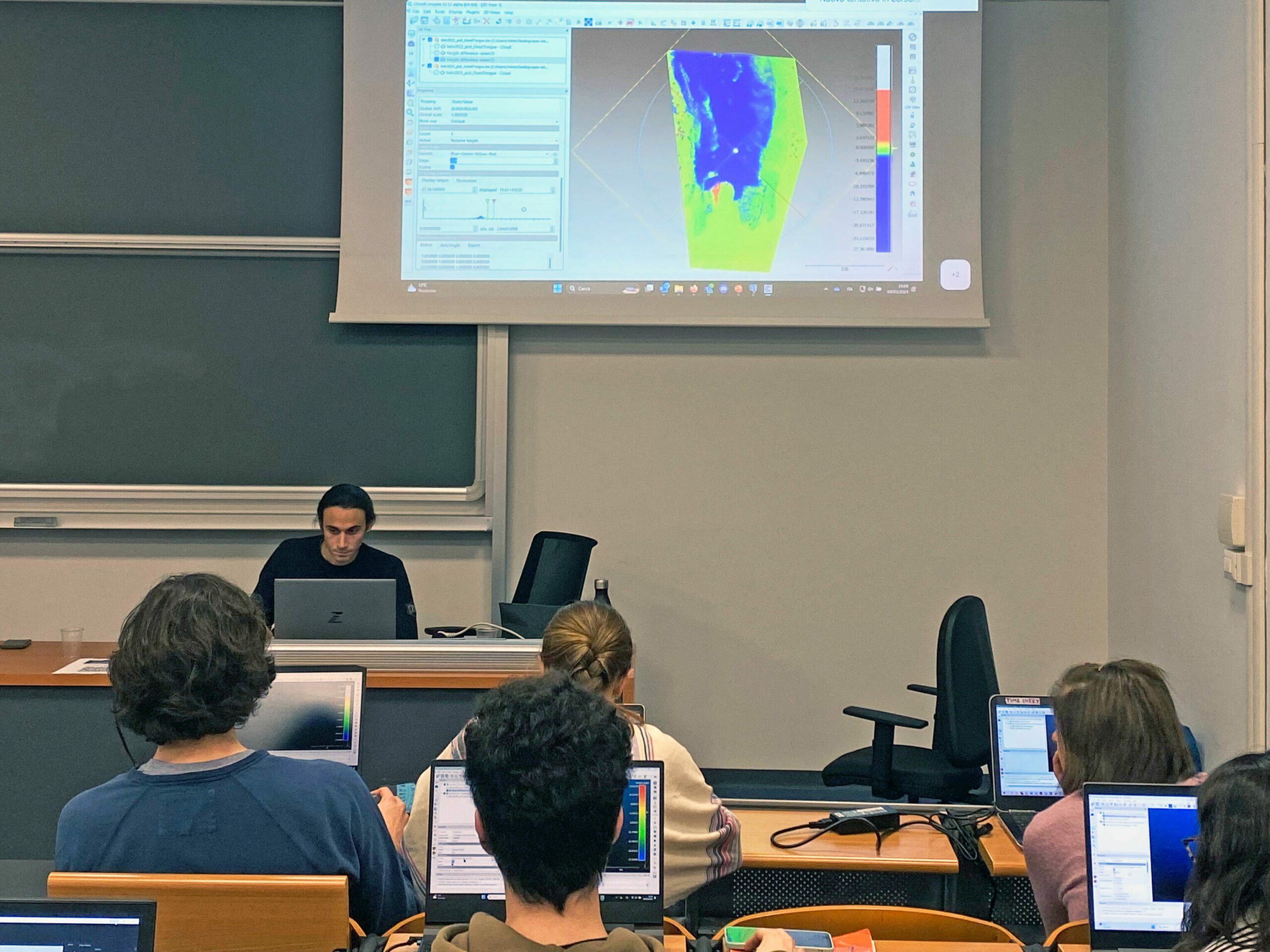
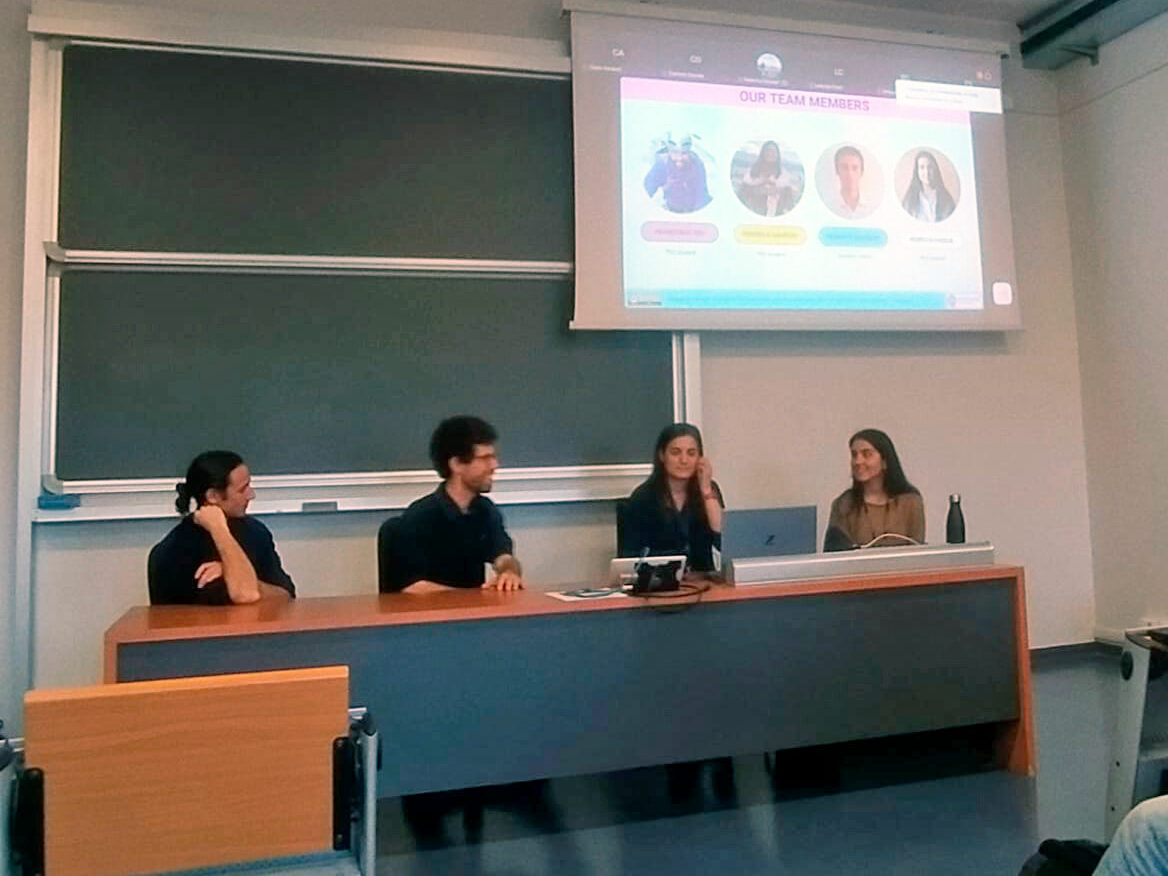
Format and Participation
The webinar combined in-person attendance at Politecnico di Milano with online streaming, enabling wider participation.
The event was organized in two modules, as follows:
Module 1: Introduction (~1 hour)
- Background on the Belvedere Glacier monitoring and research project
- Showcase of the unique Summer School initiative, “Design and Implementation of Topographic Surveys for Territorial Monitoring in Mountain Environments,” serving BSc and MSc students since 2016.
- Presentation on the preparation and significance of the long-term Belvedere Glacier monitoring results and the publicly available open data on Zenodo.
Module 2: Hands-on Workshop (~1.5 hours)
- In-depth tutorials using open-source software (QGIS and CloudCompare) for DTM and point cloud processing, fostering practical skills.
Approximately 20 participants attended the first module in-person, while 9 people attended both modules on-site. Additional 25 people joined the event online.
Resources
- Webinar Slides
- Belvedere Glacier Open Data (Zenodo): Version 2.0 includes 3D point clouds, DSM, and orthophotos from 1977 to 2023. License: GPU GPL 3.0.
The Future of AI in Search and Discovery for Manufacturers and Distributors / Lucidworks
AI has the potential to transform search and discovery processes for manufacturing buyers. Find out how.
The post The Future of AI in Search and Discovery for Manufacturers and Distributors appeared first on Lucidworks.
Time: It doesn’t have to be this way / Meredith Farkas
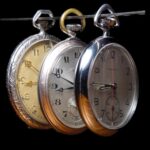
“What we think time is, how we think it is shaped, affects how we are able to move through it.”
-Jenny Odell Saving Time, p. 270
This is the first of a series of essays I’ve written on time. Here are the others (they will be linked as they become available on Information Wants to be Free):
- With Work Time at the Center
- The Productivity Trap
- Meredith’s Slow Productivity (not to be mistaken for Cal Newport’s Faux Slow Productivity)
- Queer Time, Crip Time, and Subverting Temporal Norms
- Community Time and Enoughness: The heart of slow librarianship
What I love about reading Jenny Odell’s work is that I often end up with a list of about a dozen other authors I want to look into after I finish her book. She brings such diverse thinkers beautifully into conversation in her work along with her own keen insights and observations. One mention that particularly interested me in Odell’s book Saving Time (2023) was What Can a Body Do (2020) by Sara Hendren. Her book is about how the design of the world around us impacts us, particularly those of us who don’t fit into the narrow band of what is considered “normal,” and how we can build a better world that goes beyond accommodation. Her book begins with the question “Who is the built world built for?” and with a quote from Albert Camus: “But one day the ‘why’ arises, and everything begins in that weariness tinged with amazement” (1).
“Why” is such a simple world, but asking it can completely alter the way we see the world. There’s so much in our world that we simply take for granted or assume is the only way because some ideology (like neoliberalism) has so deeply limited the scope of our imagination. Most of what exists in our world is based on some sort of ideological bias and when we ask “why” we crack the world open and allow in other possibilities. Before I read the book Invisible Women (2021) by Caroline Criado Perez, I already knew that there was a bias towards men in research and data collection as in most things, but I didn’t realize the extent to which the world was designed as if men were the only people who inhabited it and how dangerous and harmful it makes the world for women. What Can a Body Do similarly begins with an exploration of the construction of “normal” and how design based on that imagined normal person can exclude and harm people who aren’t considered normal, particularly those with disabilities. The book is a wonderful companion to Invisible Women in looking at why the world is designed the way it is and how it impacts those who it clearly was not built for. I’ll explore that more in a later essay in this series.
One thing I took for granted for a very long time was time itself. I thought of time in terms of clocks and calendars, not the rhythms of my body nor the seasons (unless you count the start and end of each academic term as a season). I believed that time was scarce, that we were meant to use it to do valuable things, and that anything less was a waste of our precious time. I would beat myself up when, over Spring Break, I didn’t get enough practical home or scholarship projects done or if I didn’t knock everything off my to-do list at the end of a work week. I would feel angry and frustrated with myself when my bodily needs got in the way of getting things done (I’m writing this with ice on both knees due to a totally random flare of tendinitis when I’d planned to do a major house cleaning today so I’m really glad I don’t fall into that shooting myself with the second arrow trap as much as I used to). I looked for ways to use my time more efficiently. I am embarrassed to admit that I owned a copy of David Allen’s Getting Things Done and tried a variety of different time management methods over the years that colleagues and friends recommended (though nothing ever stuck besides a boring, traditional running to-do list). I’d often let work bleed into home time so I could wrap up a project because not finishing it would weigh on my mind. I was always dogged by the idea that I wasn’t getting enough done and that I could be doing things more efficiently. It felt like there was never enough time all the time.
I didn’t start asking questions about time until I was 40 and the first one I asked was a big one “what is the point of our lives?” Thinking about that opened a whole world of other questions about how we conceive of time, what kinds of time we value, to what end are we constantly trying to optimize ourselves, what is considered productive vs. unproductive time, why we often value work time over personal time (if not in word then in deed), why time often requires disembodiment, etc. The questions tumbled out of me like dominoes falling. And with each question, I could see more and more that the possibility exists to have a different, a better, relationship with time. I feel Camus’ “weariness, tinged with amazement.”
This is an introduction to a series of essays about time: how we conceive of it, how it drives our actions, perceptions, and feelings, and how we might approach time differently. I’ll be pulling ideas for alternative views of time from a few different areas, particularly queer theory, disability studies, and the slow movement. I’m not an expert in all these areas, but I’ll be sure to point you to people more knowledgeable than me if you want to explore these ideas in more depth.
How many of you feel overloaded with work? Like you’re not getting enough done? How many of you are experiencing time poverty: where your to-do list is longer than the time you have to do your work? How many of you feel constantly distracted and/or forced to frequently task-switch in order to be seen as a good employee? How many of you feel like you’re expected to do or be expert in more than ever in your role? How many of you feel like it’s your fault when you struggle to keep up? More of us are experiencing burnout than ever before and yet we keep going down this road of time acceleration, constant growth, and continuous availability that is causing us real harm. People on the whole are not working that many more hours than they used to, but we are experiencing time poverty and time compression like never before, and that feeling bleeds into every other area of our lives. If you want to read more about how this is impacting library workers, I’ll have a few article recommendations at the end of this essay.
My exploration is driven largely by this statement from sociologist Judy Wajcman’s (2014) excellent book Pressed for Time: “How we use our time is fundamentally affected by the temporal parameters of work. Yet there is nothing natural or inevitable about the way we work” (166). We have fallen into the trap of believing that the way we work now is the only way we can work. We have fallen into the trap of centering work temporality in our lives. And we help cement this as the only possible reality every time we choose to go along with temporal norms that are causing us harm. In my next essay, I’m going to explore how time became centered around work and how problematic it is that we never have a definition of what it would look like to be doing enough. From there, I’m going to look at alternative views of time that might open up possibilities for changing what time is centered around and seeing our time as more embodied and more interdependent. My ideas are not the be-all end-all and I’m sure there are thinkers and theories I’ve not yet encountered that would open up even more the possibilities for new relationships with time. To that end, I’d love to get your thoughts on these topics, your reading recommendations, and your ideas for possible alternative futures in how we conceive of and use time.
Works on Time in Libraries
Bossaller, Jenny, Christopher Sean Burns, and Amy VanScoy. “Re-conceiving time in reference and information services work: a qualitative secondary analysis.” Journal of Documentation 73, no. 1 (2017): 2-17.
Brons, Adena, Chloe Riley, Ean Henninger, and Crystal Yin. “Precarity Doesn’t Care: Precarious Employment as a Dysfunctional Practice in Libraries.” (2022).
Drabinski, Emily. “A kairos of the critical: Teaching critically in a time of compliance.” Communications in Information Literacy 11, no. 1 (2017): 2.
Kendrick, Kaetrena Davis. “The public librarian low-morale experience: A qualitative study.” Partnership 15, no. 2 (2020): 1-32.
Kendrick, Kaetrena Davis and Ione T. Damasco. “Low morale in ethnic and racial minority academic librarians: An experiential study.” Library Trends 68, no. 2 (2019): 174-212.
Lennertz, Lora L. and Phillip J. Jones. “A question of time: Sociotemporality in academic libraries.” College & Research Libraries 81, no. 4 (2020): 701.
McKenzie, Pamela J., and Elisabeth Davies. “Documenting multiple temporalities.” Journal of Documentation 78, no. 1 (2022): 38-59.
Mitchell, Carmen, Lauren Magnuson, and Holly Hampton. “Please Scream Inside Your Heart: How a Global Pandemic Affected Burnout in an Academic Library.” Journal of Radical Librarianship 9 (2023): 159-179.
Nicholson, Karen P. “Being in Time”: New Public Management, Academic Librarians, and the Temporal Labor of Pink-Collar Public Service Work.” Library Trends 68, no. 2 (2019): 130-152.
Nicholson, Karen. “On the space/time of information literacy, higher education, and the global knowledge economy.” Journal of Critical Library and Information Studies 2, no. 1 (2019).
Nicholson, Karen P. ““Taking back” information literacy: Time and the one-shot in the neoliberal university.” In Critical library pedagogy handbook (vol. 1), ed. Nicole Pagowsky and Kelly McElroy (Chicago: ACRL, 2016), 25-39.
Awesome Works on Time Cited Here
Hendren, Sara. What Can a Body Do?: How We Meet the Built World. Penguin, 2020.
Odell, Jenny. Saving Time: Discovering a Life Beyond Productivity Culture. Random House, 2023.
Wajcman, Judy. Pressed for time: The acceleration of life in digital capitalism. University of Chicago Press, 2020.
With Work Time at the Center / Meredith Farkas
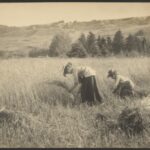
This is the second in a series of essays I’ve written on time. You can view a list of all of them on the first essay.
Once upon a time, people lived more by the natural rhythms of seasons, the movement of the sun, and their bodies. There weren’t clocks to tell them when to do things and there wasn’t electric light and heat to make it easy to pretend it’s normal to work in the dark (burning the midnight oil used to be a real thing, and wasteful!). People didn’t live by precise times and society didn’t require the sort of coordination and standardization we have today. We’ve lived so long with clocks, it can be hard to imagine waking with the sun rather than at a specific appointed number on a clock every day. Clock time might even feel to us a more objective measure of time because it’s what we’ve used all our lives to determine our waking and sleeping, our work time and leisure, and our times to eat and exercise. In reality, it’s the most contrived measure of time, one deeply encumbered by social values and largely designed around economic needs.
Precision and coordination in measuring time was first used by monks to ensure they were adhering to the proper times for prayer, but even those times often differed depending on the time of year (the position of the sun). It wasn’t until Huygens developed the pendulum clock that clock time came into wider use, but this was also the moment of the birth of industry, which required time discipline, the “high degrees of standardization and regularity and coordination” of people’s time (Glennie & Thrift 1996, 287). E. P. Thompson, who wrote the seminal work on the growth of clock time in early modern Britain, writes about how quickly clock time and time as a commodity became the only ways of conceiving of time:
The first generation of factory workers were taught by their masters the importance of time; the second generation formed their short-time committees in the ten-hour movement; the third generation struck for overtime or time-and-a-half. They had accepted the categories of their employers and learned to fight back within them. They had learned their lesson, that time is money, only too well.
-E. P. Thompson 1967, 86
Even in that early-modern era, there wasn’t the sort of standardization of time we see today. The idea of standard times and time zones are a 19th Century invention that wasn’t fully adopted by the entire world well into the 20th Century. Localities used to have their own time — originally determined by the movement of the sun — which was not a problem until the growth of fast transportation and communication technologies made those local differences more glaring and inconvenient. Eventually, Greenwich Mean Time became the standard all were forced to follow, though even now, the main tower clock in Bristol, England has a third hand that denotes their original local time. Like so many things that stand in opposition to nature, clock time became a tool of oppression: “the Western separation of clock time from the rhythms of nature helped imperialists establish superiority over other cultures” (Zadeh). Barbara Adam (2002), who has written brilliantly on the sociology of time, rightly brings up the fact that those natural rhythms did not cease to exist with the invention of clock time: “Yet clock time has not replaced the multiple social, biological, and physical sources of time; it has rather changed the meanings of the variable times, temporalities, timings, and tempos of bio-cultural origin… Machine time has been reified to a point at which we have lost touch with other rhythms and with the multiple times of our existence” (513-14).
At the same time that this was happening, Protestantism was preaching time discipline, with leisure seen as an affront to God, and labor “serve[ed] to increase the glory of God, according to the definite manifestations of His will” (Weber Ch. 5). According to Max Weber, this Protestant ethic has become fully secularized over time, though the fervor behind people’s sense of vocation and the importance of money-making beyond real need still feels almost religious in its fervor:
If you ask them what is the meaning of their restless activity, why they are never satisfied with what they have, thus appearing so senseless to any purely worldly view of life, they would perhaps give the answer, if they know any at all: “to provide for my children and grandchildren.” But more often and, since that motive is not peculiar to them, but was just as effective for the traditionalist, more correctly, simply: that business with its continuous work has become a necessary part of their lives. That is in fact the only possible motivation, but it at the same time expresses what is, seen from the viewpoint of personal happiness, so irrational about this sort of life, where a man exists for the sake of his business, instead of the reverse.
-Max Weber, ch 2.
These forces all helped to place work time at the center of our lives and cement the notion of time as money; two things that have only been further entrenched in our contemporary neoliberal society. Time discipline was originally enforced by managers, but we have seen “a movement away from an outer, visible coercion toward an inner regulation administered by the individual himself” (Rosengren 2019, 622). We are driven to reproduce time discipline ourselves, more by virtue of our own sense of precarity (whether because of the nature of our job, our internal insecurity, or both) and individualistic desire to rise than by specific discipline from a manager.
Luckily, some of us are starting to question what is behind all this. While business leaders were promoting the idea of quiet quitting as a dereliction of duty, most of us could clearly see that what they were describing as “quiet quitting” was “just doing your job.” We’ve been so conditioned to see going above and beyond as the minimum expectation that the idea of doing just what is required of us was seen as tantamount to quitting.
I’ve been working in libraries for 20 years at this point and I’ve felt over the past thirteen years more overburdened and exhausted than I did in the preceding seven. The pace of work feels like it’s accelerating. And I could assume that it’s just me and aging and the fact that I’ve been a parent for all of those latter years, but the literature suggests that this goes far beyond my individual experience. We’re being asked to do more than ever before. As a liaison librarian, I remember reading New Roles for New Times a decade ago and thinking how difficult it would be to develop expertise in all of the listed areas. Since then, we’ve been asked to become experts in even more, like AI, algorithmic bias, open educational resources, and more. And we’re also subject to more interruptions than ever before (as Lennertz and Jones; Bossaller, Burns, and VanScoy; and Nicholson all highlight). Bossaller, Burns and VanScoy (2017) found that the librarians they interviewed “experienced time famine, time pressure, time poverty, and time fatigue” (15). Even if you’re not working more hours, the feeling that you can never catch up, that you’re drowning in to-do’s can have a significantly negative impact on your mental health and relationships (Giurge, Whillans, and West 2020).
I’ve seen the argument made that since people aren’t actually working that much more than they did 30 years ago, this stress is of their own creation, but I take that claim about as seriously as the claim that people just aren’t as “resilient” as they used to be. There are systemic changes that some are trying to frame as individual changes to get us to grind harder. I’ve written in the past about the normalization of overwork and Brons, et al. (2022) have written about how precarity helps to further entrench overwork as the norm. Mazzetti, Schaufeli, & Guglielmi (2014) demonstrated that while there might be personality characteristics common to workaholics, the greatest determinant of overwork is the organizational climate. When overwork is seen as the minimum expectation and it is rewarded with promotions and raises, it becomes the base expectation. Yet the research on the negative long-term effects of overwork is unequivocal:
Overwork refers specifically to the cumulative consequences of operating at ‘‘overcapacity.’’ Additional hours spent at work eventually creates fatigue or stress so that the worker’s physical or mental health, well-being, health, or quality of life is not sustainable in the longer run. Adverse effects of excess work on various indicators of worker’s well-being from individuals and families to employers and the (national or global) economy have been fairly well established empirically.
-Golden & Altman, 65
Our organizations are short-sightedly running their employees into burnout or worse when a sustainable pace would likely provide better long-term outcomes for the organization (and the individual!).
Urgency is also a huge part of the timescape of libraries. Lennertz and Jones (2020) write about a study that “found greater urgency in the university than the factory” which surprises me not at all. I think that’s become true of a lot of knowledge work-type jobs. I’ve seen libraries go through multiple overlapping cycles of “crisis” and what Meyers et al. (2021) refer to as “the exceptional present” in order to create urgency and pressure us to overwork. Because when there’s a crisis, we all need to step up and do our part. But what if we’re just in a constant state of crisis? It seems like there’s always another reason to step up and do more and its treated like a temporary blip. I remember for years at the past two libraries I worked with, we kept putting off major departmental planning until things settled down because we felt we were always in reaction mode. But things never settled down at either library. And being in a constant state of crisis and urgency eventually wears you out and numbs you. We can’t operate in reactive mode forever; it’s just not healthy for us as individuals or for the organization. It makes thoughtful and inclusive planning impossible. And urgency has been called out as being a characteristic of white supremacy culture.
There’s also this feeling that we need to keep growing and building limitlessly; there’s no vision for what enough might actually look like. I remember years ago when I first became an instruction head, my boss asked me to have as an annual goal to increase instruction sessions by 25%. A daunting task, right? Well, through quite a lot of outreach and the generosity of my fellow instruction librarians, we actually met that goal! Woo hoo! But to my surprise, my boss wanted me to put the exact same metric into my goals for the following year. This time I objected. The first reason was because we had a lot of folks who were new to or were uncomfortable with teaching and it made a lot more sense to focus that year on the quality of our teaching than the quantity (because who cares that you’re teaching a lot if your teaching is not effective? We’re not made of magic). But her request also made me wonder at what point would we be teaching enough? What was the magic number? Would I be expected to increase the number of classes we taught endlessly? And how would we manage all that teaching (and outreach!) with already full workloads? Our profession is incredibly bad at defining what enough looks like and I think it’s a key reason why we constantly add new projects and services without considering long-term sustainability. We feel like we have to constantly do more and new things to prove our worth, and the worth of the library, and it’s an exhausting treadmill we could run on forever.
We talk a lot in libraries about work-life balance, but I’ve come to believe a balance is really impossible if work is always treated as more important than life. Work time dominates and shapes the rest of our time, impacting our wellness and our relationships. Arlie Russell Hochschild (1997) documented how work time encroaches on family and leisure time in her ethnographic research: “the more its deadlines, its cycles, its pauses and interruptions shape our lives and the more family time is forced to accommodate to the pressures of work” (45). When we are at home, work interruptions (like a quick email or something) are considered no big deal these days, but we’re expected when we’re at work to be 100% focused on work and pretend that we don’t have bodies, caregiving responsibilities, and worries in our lives. We’re expected to shut those parts of ourselves off. But how many times have you checked work email before you’ve gone to work or on the weekend “just in case?” It’s become totally acceptable for work to encroach on our personal lives, but not the other way around. We take pains to keep our lives from spilling into our work.
I remember when I had a baby and experienced the absurdity of being expected to suddenly show up back at work and do exactly as much as I did before. I was suffering from postpartum depression and both my son and I were dealing with one health issue after another, but the message coming from work and society was that if I wasn’t just as productive as I’d been pre-baby, I was a failure. I didn’t feel like I could ask for help at work because we’re socialized to see that as weakness and asking for preferential treatment. I felt pressure to be perfect at home lest I make some minor mistake that harmed my child’s entire future (the mommy message boards were full of fear-mongering) and to also prove that I could still do all my work, speak at conferences, write book chapters and articles, and do everything at just as high a level as I ever did. It almost killed me. And I truly believe that our culture pushes caregivers to pursue a level of perfection that is unsustainable. I identified so much with this quote from Mitchell, Magnusen, and Hampton’s (2023) autoethnography about burnout:
I knew that being a single mother made me a liability – at least in a world where capitalism is valued over having an actual life. I say this because in my experience, in academic spaces, it was expected that all employees leave our personal lives at the door. We were strictly meant to focus on work and not concern ourselves with our personal lives once we were on the clock. (166)
We live and work in a society that believes we shouldn’t have bodies, loved ones, or needs outside of the workplace at any time while we’re working. Stuffing those things down while we’re at our jobs tends only to exacerbate those issues. As someone who taught and worked reference shifts with migraines, I can attest that muscling through the pain only made it more difficult to get rid of the migraine once I got back home. I once ended up spending over $1000 and a whole summer in physical therapy (and pain) because I ignored the harm my desk chair was causing me and kept sitting in it for work despite the growing pain in my hips. Yet I blithely let work bleed into my personal life without a second thought and gave so many hours of my personal time to work without thinking about the cost to my well-being and that of my loved ones.
I’m going to write more about things that push us out of sync with work temporality (disability, caregiving, etc.) and more embodied and interdependent ways to be at work in a future essay in this series. We can’t just be floating brains at work with no encumbrances; what makes us great at our jobs is everything that makes us who we are. We are whole people and deserve to be valued for our wholeness. We need to stop punishing people (whether explicitly or through the cultural norms we’ve created) for having needs that may sometimes interfere with work.
Some might counter with the fact that we have more flexibility at work than ever. Some of us can work from home. Some of us can flex our time to be at our child’s play or our loved one’s medical appointment. We can often work from anywhere we have an internet connection. Workplace flexibility has been touted as being beneficial for workers (especially caregivers and those with illnesses or disabilities), but is usually gamed to benefit the employer. Golden and Altman (2006) and Anttila, et al. (2015) found that workers with more flexibility tend to work longer hours. I’ve certainly seen it myself where those who take advantage of flexible hours to accommodate caregiving needs feel obligated to prove their worthiness by working even more. It’s like how so many child-bearing academics are afraid of accepting a tenure clock extension because they fear they will be seen as weak or their tenure packet judged more harshly (and in many cases, they are). I’d imagine that those who do accept the extension feel enormous pressure to prove that they are even more productive as those who do not have such a gap in their CVs.
Flexibility and our 24/7 culture combine to create an expectation of constant availability. And the flex tends to be toward work, not toward the needs in our personal lives. We stay late, we check work email at night or when we first wake up in the morning, we do work on our laptops while watching TV with the family and pretend that it’s quality time. Lennertz and Jones (2020) found that the vast majority of library workers have had to do work during vacations, which is patently absurd in a profession where no one is going to die due to our lack of availability. Bourne and Forman (2014) suggest that flexibility won’t help fix work-life balance issues as long as our society continues to value work time and devalue what we do outside of work.
Nothing will materially change until we 1) change the calculus where work is seen as more important than anything else in our lives and 2) resist norms around overwork, availability, and response times. And really, it has to be those of us with the most privilege and job security to fight the hardest to change these norms in our organizations because those working in precarity will not have the safety to do so outside of a union (and even then, it can be risky in some orgs). Every time we check our email outside of work hours, every time we “just finish this up” when we’re supposed to be spending time with loved ones, every time we overwork, we are helping to reproduce the existing norms. I’ve written in the past about uncoupling our sense of worth from our work identity and achievements and I think that’s an important first step toward changing work’s dominant place in our lives. But it’s going to require real collective action and solidarity to change the norms, especially when there’s always the promise that if you, as an individual, work harder, you will be rewarded. This monstrous treadmill will never stop unless we stand together.
What you contribute to work is not the measure of your worth. And it’s certainly not more important than you or the people you love. Can you imagine how things might look different if work were not centered?
Adam, Barbara. “Perceptions of time.” In Companion encyclopedia of anthropology, ed. Tim Ingold pp. 503-526. Routledge, 2002.
Anttila, Timo, Tomi Oinas, Mia Tammelin, and Jouko Nätti. “Working-time regimes and work-life balance in Europe.” European Sociological Review 31, no. 6 (2015): 713-724.
Bourne, Kristina A., and Pamela J. Forman. “Living in a culture of overwork: An ethnographic study of flexibility.” Journal of Management Inquiry 23, no. 1 (2014): 68-79.
Bossaller, Jenny, Christopher Sean Burns, and Amy VanScoy. “Re-conceiving time in reference and information services work: a qualitative secondary analysis.” Journal of Documentation 73, no. 1 (2017): 2-17.
Brons, Adena, Chloe Riley, Ean Henninger, and Crystal Yin. “Precarity Doesn’t Care: Precarious Employment as a Dysfunctional Practice in Libraries.” (2022).
Glennie, P. and Thrift, N., 1996. Reworking EP Thompson’sTime, work-discipline and industrial capitalism’. Time & Society, 5(3), pp.275-299.
Giurge, Laura M., Ashley V. Whillans, and Colin West. “Why time poverty matters for individuals, organisations and nations.” Nature Human Behaviour 4, no. 10 (2020): 993-1003.
Golden, Lonnie and Morris Altman. “How long? The historical, economic and cultural factors behind working hours and overwork.” Research companion to working time and work addiction Ed. Ronald J. Burke, Edward Elgar Publishing (2006): 36-57.
Hochschild, Arlie Russell. 1997. The Time Bind : When Work Becomes Home and Home Becomes Work. 1st ed. New York: Metropolitan Books.
Lennertz, Lora L. and Phillip J. Jones. “A question of time: Sociotemporality in academic libraries.” College & Research Libraries 81, no. 4 (2020): 701.
Mazzetti, Greta, Wilmar B. Schaufeli, and Dina Guglielmi. “Are workaholics born or made? Relations of workaholism with person characteristics and overwork climate.” International Journal of Stress Management 21, no. 3 (2014): 227.
Meyers, Natalie K., Anna Michelle Martinez-Montavon, Mikala Narlock, and Kim Stathers. “A Genealogy of Refusal: Walking Away from Crisis and Scarcity Narratives.” Canadian Journal of Academic Librarianship 7 (2021): 1-18.
Mitchell, Carmen, Lauren Magnuson, and Holly Hampton. “Please Scream Inside Your Heart: How a Global Pandemic Affected Burnout in an Academic Library.” Journal of Radical Librarianship 9 (2023): 159-179.
Nicholson, Karen P. “Being in Time”: New Public Management, Academic Librarians, and the Temporal Labor of Pink-Collar Public Service Work.” Library Trends 68, no. 2 (2019): 130-152.
Rosengren, Calle. “Performing work: The drama of everyday working life.” Time & Society 28, no. 2 (2019): 613-633.
Thompson, E. P. “Time, Work-Discipline, and Industrial Capitalism.” Past & Present 38 (1967): 56-97.
Weber, Max. 2001 [1930]. The Protestant Ethic and the Spirit of Capitalism. New York, NY: Routledge.
Zadeh, Joe. “The Tyranny of Time.” NOĒMA, (3 June 2021) www.noemamag.com/the-tyranny-of-time/.
#ODDStories 2024 @ Quezon City, Philippines 🇵🇭 / Open Knowledge Foundation
In commemorating Open Data Day 2024, we, the UP Resilience Institute YouthMappers (UPRIYM) embraced a vision recognizing biking communities as integral yet vulnerable segments within the urban landscape. Constantly navigating streets shared with motorized vehicles, these communities face the daily struggle for equitable space allocation, advocating for their right to safe and accessible routes. Simultaneously, they embody a passion for bi-modal transportation, championing the versatility of folding bikes as a sustainable alternative. Recognizing their unique position at the intersection of activism and recreation, we sought innovative ways to engage with these communities, harnessing their inherent enthusiasm to drive positive change.
By partnering with biking communities such as the Tiklop Society of the Philippines (TSP), we seized the opportunity to celebrate this year’s Open Data Day by launching Pedal Map, a project to engage biking communities with open mapping for Sustainable Development Goals. This project is a tailored approach that directly addresses their interests and concerns.
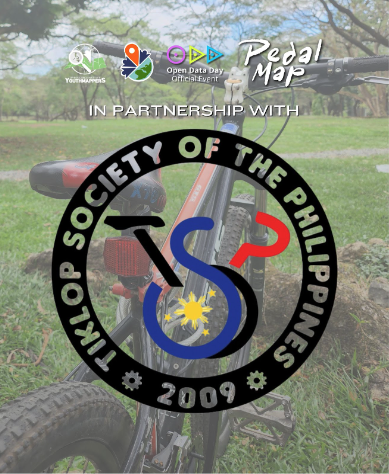
Understanding the transformative potential of open data in shaping urban environments, we collaborated with TSP to facilitate a workshop and conduct a short field session to demystify the open data concept and elucidate its relevance to their daily experiences. Through a session on Mapillary, a platform for street-level imagery collection for OpenStreetMap, bikers were empowered with the knowledge and tools to actively contribute to creating more inclusive and accurate mapping solutions, leveraging their unique perspectives as frequent users of urban thoroughfares.
With a team of 15 dedicated bikers, we traversed the streets of UP Diliman, armed with cameras through their smartphones and a shared vision of enhancing open data accessibility. Through our collective efforts, we contributed over 2,000 images to Mapillary, bridging crucial gaps in our urban landscape’s visual representation through OpenStreetMap.
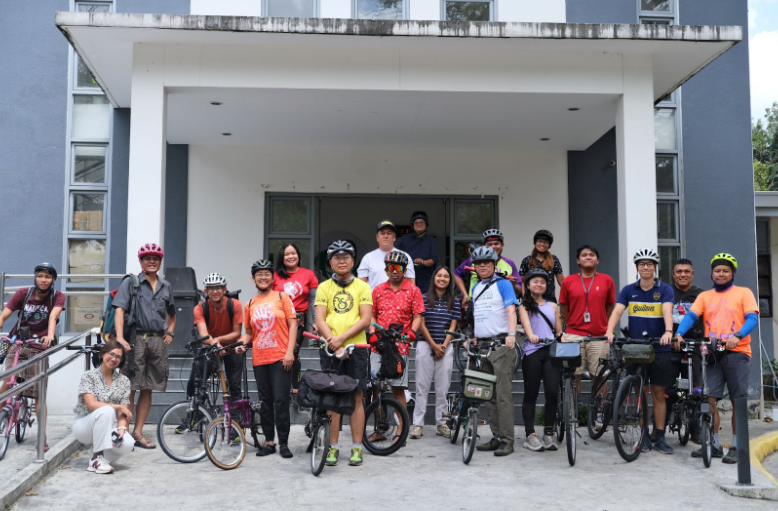
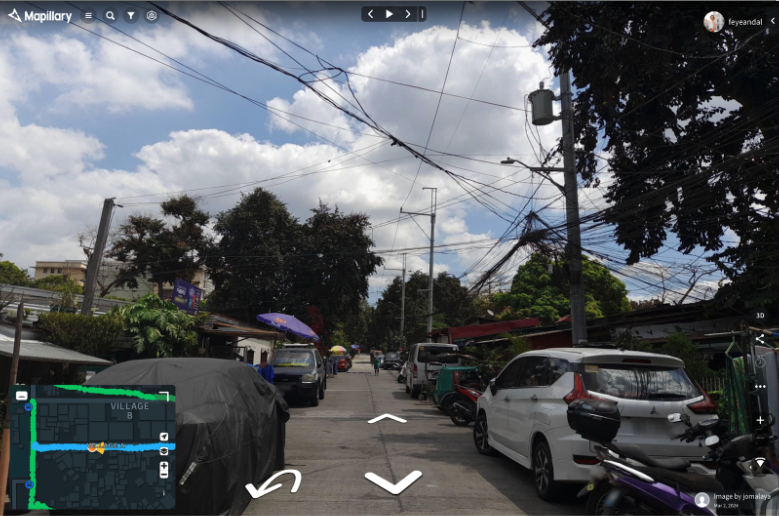
The impact of our collaborative endeavor promises to be profound, fostering a deeper understanding of our surroundings and empowering communities through accessible, open data. Furthermore, our engagement with biking communities extended beyond direct participation in data collection activities to encompass broader advocacy efforts to foster a culture of data literacy and civic engagement. By integrating open data principles into their advocacy initiatives, bikers were equipped to amplify their voices and advocate for infrastructure improvements that prioritize the needs of non-motorized road users. Through this multifaceted approach, we celebrated Open Data Day and cultivated lasting partnerships with targeted communities, catalyzing both direct and indirect impacts on urban mobility and accessibility.
As we reflect on this enriching experience, we eagerly anticipate future partnerships with the Tiklop Society of the Philippines, recognizing the invaluable role of grassroots initiatives in driving positive change.
Our heartfelt gratitude extends to the Open Knowledge Foundation (OKFN) and the Humanitarian OpenStreetMap Team (HOT) for their unwavering support and sponsorship, underscoring the significance of collaborative networks in advancing the global open data movement.
We reaffirm the belief that we are truly #BetterTogetherThanAlone. Through collective action, we can harness the transformative power of open data to build a more sustainable and inclusive future for all.
Transitions for the Caselaw Access Project / Harvard Library Innovation Lab
The Library Innovation Lab is excited to announce that the original limitations on the data available for the Caselaw Access Project expired this month, and that data can now be fully released without restriction on access or use.
As part of our original collaboration agreement with Ravel Law, Inc. (now part of LexisNexis) for the Caselaw Access Project there had been access limitations on the full text and bulk data available, which have now expired. Over the next few months, we will be partnering with other organizations in the open legal data space like the Free Law Project to shepherd this data into its next phase. The Free Law Project already includes all CAP cases, as well as cases scraped from court websites, in its CourtListener search engine.
We will continue hosting the CAP data in bulk for researchers, and as individual readable cases, at case.law. However, we will be winding down services that can be better provided elsewhere, such as the search function and API.
The previous version of the site will still be available at old.case.law until September of this year. If there are features of the previous site that are not well covered by the current site or by CourtListener, we welcome feedback to info@case.law.
This transition will allow new avenues for users to access the data produced by the Caselaw Access Project, and will consolidate efforts to create centralized access points for the law. We are very proud of the contribution that CAP has made to the open legal data movement, and will continue working to expand and support free, open, and fair access to information.
History of the Caselaw Access Project
In 2018, the Library Innovation Lab launched case.law to host and distribute data created by the Caselaw Access Project. Its release was the culmination of several years of work at the Harvard Law Library to digitize a corpus of 6 million cases representing almost all precedential law in the United States. The cases were digitized from Harvard’s own collection of hardbound court reporters from across the nation, an archive which predates the founding of the United States. The digitization process involved removing the binding of each volume, scanning 40 million pages, and using OCR technology to convert the PDF images into human and machine-readable text. You can see parts of that process in this video we released about the project.
Though most government documents are in the public domain, including case law, this scope of United States case law had never before been made easily accessible to the public.
Angela Oduor Lungati: ‘When embracing open source, one must be intentional about being inclusive’ / Open Knowledge Foundation
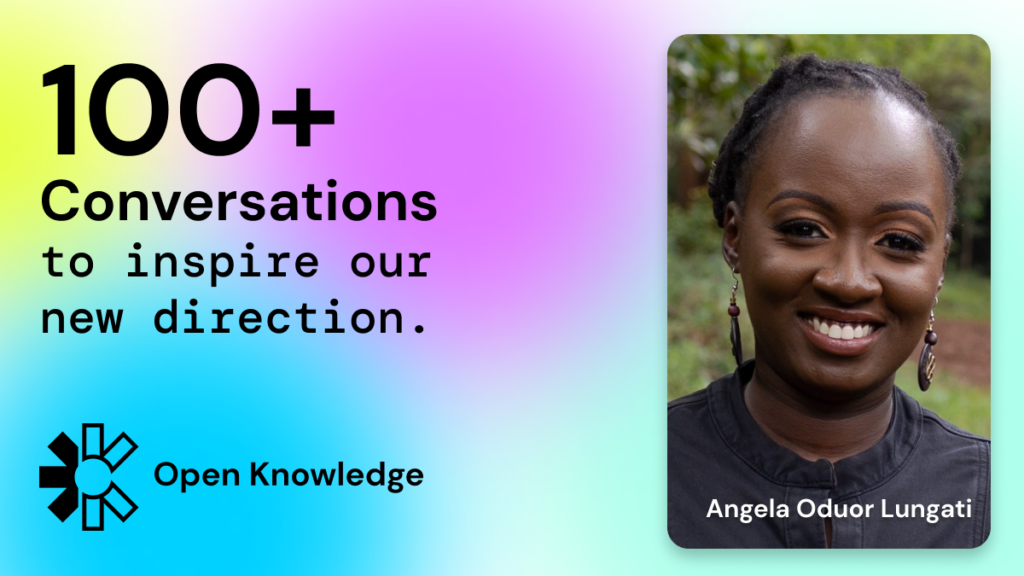
This is the ninth conversation of the 100+ Conversations to Inspire Our New Direction (#OKFN100) project.
Since 2023, we are meeting with more than 100 people to discuss the future of open knowledge, shaped by a diverse set of visions from artists, activists, scholars, archivists, thinkers, policymakers, data scientists, educators, and community leaders from everywhere.
The Open Knowledge Foundation team wants to identify and discuss issues sensitive to our movement and use this effort to constantly shape our actions and business strategies to deliver best what the community expects of us and our network, a pioneering organisation that has been defining the standards of the open movement for two decades.
Another goal is to include the perspectives of people of diverse backgrounds, especially those from marginalised communities, dissident identities, and whose geographic location is outside of the world’s major financial powers.
How openness can accelerate and strengthen the struggles against the complex challenges of our time? This is the key question behind conversations like the one you can read below.
*
This week we had the opportunity to speak with Angela Oduor Lungati, Executive Director of Ushahidi, a global not-for-profit technology company based in Nairobi, Kenya, that helps communities collect and share information.
Angela is a technologist, community builder and open-source software advocate who is passionate about building and using appropriate technology tools to create an impact in the lives of marginalized groups. She has over 10 years’ experience in software development, global community engagement, and nonprofit organizational management. She is also a co-founder of AkiraChix, a non-profit organization that nurtures generations of women who use technology to develop innovations and solutions for Africa.
Like the Open Knowledge Foundation, Ushahidi is a member of the Digital Public Goods Alliance (DPGA), having their software recognised as a Digital Public Good. In December last year, our organisations jointly delivered a workshop on information pollution during elections in Addis Ababa, Ethiopia.
This conversation took place online on 12 March 2024 and was moderated by Renata Ávila, CEO of OKFN, and Lucas Pretti, OKFN’s Communications & Advocacy Director.
We hope you enjoy reading it.
*
Renata Ávila: One of the memories of the early days of the open movement is how strongly it rallied around a positive agenda, rather than just opposing everything. It was this spirit of “I can do it myself”. If I’ve got a computer at home, access to the internet and some skills, I’ll do it. Looking back so many years later, I define them as very privileged compared to so many people who didn’t have those resources. I wanted to do this little introduction to ask you to tell me the story of Ushahidi. How was it born? How long ago?
Angela Oduor Lungati: Ushahidi is a Swahili word that means testimony. The company was born out of a very dark time in Kenya’s history. The background is the 2007 elections. There were some really strong tribal tensions, a lot of mistrust and people were very resistant to the election results if they weren’t the winners. So when the results were announced, violence broke out in all parts of the country. One of the main problems at the time, apart from the violence itself, was that there was a nationwide media blackout. This meant that not everyone in the country was fully aware of the extent of the situation because the media wasn’t able to cover it. There was a huge information vacuum, whether it was for people living in Kenya at the time or people in the diaspora who were worried about their families and the country.
So a group of five Kenyan bloggers got together and decided to find a way to let the world know what was happening. It was a way of bearing witness, sharing testimony. A platform was set up very quickly and people were able to share news of what was happening around them. It could be the riots, or information about people invading a particular place, or the opposite, reports of a largely peaceful area. All very easily via text message, email or Twitter. This information would be pulled into the platform and then visualised on a map. In this first instance of Ushahidi, it was possible to get a very quick visual representation of the pockets of violence in each region and a very quick situational awareness of how deep the problem was.
It’s a context that’s probably common to a lot of other African countries: bad governance and low bandwidth at a time when social platforms were emerging and people could be a bit more vocal about their opinions. So the founders of Ushahidi were able to build a tool that changed the way information flows, because it wasn’t trickling down from official sources, it was more of a bottom-up approach.
They quickly realised that a tool built for this kind of situation could be useful in other places. How can we make it easier for people to replicate this? And that’s the origin story of making the Ushahidi platform open source, making sure that it was easy to download or that people could sign up and get it up and running quickly. Something similar for them to be able to engage with disenfranchised communities and see how they can come together to be part of the solution rather than passive recipients of information.
Renata Ávila: How did Ushahidi develop after that initial spark?
Angela Oduor Lungati: Over the last 16 years, Ushahidi has been used in several categories of social impact. The main one is crisis response. If you think about the Kenyan elections, the Haiti earthquake, the Nepal earthquake, COVID-19, etc., the platform is a very good way to engage with people affected by crises and then have that feedback directly influence humanitarian response and human rights protection.
One of my favourite examples is HarassMap in Egypt. They started by just documenting cases of sexual harassment of women in Egypt. Over time, they built a model that has inspired 20 other different harassmaps around the world. With this initiative, people are not only collecting data and raising awareness, but also thinking about how to use data to influence behavioural and political change.
In Kenya, we’ve used Ushahidi in every single election since 2007. In 2010, during the constitutional referendum, the general elections in 2013, 2017, and 2022. Nigeria communities have also used it extensively. I know it’s also been used in the US and many other spaces. More recently, we’ve been reaching disenfranchised communities to centre the citizen voice in the conversation around climate change.
Renata Ávila: In the beginning, openness was a very technical thing, basically referring to rules in the boundaries of mainstream systems. Open code and open licences, for example, refer to restrictions on the ability to share. So activists were dedicated to opening little windows and cracks in the system to allow better sharing. I’d like to explore the different perspectives of openness today and the different degrees of openness – like the community aspect, participation, governance and accessibility. What role do these elements play in keeping Ushahidi going?
Angela Oduor Lungati: Before I get into the context of Ushahidi, I want to answer this from a conceptual perspective. If you don’t have a community around a tool that you’re trying to make open, that might be a sign that there’s a challenge to openness. A classic example is, if you don’t have people contributing to your code, you start to wonder, is there a challenge with them installing the software? With them understanding how to contribute? With the language that they’re interacting with? I think a great indicator of openness might actually be the size and health of your community. That could tell you how open you are.
If you think about it in the context of Ushahidi, from the moment we open-sourced the platform, the founders were very intentional about being inclusive. We wanted to make sure that anyone who needed the tool could use it, by lowering the barriers. In a time of crisis, there are people who do not have the technical skills or resources to set up a self-hosted instance and keep the technical infrastructure running smoothly.
One of our strategies was to focus the developments on the mobile aspect, that was the lowest hanging fruit to make sure that people could be involved. Mobile phones are the most ubiquitous device, even my grandmother will have one, whether she has access to the internet or not. Another strategy was to recognise that not everyone in the world speaks English. So the logic is, how do we make it easy for people to interact with the tool in a way that they already can? And then, most importantly, how do we facilitate the connection between people to ensure that they can share their learning with each other. If somebody in Kenya is doing an election project and they learn something from it, how do they share that with somebody else in Nigeria, or somebody else in Congo, somebody else in Zambia, so that they can pick it up and build on it and build on it. A lot of the growth we’ve seen has come from making sure that there are structures in place that invite people to participate.
Over time, of course, and this isn’t just Ushahidi, it’s many other non-profits, we’ve had to think about how to sustain this and keep the lights on. That is the sustainability aspect of openness. There’s been quite a bit of friction between the need for openness and maintaining an open community and meeting the needs of the places where the revenue comes from. That’s what we’ve been dealing with for a significant part of our journey.
In the last five or six years that I’ve been executive director, it’s been about how do we get back to our roots and nurture that open source community while making sure that there’s a business model that can still support all of that to keep the lights on.
Renata Ávila: We share the same challenges with CKAN, a software managed and held in trust by OKFN. How should we spin while nurturing the community? How should we invest in governance? How should we invest in translation and localisation? Compared to 20 years ago, societies all over the world have become more precarious, which means, for example, that a student or volunteer who used to be able to devote time to a project now has to work three jobs to pay the bills. On the other hand, there’s basically no public funding that’s really willing to contribute. So these new austerity measures have undoubtedly had an impact on our communities. Today, bodies like the Digital Public Goods Alliance (DPGA), on which we both sit, are opening a very interesting door for collaboration on projects like ours.
So what are your thoughts on how to keep communities alive? How can we keep people coming and nurture the open communities to create value for the public good in such an unfavourable context?
Angela Oduor Lungati: The short answer is that we are still trying to find out. I can give examples of programmes that have been broadly useful in acknowledging the economic challenges while still being able to demonstrate value.
I took over Ushahidi at the beginning of the COVID-19 crisis. Surprisingly, the pandemic was a silver lining because the use of our platform skyrocketed, proving its usefulness. There was a group in Spain, Frena La Curva, who set up the platform to create a kind of mutual aid functionality. They documented that model and shared it with 22 other Latin American and Spanish-speaking countries, and it ended up being used. It’s been very helpful because we’ve been able to communicate the value of the platform in this day and age, while showing the challenges in terms of resources. Our messaging was around ‘we are a small team’, ‘here are ways you can get involved and help’, ‘you can start an instance’, ‘you can share some of your feedback’, etc. Then we combined that with participating in programmes like Google Summer of Code and Outreachy.
I mention that because we got over the barrier of entry on the first point. We’ve managed to get the volunteers paid, thankfully, through the support of the Outreachy structure programme, and I’ve actually been able to hire two people who have come out of the community. That’s all well and good. I don’t know what will help us to grow, but it’s a first step.
I think that’s why we have programmes like the Digital Public Goods Alliance. I really appreciate the wave of awareness among funders to see the value of these tools in achieving the Sustainable Development Goals, and to understand that there’s some maintenance that’s required, not just on the technical side, but in the communities that are the backbone.
I think the other part might actually be in reinventing or rethinking our engagement strategies. The way we engage, the way we motivate volunteers now is very different from the way we used to do it.
Lucas Pretti: Let me build on this question of motivation. The spirit of the open movement that Renata talked about at the beginning came with a hacker component. We used to create our own tools, but we also hacked all the other tools available, whether mainstream or not. Recently, with the growing hegemony of Big Tech and the concentration of power around them, that hacker motivation has diminished considerably. At the same time, I feel that this hegemony has passed its peak, and there is considerable awareness that the software produced by Big Tech is harmful.
At OKFN we’re constantly asking ourselves what kind of software we need to develop. What advice would you give us? How can we motivate developers to be on this side?
Angela Oduor Lungati: When it comes to the contrast with Big Tech, I think we will always be playing catch-up. The Big Tech companies have all the resources, all the manpower and, to be honest, they’re taking all the talent because of the same economics that Renata was talking about. People need to be able to put food on the table and take care of their families.
Right now it feels like we are in competition or we have to build tools that can be on par with big tech, but we don’t have the capacity, the talent or the funding to be able to work at that level. I wonder if there’s complacency or just acceptance of a fact. We may have people who are driven by passion to change things, but who have to rely on the fact that they still have to put food on the table.
But sometimes there’s an alternative view. In the Kenyan context, I see developers saying, “I’ll come in for a while, get the resources I need for a while, and then come back.”
Lucas Pretti: That would be a kind of hacking.
Angela Oduor Lungati: It could be. But I’m still waiting for that comeback. Because what I’m seeing now is a huge avalanche that’s just sucking the talent out of the non-profit sector and it’s all going into Big Tech. I know there are people in there trying to fight the good fight. But I wonder if it’s going to be a lasting game.
Renata Ávila: Absolutely. One of our hopes is that the open movement will sit down and discuss these difficult questions together, because there is part of the answer in every community. We need those answers to understand what unites us to move forward.
We’ve also had a lot of discussion at OKFN about openness as a design principle. In conclusion, I can confidently say that Ushahidi is a perfect example of an open-by-design effort from day one. You’ve been true to openness in the design of the practices, the platforms, the governance system, and so on. It is such a privilege to see a real, tangible example of what openness as a design principle can achieve in institutions, communities and as a global effort. I learned a lot from this conversation.
Lucas Pretti: Absolutely. On that note, Angela, perhaps you could say a few final words. Maybe starting with what’s next for Ushahidi?
Angela Oduor Lungati: We feel like we’ve got to a point where there’s a lot of data, I mean the butter is on the bread, but what’s the data saying? What stories are we telling? How do we make this emergence or this resume of voices more meaningful? We realised that there’s a gap between the point where you get data and the point where it actually influences change. When we try to think critically about what role we can play in that, we have to think about whether the data we have is actually representative.
When we say we are working with disenfranchised communities, can we actually attribute what disenfranchisement means? Can we break that down by gender? Can we break that down along geographical lines, social lines, economic lines? And for somebody who’s a decision-maker, it could be somebody in government or a donor, how do we make it easier for them to gain insight and make sense of the situation? Are they able to draw comparisons or similarities between some of these different areas?
We have some hypotheses about how we might do some of these things. One of the biggest is to think about creating this federated knowledge hub of Ushahidi instances, putting the data from all those instances in one place that anyone can query, but in a way that protects cultural appropriation, that takes ownership into account and makes sure that it’s not extractive, that it’s engaging and that that consent is built into it and that local laws are respected. That’s going to be very interesting to watch.
Another very big question mark is what role can we play in continuing to foster a thriving open ecosystem, when new technologies are moving at such a fast pace. That’s basically the conversation we just had. How do we also contribute to building a responsible ecosystem while all this is happening? We know that a lot of the knowledge that’s out there right now is because of all the work that’s been done by the open movement, but that’s all going into proprietary models, which can have a direct impact on motivations.
It’s still a complex challenge, but one that we’re really thinking critically about.Renata Ávila: I think we will continue the conversation because our community is very interested in that. We are very keen to help in any way we can.